Introduction
Eye movements have been increasingly recognized as a valuable source of information in understanding visual cognition and human-computer interaction (HCI). Recent research has highlighted the importance of eye tracking in various domains, providing insights into cognitive processes and user behavior.
Holmqvist et al. (2021) reviewed eye-tracking measures and their applications in visual cognition. They emphasized the importance of microsaccades, small involuntary eye movements that occur several times per second, in maintaining visual perception and attention. Their study highlighted how these micro-movements contribute to the stability of visual perception and can be used as indicators of cognitive load and attention allocation (Holmqvist et al., 2021).
Building on this, Eckstein et al. (2017) explored the relationship between eye movements and decision-making processes. Their research demonstrated that eye movements reflect cognitive processes and play an active role in shaping decisions. They found that the pattern of eye movements before a decision could predict the outcome with above-chance accuracy, suggesting a bidirectional relationship between visual attention and decision-making (Eckstein et al., 2017).
In the context of human-computer interaction, Feit et al. (2020) investigated eye tracking for understanding user behavior in graphical user interfaces. Their study revealed that eye movement patterns could provide valuable insights into users’ search strategies, information processing, and usability issues. They proposed novel metrics for analyzing eye-tracking data in HCI contexts, which could lead to more intuitive and user-friendly interface designs (Feit et al., 2020).
Furthermore, Kiefer et al. (2017) explored the potential of eye tracking in virtual and augmented reality environments. They found that eye movements could be used to enhance interaction in these immersive environments, potentially leading to more natural and efficient user experiences. Their work highlighted the importance of considering eye movements in the design of next-generation user interfaces (Kiefer et al., 2017).
Recent advancements in eye-tracking technology have also enabled more nuanced studies of reading behavior. Schotter and Payne (2019) used eye-tracking to investigate the cognitive processes involved in reading comprehension. Their study revealed how readers allocate attention during text processing and how this allocation changes based on text difficulty and reading goals. These findings have implications for both educational practices and the design of digital reading interfaces (Schotter & Payne, 2019).
Recent literature underscores the significant impact of eye movements in understanding visual cognition and improving human-computer interaction. From microsaccades to complex gaze patterns, eye movements provide a window into cognitive processes and user behavior. As eye-tracking technology advances, it promises to offer even deeper insights into how we perceive, process, and interact with visual information in both digital and physical environments.
Studying eye movements during psychophysical activities has become an increasingly important area of research, providing valuable insights into information-processing strategies and task performance that are often inaccessible to conscious introspection. Recent advances in eye-tracking technology have allowed researchers to explore various aspects of visual perception, cognition, and behavior in greater detail.
Mathôt et al. (2021) investigated the relationship between pupil size and visual perception, demonstrating that changes in pupil size can affect visual sensitivity and reaction times. Their findings suggest that pupil size should be considered a potential confounding factor in psychophysical experiments involving visual stimuli. This study highlights the intricate connection between physiological responses and cognitive processes, emphasizing the need for careful experimental design in visual perception studies.
In the context of reading, Schotter and Payne (2019) used eye-tracking to examine how readers process text and decide when to move their eyes. Their research revealed that readers use low-level visual information and higher-level linguistic knowledge to guide eye movements during reading. This work underscores the complexity of the reading process and the importance of considering multiple factors when studying eye movements in linguistic tasks.
Poletti and Rucci (2021) provided a comprehensive review of recent advances in understanding the role of fixational eye movements in vision. They highlighted how these subtle movements, often overlooked in traditional eye-tracking studies, play a crucial role in visual perception and information processing. This research emphasizes the importance of considering even minute eye movements when interpreting visual processing and perception data.
Expanding the application of eye-tracking beyond basic research, Shiferaw et al. (2019) explored its use in clinical settings. They reviewed the use of eye movement analysis in diagnosing and monitoring neurological disorders, emphasizing the potential of eye-tracking as a non-invasive tool for assessing cognitive and motor function in various clinical populations. This work demonstrates the broader implications of eye movement research, extending its relevance to medical diagnostics and patient care.
These recent studies collectively highlight the ongoing importance of eye movement research in understanding human perception, cognition, and behavior. As eye-tracking technology advances, it is likely to play an increasingly important role in basic research and applied settings. The ability to measure eye movements during psychophysical activities provides a window into cognitive processes that are typically inaccessible through other means, offering insights into information processing strategies and task performance. This growing body of research enhances our understanding of visual perception and cognition and has practical applications in fields ranging from human-computer interaction to clinical neurology.
Eye tracking can be used to analyze and understand how people read a text. It is done through a camera that records where the subject’s eye looks, as these points are placed in the text to follow the subject’s reading behavior. The eye moves while reading and reads a line of text by making a series of fixations and movements. When we look at something, read, or search for an object, we make “saccadic” eye movements.
Regarding web tasks, searching is one of the most common actions in our daily lives, and the shorter the time needed to find the target, the more influential the search. When referencing eye movement patterns for simpler stimuli, the target stimulus is found with a few fixations, but the average durations of these fixations are high. During web searches, the number of times users look at the target after the first fixation provides information about the development of the search process (Hautala et al., 2018).
As the degree of stimulus complexity increases, the number of fixations needed to find the target stimulus increases, and the average duration of fixations decreases (Wu et al., 2019). According to Wu et al. (2019), the analysis of eye movements can also suggest differences in ocular processing between easy and difficult tasks. However, it should be noted that knowing the task objective accelerates target detection in web search tasks. This indicates that our visual system influences our attentional system, as the early presentation of the target makes the goal easier to achieve).
Gayet et al.’s (2024) study explore how the attentional template, a mental representation used in visual search, incorporates viewing distance. Four experiments asked participants to search for familiar objects (like cars or people) at varying distances in outdoor scenes. The results showed that participants were more accurate in identifying objects when the size of the objects matched the expected size based on viewing distance. This effect was stronger when the objects also matched the expected shape. The study concludes that attentional templates are influenced by both category-specific attributes (shape) and context-dependent expectations (size).
Various studies have been conducted based on eye-tracking data characteristics, such as fixation duration and pupil size. In one such study, subjects were presented with a list of results from which they selected links for consultation. It was concluded that subjects spend considerable time fixating on the first and second results before selecting a link (Kammerer & Gerjets, 2014).
Research indicates that if a website is not visually appealing, it has a powerful impact on the subject’s perception of the web page (Seckler et al., 2015). In this sense, the subject is guided by an information need; that is, an individual’s intention to perform a web search is often not informative but navigational (obtaining the URL of a desired site) or maybe transactional (the individual aims to perform a specific transaction, such as purchasing or downloading a document).
In another study, users had clear expectations of where objects on the web were located. However, the availability of web objects led to fewer fixations, and participants found objects more quickly (Bernstein et al., 2020). In a study on search tasks, participants freely navigated various web pages while performing specific tasks, such as removing links. Navigation between columns showed that participants are slightly more likely to shift their view between columns than to remain fixed within a column (Arapakis et al., 2020).
Regarding advertisements on web pages, studies show that eye movements are strongly related to the order in which ads are presented. The type of task, ads, and sequence influence the search (Ahn et al., 2018). These can represent information processing. Referencing studies that considered user characteristics, the fixation pattern on almost all web pages showed that adults looked at parts of the page more than young adults (Wu et al., 2019).
The present work aims to understand how the target’s position in navigational and informative tasks influences eye movement in university students. It thus aims to compare the relationship between the target’s position in navigational and informative tasks with the speed of eye movement and the relationship between the individual’s position and eye movement.
Methods
This study employs a quasi-experimental, cross-sectional quantitative design.
Participants
The sample consisted of 20 participants (13 females, seven males) aged 18 to 44. Regarding web usage experience, 75% reported daily internet use. Time spent online daily was distributed as follows: 35% spent 2 to 3 hours, 30% spent 3 to 4 hours, and 35% spent over 5 hours. 75% of participants did not wear glasses, of which 33.3% were male. Half of the participants had experience with virtual reality glasses.
Materials
An eye-tracking device recorded participants’ gaze patterns during the search tasks. This equipment allows for tracking eye movements and collecting visual information processing data. Stimuli consisted of two websites: YouTube for the navigational task and Momondo for the informational task.
Procedure
Before the experiment, participants provided informed consent and completed a sociodemographic questionnaire. The eye-tracker was calibrated individually for each participant, followed by a familiarization session.
Based on the study design, the task type (navigational vs. informational) was crossed with the target position. For the navigational task, participants were instructed to open YouTube and search for a song of their choice. The informational task required participants to open Momondo and follow specific instructions. Each participant completed both task types in a fixed order. Tasks began with participants reading the task description and clicking a provided hyperlink to initiate a predefined search. A task was deemed complete when the participant verbally confirmed following instructions.
Data Analysis
Given the study’s objectives to compare groups with three independent variables (Task Type, Target Position, and Individual Position) and three dependent variables (Average Fixation, Number of Blinks, and Average Blink Duration), a 2x2 MANOVA was employed. The confirmation of normal distribution in the data supported this choice. The significance level was set at 5% (p < .05) for all analyses.
Results
Prior to conducting the multivariate analysis, the normal distribution of data was confirmed using Skewness and Kurtosis measures (±2). Linear combinations of dependent variables were then computed to maximize the differences across various conditions of the independent variables. The multivariate analysis revealed strong effects for two key interactions. First, the interaction between Sex and Target position in the informative task yielded F(6, 2) = .483, p = .793, η p 2 = .592, with an observed power of .073 and Wilks’ λ = .408. Second, the interaction between Sex and Individual position produced F(6, 2) = .398, p = .839, η p 2 = .544, with an observed power of .069 and Wilks’ λ = .456. These results suggest substantial interaction effects, as evidenced by the large effect sizes (η p 2). However, it is important to note that the p-values were not statistically significant despite these strong effects. This apparent contradiction may be attributed to the small sample size, reflected in the low observed power for both interactions. These findings underscore the complex interplay between participant sex, target position, and individual position in influencing the dependent variables under study.
According to the results presented in Table 1, a strong effect was observed for the interaction between average fixation duration and target position in the navigational task (η p 2 = .167). Conversely, weak effects were found for the interaction between average fixation duration and target position in the informative task (η p 2 = .039).
Table 1: Relationship Between Target Position and Eye Movement Patterns in Navigational and Informative Tasks.

The dependent variables are presented with 95% confidence intervals. The dependent variable, average fixation duration in the navigational task, ranges from 255.410 to 326.931 [units not specified, presumably milliseconds]. The average fixation duration in the informative task ranges from 274.031 to 374.527 [units].
As observed from the data in Table 2, it can be concluded that there is a moderate effect for two variable combinations: Target position in the navigational task and average blink duration in the navigational task (η p 2 = .093); Target position in the informative task × average blink duration in the informative task (η p 2 = .085).
Table 2: Relationship between target position and eye movement speed in navigational and informative tasks.
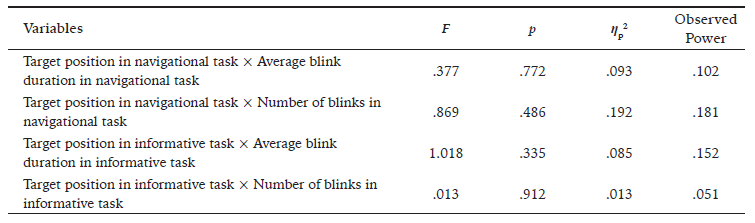
The interaction between number of blinks and target position in the navigational task shows a strong effect (η p² = .192). In contrast, the combination of target position in the informative task × average fixation duration and the combination of target position in the informative task × number of blinks show weak effects: η p 2 = 0.085 and η p² = .013, respectively (Table 2).
The dependent variables in our study were analyzed using 95% confidence intervals. For the navigational task, we observed an average blink duration ranging from 204.236 to 1656.397 milliseconds, while the informative task yielded average blink durations between 553.598 and 1864.440 milliseconds. The number of blinks also varied between tasks, with the navigational task showing 1.987 to 9.786 blinks, and the informative task recording 9.648 to 20.040 blinks.
Table 3 results reveal strong effects in the interactions between variables. Specifically, we found robust interactions between average fixation duration and individual position in both task types. For the navigational task, this interaction showed an effect size of η p² = .216, while the informative task demonstrated an effect size of η p² = .176. These substantial effect sizes suggest that an individual’s position significantly influences their fixation patterns across both task types.
Table 3: Interaction between individual position and eye movement patterns in navigational and informative tasks.

Further analysis of the dependent variables using 95% confidence intervals again showed that the average fixation duration in the navigational task ranged from 263.566 to 322.430 milliseconds. In comparison, the average fixation duration for the informative task spanned from 270.076 to 342.473 milliseconds.
These findings highlight interesting differences in eye movement patterns between navigational and informative tasks. The longer blink durations and higher blink frequencies observed in the informative task might indicate increased cognitive load or visual processing demands. Similarly, the slightly longer fixation durations in the informative task could suggest more complex information processing requirements.
The strong interaction effects between fixation duration and individual position across both tasks underscore the importance of considering spatial factors in eye movement studies. However, it’s worth noting that while these effect sizes are large, their statistical significance should be carefully evaluated in the context of the study’s sample size and power.
Overall, these results provide valuable insights into how task type and individual positioning influence eye movement patterns, paving the way for further research.
Discussion
In discussing the results presented, it is essential to consider the theoretical frameworks that underpin eye movement behavior in different task contexts. The observed differences in effect sizes between navigational and informative tasks can be explained through the lens of several established theories in cognitive psychology and human-computer interaction.
The strong effect (η p² = 0.167) observed for the interaction between average fixation duration and target position in the navigational task aligns with the Visual Search Theory proposed by Wolfe in 1994 and the Active Vision paradigm advanced by Findlay and Gilchrist in 2003. These theories posit that individuals employ more strategic and efficient visual search patterns during goal-directed tasks like navigation. The strong interaction effect suggests that the target’s position significantly influenced participants’ fixation durations, indicating a more focused and purposeful visual exploration strategy in navigational contexts.
Conversely, the information Foraging Theory can interpret the weak effect (η p² = .039) for the same interaction in the informative task (Pirolli & Card, 1999). This theory suggests that individuals adopt a more exploratory and less targeted approach in information-seeking tasks, akin to foraging behavior. The weak interaction effect implies that fixation durations were less dependent on target position, possibly due to a more distributed attention allocation across the visual field when processing informative content.
The moderate effects observed for the interactions between target position and average blink duration in both task types (η p² =.093 for navigational and η p² = .085 for informative tasks) can be understood through the Cognitive Load Theory presented by Sweller in 1988. Blink duration has been associated with cognitive processing demands (Siegle et al., 2008). The moderate effect sizes suggest that the target’s position influences cognitive load similarly in both task types, possibly reflecting the general attentional demands of locating and processing visual targets regardless of the specific task context.
The strong effect (η p² = .192) observed for the interaction between the number of blinks and target position in the navigational task is particularly intriguing. This finding can be interpreted through the Embodied Cognition framework developed by Wilson in 2002 in na article with the title Six views of embodied cognition, which emphasizes the interplay between cognitive processes and physical actions. The strong relationship between blink frequency and target position in navigational tasks might indicate a more pronounced coupling between visual-motor behavior and spatial cognition.
The weak effects observed for the interactions involving target position in the informative task (η p² = 0.085 for average fixation duration and η p² = .013 for number of blinks) align with Bruner’s Constructivist Theory of Learning developed by Bruner in is book Toward a Theory of Instruction published in 1966. This theory posits that individuals construct knowledge by exploring and integrating new information with existing knowledge structures. The weak effects suggest that in informative tasks, eye movement patterns are less constrained by target position and more influenced by individual differences in information processing strategies and prior knowledge.
These findings contribute to understanding how task demands modulate visual attention and eye movement behavior. The stronger effects observed in navigational tasks suggest a more direct coupling between visual behavior and task goals in spatial contexts. In contrast, the weaker effects in informative tasks imply a more flexible and individualized visual exploration strategy when processing complex information.
The observed patterns in eye movement behavior across navigational and informative tasks can be interpreted through several theoretical frameworks in cognitive psychology, human-computer interaction, and visual attention research. This discussion will focus on explaining the underlying mechanisms that may account for the differences in blink duration, blink frequency, and fixation patterns between the two task types.
Blink Duration and Frequency: The marked difference in blink duration and frequency between navigational and informative tasks aligns with the Cognitive Load Theory and the Adaptive Gain Theory of Locus Coeruleus-Norepinephrine (LC-NE) function (Aston-Jones & Cohen, 2005).
The longer blink durations observed in the informative task (553.598 to 1864.440 ms) compared to the navigational task (204.236 to 1656.397 ms) suggest a higher cognitive load associated with processing complex information. This aligns with research by Siegle et al. (2008), which demonstrated that blink duration increases with cognitive processing demands. The informative task likely requires more in-depth information processing and integration, leading to longer blinks as participants engage in deeper cognitive processing.
The Adaptive Gain Theory can explain the increased blink frequency in the informative task (9.648 to 20.040 blinks) compared to the navigational task (1.987 to 9.786 blinks). This theory posits that blink rate is modulated by the LC-NE system, which regulates arousal and attention. The higher blink rate in the informative task may indicate a more exploratory attentional state, where participants actively seek and integrate new information. Conversely, the lower blink rate in the navigational task suggests a more focused, exploit-oriented attentional state consistent with goal-directed navigation.
Fixation Duration and Individual Position: The strong interaction effects between average fixation duration and individual position in both task types (η p² = .216 for navigational, η p² = .176 for informative) can be interpreted through the lens of the Active Vision paradigm (Findlay & Gilchrist, 2003) and the Theory of Embodied Cognition proposed by Wilson in 2002 ).
The Active Vision paradigm posits that eye movements are not passive responses to visual stimuli but are actively controlled to support ongoing cognitive processes. The strong interaction effects suggest that participants’ fixation patterns are significantly influenced by their physical position, indicating a tight coupling between bodily orientation and visual attention allocation. This interpretation is further supported by the Embodied Cognition framework, which emphasizes the interplay between cognitive processes and physical states. The robust interaction between fixation duration and individual position across both task types suggests that spatial cognition and visual attention are deeply intertwined with the observer’s physical orientation in space.
The slightly stronger effect in the navigational task (η p² = .216) compared to the informative task (η p² = .176) may reflect the heightened importance of spatial awareness in navigation. This aligns with the Spatial Cognition Theory developed by Montello in 1993, which emphasizes the role of spatial representations in navigational tasks.
Fixation Duration Differences
The observed differences in fixation durations between navigational (263.566 to 322.430 ms) and informative (270.076 to 342.473 ms) tasks, although slight, can be explained by the Information Processing Theory presented by in Miller in 1956, and the Limited Capacity Model of Attention in 1973.
The marginally longer fixation durations in the informative task suggest that participants required more time to process and integrate complex information. This aligns with the Information Processing Theory, which posits that cognitive processing occurs in stages, with more complex information requiring longer processing times.
Future studies should aim further to elucidate these task-dependent differences in eye movement patterns, potentially incorporating neuroimaging techniques to explore the underlying neural mechanisms. Additionally, investigating how these patterns may vary across different populations (e.g., experts vs. novices) could provide valuable insights for user interface design, educational technology, and cognitive ergonomics.
The Limited Capacity Model of Attention further supports this interpretation, suggesting that the informative task may demand more attentional resources, leading to slightly prolonged fixations as participants allocate their limited cognitive resources to process the information.
Conclusion
The primary objective of this study was to understand how the target’s position influences eye movement in navigational and informative tasks. By analyzing a sample of 20 university students using the MANOVA (2x2) test to maximize the differences between various conditions of the independent variables, significant results were obtained that effectively addressed the study’s objectives.
The findings indicate that the target’s position in the navigational task significantly influences the eye movement of university students. Specifically, participants exhibited distinct eye movement patterns depending on the target’s location on the screen, suggesting that target position can affect the efficiency and strategy of visual search.
Moreover, it was found that the individual’s position also influences eye movement in both navigational and informative tasks. This finding underscores the importance of considering the target’s position and the observer’s position when analyzing eye movement in different task contexts.
These conclusions highlight the relevance of incorporating variables such as target position and observer position in studies of visual perception and human-computer interaction. Understanding these influences can contribute to developing more intuitive and efficient interfaces, enhancing the user experience in various applications, from web navigation to augmented and virtual reality environments.
In summary, this study provides robust evidence that both the target’s and individual’s positions are crucial determinants of eye movement during navigational and informative tasks. Future research may expand on these findings by exploring different populations and task contexts and integrating other measures of cognitive and behavioral performance.