I. Introduction
The residential location plays a crucial role in shaping urban spatial structure and city dynamics. It impacts employment, economic development, social structure, spatial segregation, and the transport system (Schirmer et al., 2014). In Latin American cities, the inequality in wealth distribution results in a highly segmented formal real estate market, driven by the purchasing power of the demand. Abramo (2007) highlights a distinctive interaction between formal and informal real estate markets in these large cities, leading to specific patterns of urban structure. Understanding the specificities of residential location is important for urban planners, policymakers, and researchers.
Literature on the factors influencing the residential real estate market explores the impacts of residential location on the unequal pricing of urban land. Generally, proximity to employment and consumption centres, as well as location along preferential routes and public transportation, positively influences residential choices and prices. However, divergent results show that some residents prefer quietness and proximity to natural assets over busy and noisy areas, complicating the relationship between location attributes and urban land prices. This article reviews various perspectives on this matter, noting that higher-income groups may have different preferences, potentially leading to self-segregation, while the most vulnerable populations face imposed segregation (Corrêa, 2013).
This paper presents a quantitative approach focusing on two location attributes, accessibility and centrality, based on weighted network models. The objective is to analyze whether and how these spatial attributes impact the prices of used apartments in Porto Alegre, Brazil. We correlate the apartment prices with these configurational measures using the Kernel Density Estimation (KDE) method, while acknowledging that other locational factors may also influence property appreciation.
The article is structured into six sections, in addition to this Introduction. Next, we present the theoretical framework regarding the relationship between urban location and residential land prices (section 2), as well as the influence of spatial centrality on prices (section 3). The fourth section details the methodological pathway followed in this research, and the fifth section presents and discusses the results. In the final section, we summarize the main findings, relating them to the specificities of residential locations in a Global South city.
II. Location and residential land prices
According to the premises of classical urban economics, the land value is inversely proportional to the distance from the Central Business District (CBD) (Alonso, 1964) or to the multiple centres present within the urban system (Narvaez et al., 2013). Alonso considers three classes of land uses competing for the best location to satisfy their needs: businesses, residences, and agricultural uses. It is assumed that business owners make location decisions with the sole goal of profit, defined as the subtraction of operational costs (including land costs) from business volume. For retail and services in general, this volume decreases as one moves away from the CBD at a rate higher than the decrease in land costs, meaning their profits are higher in more accessible or centrally located areas (Richardson, 1975). The pursuit of economic activities toward the centre(s) makes this portion of land extremely competitive and, therefore, more expensive, hindering uses incapable of sustaining their costs due to lower productivity or economic surplus. As the distance from these central areas increases, less competitive and cheaper land allows for less profitable uses, such as residential. These locations are characterized by lower built densities and a lower diversity of uses while at the same time lacking the positive aspects associated with urban vitality (Jacobs, 2011; Montgomery, 1998; Netto, 2017) as well as their negative externalities, such as air and noise pollution, congestion, and presumed higher insecurity (Neuman, 2005).
Classical economic theories, while effective in modelling the general structure of urban land prices and their macro-spatial repercussions, do not account for crucial local peculiarities that determine real estate appreciation or depreciation. Specifically, concerning residential use, Schirmer et al. (2014) emphasize that land prices can capture various locational characteristics, with accessibility being one of them. Others include the shape and arrangement of built objects, the socio-economic environment, and points of interest that provide relevant services to the population. Seabra et al. (2016), for instance, found that factors such as proximity to parks and bodies of water significantly increase the value of residential properties, while proximity to areas of social interest and no-go areas decreases their value. Therefore, the highest prices do not always coincide with areas of superior centrality due to the existence of disamenities in those locations, such as congestion, noise, air pollution, higher crime rates etc., in addition to the possible occurrence of demand attractors in other areas. Moreover, since the crisis of urban Fordism and due to the growing importance of the real estate market in coordinating land uses, these local attributes have become crucial in defining land prices and determining who can afford to live there (Abramo, 2007). For this reason, any effort to correlate an independent variable related to urban spatial configuration with the dependent variable price must assume imperfect correspondences, constrained by "noise" related to other locational factors, as well as intrinsic aspects of the property itself.
III. Centrality and residential land prices
As mentioned, this article adopts two network measures: accessibility and centrality. The first is associated with the idea of proximity or ease of reaching a location in relation to the set of locations within an urban system (Hansen, 1959), while the second assesses the role of nodes as intermediaries in information exchanges (Freeman, 1978). Both capture the essence of locational advantage in a city, with their value reflected in land use intensity (Porta et al., 2009). Land portions with high accessibility are contested by activities with high productivity and tend to be more expensive, concentrating uses that satisfy demands for public or private goods and services. In addition to the cost increase resulting from competition among businesses for scarce central land, proximity to certain points of interest is also considered in residential location decisions, which can contribute to land appreciation. For example, Axhausen et al. (2004) and Pinjari et al. (2009) found a strong preference for proximity to educational facilities. Similarly, the density of retail establishments, services, and shopping centres has a positive influence on residential choices (Guo & Bhat, 2007; Schirmer et al., 2014; Zondang & Pieters, 2005). However, Heyman and Manum (2016) caution that a certain distance between such establishments and residences is also relevant because while proximity is appreciated, its negative effects (traffic, noise) are undesirable.
For this reason, if central locations are preferred by retail and business uses, for residences this attribute has contradictory effects. Accessibility to employment hubs is estimated to be significant, as per the findings of Guo and Bhat (2007) and Zolfaghari et al. (2012) for San Francisco and London, respectively. However, general accessibility was considered marginal by Zondang and Pieters (2005) in a study of the Dutch urban network. Proximity to highly central roads, on the other hand, can be devalued when the impact of negative externalities (air and noise pollution) outweighs the benefits of positive ones (proximity to goods, services, and jobs), as indicated by Bürgle (2006). Possibly for this reason, Bürgle (2006), Palma et al. (2005), and Kim et al. (2005) conclude that being further away fro the main centres of the studied urban areas (Paris, the Oxford region, and Zurich, respectively) is highly valued. Conversely, Axhausen et al. (2004), while confirming this phenomenon in Zurich, find that proximity to local centres is preferred. Hence, it is important the simultaneous measure of centrality at both the global and local scales. Furthermore, in certain contexts (such as in the Danish and Swedish capitals), accessibility, both locally and globally, was positively related to apartment markets, a phenomenon not observed in the single-family home market, where residents supposedly reject more central and busy areas (Heyman & Manum, 2016).
Chiaradia et al. (2009), in a detailed study of an extensive suburban area of London, concluded that local centres are characterized by higher land use intensities. Although the absolute prices of many units are low, unit values are significantly higher near areas with greater centrality. According to the authors, there is a clear substitution effect between density, plot size, and proximity to central amenities: if a portion of solvent demand seeks to escape the negative externalities resulting from high density and consumes larger spaces on the periphery, others prefer the positive externalities of density and proximity to central amenities. The first case would constitute what Corrêa (2013) calls self-segregation, a prerogative inherent to those who can choose the location of their homes, i.e., middle and upper classes, since for them, land price is secondary or marginal. Economically less privileged individuals and families, on the other hand, are compelled to settle in areas rejected by the former, a fact described by Corrêa (2013) as imposed segregation.
In the Brazilian municipality of Bento Gonçalves (RS), Spinelli (1997) found significant correlations between land prices and centrality weighted by building density, economic activities, and local amenities and points of interest. In the context of Porto Alegre (RS), Costa (2016) reveals that upmarket multifamily residential buildings are more centrally located compared to the overall universe of residences and other classes of apartments. Additionally, this high-income residential typology shows a more significant concentration than others along roads with high centrality weighted by retail establishments.
VI. Materials and methods
1. Network measures
Hedonic models1 are commonly estimated in which the property's rent or purchase/sale value is included as the dependent variable, while intrinsic property characteristics, neighborhood amenities, and locational endowments are included as explanatory variables. The distance to the CBD is considered a proxy for differential location advantages, although criticism arises regarding the definition of the centre or the identification of the CBD being exogenous to the model, and the fact that this geometric distance disregards differences in accessibility and other micro-locational aspects. Therefore, accessibility to goods and services, rather than Euclidean distance, is a complex notion that greatly influences land value. A configurational approach, which considers the relative location of urban space units (street segments), and simultaneously, addresses these inconveniences, accurately incorporating the locational factor into the model and endogenizing the notion of centrality - or relative accessibility to the centre(s) (Chiaradia et al., 2009).
This work uses two of its measures as a methodological strategy to represent the locational advantages of spaces, both weighted by the population's intensity of occupation (given by the population of the respective census tract). The first measure, weighted accessibility, determines the degree of proximity of a cell (street segment) in relation to the set of cells that define the urban system under analysis. In this research, it relates to the degree of proximity of a particular apartment to the set of urban locations or, in other words, to the ease to access all goods and services (and other residential units) in the system. This measure is calculated by summing the shortest paths2 between each cell and all others, and the results are weighted by the population of the area (in this case, the census tract) (Krafta, 2014). This weighting allows for the relativization of accessibility by considering that street segments in more populous sectors are, on average, closer to people and are, therefore, more accessible than segments with identical unweighted values in areas of lesser demographic importance. Equation 1 specifies the calculation of weighted accessibility.
WA i represents the accessibility of entity i; W j corresponds to the attribute (weight) of entity j; and d ij indicates the distance between entities i and j (Dalcin & Krafta, 2021).
The second measure, Freeman-Krafta weighted centrality (hereinafter FK), considers that in the case of two non-adjacent segments, the flows of people between them depend on other segments that intersect in their shortest path(s). This indicator, also weighted by the population, aims to determine the frequency a segment participates in the shortest paths between all the pairs of the system. Krafta's model enhances the one Betweeness Centrality originally proposed by Freeman (1978) by including the notion of tension and dissipation, i.e., population concentration (in this case) creates tension on each roadway segment, which is dissipated by the distance between the origin and destination segments (Krafta, 1994). In other words, a segment with high valued centrality is expected to carry a significant flow of people (pedestrians and/or vehicles), attracting retail and service-related businesses (Krafta, 2014). This can potentially rise land prices for these economic uses. This locational advantage can also increase land prices for nearby residential uses, either due to the greater attractiveness of vibrant surroundings or to increased competition for land. Equation 2 describes FK centrality.
FK k represents the FK centrality of entity k; g ij corresponds to the number of shortest paths between entities i and j; g ij (k) indicates the number of shortest paths between i and j that contain k; W i and W j are the attributes (weights) of i and j, respectively; and d ij indicates the distance between entities i and j (Dalcin & Krafta, 2021).
Both indicators were calculated with a global radius (covering the entire system) and a local radius of five hundred meters [considered by Gehl (2015) an acceptable walking distance limit for everyday activities]. The former detects the main centralities of the urban system, typically associated with occasional or specialized consumption activities, while the latter can reveal local centralities related to the consumption of daily goods and services (Serra & Pinho, 2013), as well as minimize edge effects, or distortions that reduce the values of configurational measures near the boundaries of the system (Porta et al., 2009). Weighted measures of accessibility and FK were calculated using the open-source software QGIS (2021), version 3.18.3, with the Graph Analysis of Urban Systems (GAUS) plugin (Dalcin & Krafta, 2021).
2.Construction of the Spatial Network
The base road network was obtained from OpenStreetMap using the QuickOSM plugin, with the municipal limits of Porto Alegre (RS)3 serving as the boundary. An additional one-kilometre buffer zone was established for calculating measures to mitigate edge distortions. Cells related to pedestrian trails or paths within parks, squares, and hills, as well as parking areas, access roads to private properties, roads within gated communities and within shanty towns were excluded4. All remaining road segments were dissolved and subsequently sectioned using the v.clean tool, part of the GRASS GIS (Geographic Resources Analysis Support System) suite, integrated into QGIS, to ensure that calculations were performed on each road segment. The described process resulted in 33 945 segments. Population data for the 2 433 census tracts in the capital of Rio Grande do Sul were downloaded in QGIS using the “Censo IBGE” (IBGE Census) plugin, which provides data related to the 2010 census. Subsequently, segment weighting was performed by distributing the population value of each tract among its respective road segments. For the measures’ calculation, in the GAUS window, it was selected the "geodetic" analysis type (geometric distances), and, in metrics to be computed, "Accessibility" and "Freeman-Krafta Centrality". For the analysis radius, initially global and then local (500 metres). Finally, the estimated population variable for each segment was included in the segment load.
3. Apartments
The data on apartments available for sale were collected from the real estate portal imovelweb (Imovelweb, 2023). The choice of this tool over others available on the internet is justified by the possibility of controlling the date when the listings were published, as an effort was made to cover the twelve months prior to the survey (from July 2022 to July 2023), avoiding potential seasonal biases that may affect prices. The only search restrictions defined were the transaction type (“Buy”), the property type (“Apartment”), and the location (municipality of Porto Alegre). A stratified sample of four hundred listings was collected, which exceeds the minimum sample size of 317 stipulated to make valid inferences (Rogerson, 2011). Listings were sought to be evenly distributed across the four quarters preceding the survey, i.e., one hundred per quarter. For each property, in addition to precise location, three attributes were recorded: usable area, absolute price, and unit price in Brazilian Reais (R$). Finally, the listings were grouped into three classes based on the absolute price requested: low price, medium price, and high price. These classes act as indirect indicators of the socio-economic profile of the respective residents. Table I summarizes the quantities and proportions of listings collected for each class5.
4. Kernel Density Estimation (KDE) as a tool for data normalization and variable correlation
Given that the road network and apartment prices constitute distinct spatial layers, with values recorded in dissimilar units, both datasets were transformed to the same analysis scale, i.e., were normalized, using the Kernel Density Estimation (KDE) technique. KDE is based on the density of points or lines (locations of a phenomenon) within a spatial window (cell) associated with each observation. For each cell, objects closer to it receive more weight than those further away, based on a Kernel density function (equation 3) (Silverman, 1986).
in which, f(i) is the density at the centre of the cell, h corresponds to the search radius for neighbouring points; w i indicates the weight of point i; d ij , the distance between the point and the centre of the cell; and n corresponds to the number of points within the search radius.
KDE transforms the density of discrete phenomena into a continuous surface (raster), where windows closer to a higher number of phenomena (and to more important phenomena in the case of weighted KDE) receive higher values (Fotheringham et al., 2000). This procedure converts different layers of information into comparable raster surfaces, as they are subject to the same unit. Porta et al. (2009) report that while the choice of different interpolation or rasterization methods does not affect the results of studies correlating spatial phenomena, the interest in KDE lies in its ability to capture phenomena that are not precisely located in space but whose influence is derived from specific characteristics of surrounding areas, simultaneously impacting them, as is the case of configurational measures and apartment prices.
For both layers of information, i.e., configurational measures and apartment location/prices, cells with a ten-metre edge length and a search radius of five hundred metres were established. Each road segment was associated with global and local accessibility and centrality values, weighted by population. When a Kernel function is applied to each segment, the density value on the line is maximum and decreases to zero at the limits of the search radius. In this case, the density corresponds to the number of segments within the search radius multiplied by the weighted accessibility or centrality values. The same applies to the apartment data, with the caveat that the function is calculated based on point populations: the density value assigned to each cell corresponds to the number of points (apartments) within its search radius multiplied by their respective absolute or unit prices. All four configurational layers created in QGIS were imported into ArcGIS, version 10.2.2 (Esri, 2014), in which KDE maps were generated using the “Kernel Density” tool. The eight apartment layers (absolute and unit prices for all apartments and for each price class) were directly created in ArcGIS and transformed into KDE maps following the same procedure described.
Finally, the four configurational layers and the eight apartment layers were related using the Pearson linear correlation coefficient (r), resulting in a total of 32 correlations performed with the help of the “Band Collection Statistics” tool, native of ArcGIS. The Pearson coefficient ranges from -1 to 1, indicating how similar are the variances of two data sets. The closer the absolute value is to 1, the more similar the variability of both sets, suggesting that they are related (a negative r indicates an inverse relationship). When the coefficient approaches zero, the phenomena are considered unrelated, i.e., they are considered independent6 (Ferreira, 2014)..
V. Results and discussions
Figure 1 locates the study area and spatially represents the collected sample of apartments for sale. It is noteworthy that the price gradient decreases from the center - particularly the axis that span from the CBD to the Iguatemi Shopping Mall - to the periphery, where most of the social housing is located.
Figure 2 spatializes the local configurational measures. Global accessibility demonstrates high values diffused throughout the urban area of the state capital, leaving a considerable part of the southern zone relatively on the margins. The peaks of global FK coincide with the layout of the municipality's main roads. The local measures confirm the maximum prominence of the Historic Centre (CBD) and adjacent neighborhoods. Neighborhoods primarily devoted to social housing (and distant from CBD) also constitute significant local centralities, notably in the north, east, and south zones.
Table II presents the descriptive statistics for the configurational measures, while table III does so for the sampled apartments, both for the entire dataset and for each price class.
Figure 3 displays the spatial distribution of apartments weighted by unit prices. The largest concentration of high-priced apartments (both absolute and unit prices) coincides with the expansion vector of high-income residences, between the CBD and the Iguatemi Shopping Mall (seven kilometres east of the former) (Maraschin, 1993). Menino Deus (a neighborhood three kilometres south of the CBD) constitutes the main stock of medium-priced apartments, followed by the eastern vector, previously defined. Finally, low-priced apartments are scattered throughout the urban fabric, although the CBD concentrates the largest stock of this class. In this group, peripheral areas devoted to social housing in the southern, northern, and eastern zones stand out, generally very distant from the CBD and near the boundaries with neighbouring municipalities.
Table II Descriptive statistics for the configurational measures.
Accessg | FKg | Access500 | FK500 | |
N | 31 006 | 31 006 | 31 006 | 31 006 |
X̅ | 200,482 | 1.203e+7 | 10 360 | 75383.375 |
Md | 207,050 | 2.344e+6 | 9 145 | 29079.013 |
s | 59,657 | 2.570e+7 | 7 868 | 148121.907 |
Minimum | 3.698e-9 | 0 | 2.706e-4 | 0 |
Maximum | 328,369 | 3.994e+8 | 62 083 | 1.105e+6 |
Access g : global accessibility; FK g : global Freeman-Krafta centrality; Access 500 : local accessibility (500 metres); FK 500 : local Freeman-Krafta centrality (500 metres).
Table III Descriptive statistics of the apartment sample. Usable area in m² and prices in Brazilian Reais (R$) (July 2023).
DATASET | LOW PRICE | MEDIUM PRICE | HIGH PRICE | |||||||||
l | Area | Abs. price | Unit price | Area | Abs. price | Unit price | Area | Abs. price | Unit price | Area | Abs. price | Unit price |
N | 400 | 400 | 400 | 161 | 161 | 161 | 85 | 85 | 85 | 154 | 154 | 154 |
X̅ | 96.9 | 674 953 | 6 402 | 46.3 | 164 611 | 3 629 | 78.2 | 448 221 | 6 099 | 160 | 1.33e+6 | 9 467 |
Md | 72.0 | 414 950 | 5 425 | 43.0 | 125 000 | 3 385 | 74.0 | 430 000 | 5 607 | 123 | 1.10e+6 | 9 297 |
s | 84.7 | 724 416 | 3 512 | 15.7 | 68 174 | 1 169 | 22.1 | 91 880 | 1 932 | 106 | 787 223 | 3 330 |
Minimum | 18.0 | 48 000 | 1 043 | 18.0 | 48 000 | 1 043 | 38.0 | 309 900 | 2 440 | 45 | 611 589 | 3 347 |
Maximum | 640.0 | 6.50e+6 | 21 715 | 106.0 | 300 000 | 8 833 | 147.0 | 600 000 | 13 684 | 640 | 6.50e+6 | 21 715 |
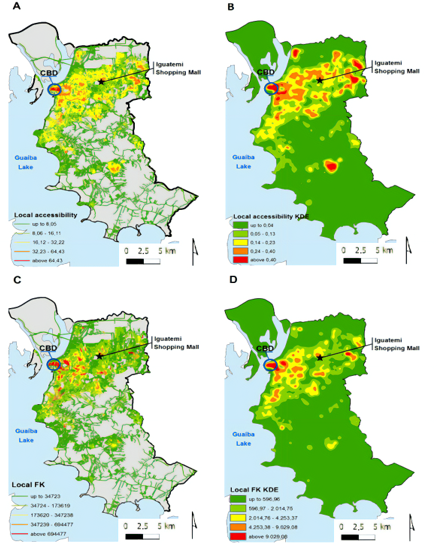
Fig. 2 Configurational measures. A: Local accessibility (network); B: Local accessibility KDE; C: Local FK (network); D: Local FK KDE.
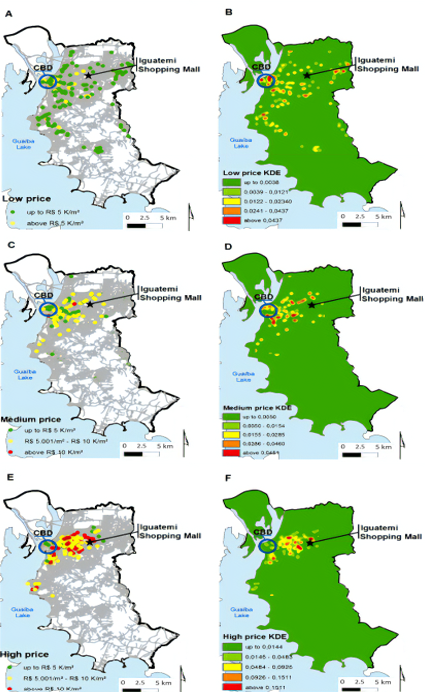
Fig. 3 Spatial distribution of apartments weighted by unit prices. A: low price (up to R$ 300 000); B: low price KDE; C: medium price (R$ 300 001 - R$ 600 000); D: medium price KDE; E: high price (above R$ 600 000); F: high price KDE.
Table IV reveals the 32 correlations calculated between the densities of configurational measures and apartment prices.
Table IV Pearson correlations (r) between configurational measures and apartment prices. All correlations are significant at p < 0,001.
Config. measures | Accessg | FKg | Access500 | FK500 |
AptsA: Absolute prices of the apartment set | 0.406 | 0.221 | 0.390 | 0.431 |
AptsU: Unit prices of the apartment set. | 0.485 | 0.266 | 0.489 | 0.533 |
AptsLA: Absolute prices of low-priced apartments. | 0.419 | 0.261 | 0.467 | 0.504 |
AptsLU: Unit prices of low-priced apartments. | 0.397 | 0.237 | 0.459 | 0.494 |
AptsMA: Absolute prices of medium-priced apartments. | 0.434 | 0.239 | 0.502 | 0.482 |
AptsMU: Unit prices of medium-priced apartments. | 0.434 | 0.239 | 0.497 | 0.480 |
AptsHA: Absolute prices of high-priced apartments. | 0.404 | 0.233 | 0.402 | 0.384 |
AptsHU: Unit prices of high-priced apartments. | 0.432 | 0.233 | 0.438 | 0.413 |
Access g : global accessibility; FK g : global Freeman-Krafta centrality; Access 500 : local accessibility (500 metres); FK 500 : local Freeman-Krafta centrality (500 metres).
First and foremost, it should be emphasized that the KDE method enables correlations of locations weighted by prices and not of prices directly. For this reason, all associations found are positive and significant, as more accessible and central areas tend to concentrate a higher supply of apartments (in other words, they are denser). That being said, we will focus on the comparative examination of values by measure and by price class.
When considering the set of all apartment listings, FK 500 showed the strongest association with prices, followed by Acess 500 , Acess g , and FK g . Therefore, it can be asserted that in Porto Alegre, central and accessible neighborhoods are sought after by potential apartment buyers, which enhances the property's value. This occurrence is presumably due to the proximity to everyday consumption goods and services, besides to environments rich in urban vitality. This result underscores the importance of the immediate surroundings of the property. In this regard, our findings align with Axhausen et al. (2004), Chiaradia et al. (2009), Guo and Bhat (2007), Schirmer et al. (2014), and Zondang and Pieters (2005). The low correlations with FK g , on the other hand, suggest that proximity to heavily trafficked roads, associated with negative externalities such as congestion, noise, and pollution, acts as a constraint on the appreciation of apartments. However, the competition for land in such locations for tertiary uses positively pressures the prices of residential units in multifamily buildings. In this respect, this research unveils processes similar to those identified by Bürgle (2006), Heyman and Manum (2016), Kim et al. (2005), and Palma et al. (2005).
The comparison of configurational measures with apartments by price class explicitly indicates the existence of location preferences that vary according to the socioeconomic profile of the residents. Regarding Access g , it is observed that for low-priced properties, the correlation with unit price is the lowest (0.397), while for medium and high-priced unit properties, the values are close, at 0.434 and 0.432, respectively. These values suggest the presence in Porto Alegre of both imposed segregation and self-segregation processes (Corrêa, 2013). Moreover, apartments with intermediate prices tend to be located in more accessible or closer locations to the set of elements (objects and activities) that constitute the urban system than high-priced and especially low-priced apartments. As mentioned earlier, the latter tend to be located in peripheral areas, within large social housing developments on the city's outskirts.
The examination of correlations involving absolute prices allows us to glimpse a significant component of self-segregation, as the correlation (with Access g ) for high prices (0.404) is even lower than that observed for low prices (0.419), with both being lower than the intermediate group (0.434). It can be inferred that the per square meter price tends to be less valued in less accessible regions (which concentrate low-priced apartments), although a significant portion of these is scattered throughout the urban fabric. Conversely, unit prices are higher in more accessible areas (with more competition for land), despite a large portion of the most expensive apartments seeking more remote (suburban) locations.
Although less highlighted, the correlations between prices and FK g reveal patterns that corroborate the literature. It was found that high-priced apartments are less associated with global centrality than medium and low-priced ones. The obtained correlations are 0.261 for the low-priced group, 0.239 for the medium-priced group, and 0.233 for the high-priced group. A somewhat different pattern is observed with unit prices, where, in addition to less discrepant correlations, the intermediate apartment group shows the highest correlation (0.239), the low-priced group comes in second (0.237), and the high-priced group exhibits the lowest correlation (0.233). Once again, we cite Heyman and Manum (2016) and their warning about the effect of negative externalities from highly central roads at the global scale and their impact on real estate prices. Conversely, the results of this study conflict with Costa (2016), as the author claims that in Porto Alegre, high-end apartments tend to concentrate along highly central roads.
The analysis of configurational measures at the neighborhood level also reveals unequal price patterns. Medium-priced apartments are the most accessible at this scale (correlation between Access 500 with absolute and unit prices of 0.502 and 0.497, respectively), followed by low-priced ones (0.467 and 0.459), and high-priced ones (0.402 and 0.438). In turn, the correlations between FK 500 and absolute and unit prices, in that order, are 0.504 and 0.494 for the low-priced group; 0.482 and 0.480 for the medium-priced group; and 0.384 and 0.413 for the high-priced group. Just as with the global measures, centrally located areas at the local level tend to concentrate commercial and service uses that generate movement, which, on the one hand, provides easy access to a certain variety of daily goods and, on the other hand, is associated with potential inconveniences that may deter some demand for residential properties. Furthermore, it is presumed that for higher-income residents, proximity to places that satisfy such demands is less necessary than for groups whose mobility is hindered by dependence on public modes of transportation or non-motorized travel (Villaça, 2001). For this reason, higher-income groups search for more homogeneous land use locations and, consequently, less crowded ones, while poorer groups seem to be subject to a process even more perverse than imposed segregation, which in this work we call spatial confinement, as they are forced to be close to places that satisfy their daily needs (wherever they are). The results of this study, therefore, disagree with what was found by Axhausen et al. (2004) in Zurich and by Heyman and Manum (2016) in the Swedish and Danish capitals, since in Porto Alegre, more expensive apartments tend to be less close to nodal configurational areas at the local level.
Lastly, it is worth noting that for both local configurational measures, correlations with absolute prices in the low and medium classes are higher than those with unit prices, while the opposite is true for the high class and for the entire apartment set (this last occurrence is also observed for global measures). This situation indicates that areas with high accessibility and centrality have more expensive land, a result of the competition for their locational advantages, even though the apartments located in them are not as expensive in absolute terms. This situation has long been pointed out by urban economists (Alonso, 1964; Chiaradia et al., 2009; Goodall, 1972; Hawley, 1986) which say that, on the one hand, competition for accessible land increases its price, while, on the other hand, transportation costs are much more significant in relation to the budget for economically vulnerable groups than for higher-income groups, immobilizing the former in areas with higher accessibility and proximity to job and consumption locations, at the cost of residing in significantly smaller units (or, not infrequently, in poor condition).
VI. Final remarks
Considering theories on residential location and the interplay between asset appreciation/depreciation and spatial configuration, phenomena like those found in other cities were observed in Porto Alegre. With some nuances, both absolute and unit prices across the entire apartment sample, and through each price class, exhibited positive correlations with the four adopted network measures, particularly the local ones - FK 500 and Acess 500 . In contrast, FK g showed the least association with prices, though the results remained statistically significant.
As a general pattern, locations with greater accessibility (smaller distances to the urban set), and greater centrality (nearer high-order roads) - both on a global and neighbourhood scale - tend to concentrate a larger supply of apartments, including more expensive properties. Nevertheless, this trend reveals significant nuances in the property patterns and, consequently, in the socioeconomic profiles of their inhabitants.
The sampled low-priced apartments (up to R$ 300 0007) showed weaker associations with global accessibility and stronger associations with accessible and central locations at the neighborhood scale. Such a phenomenon suggests the condition of imposed segregation for their populations (Corrêa, 2013), given their limited connection to more central spaces in the city and their confinement to locations that cater almost exclusively to the neighbourhood's population's daily needs. This situation hinders or even prevents experiences of exchanges at higher spatial levels and with social groups of different profiles (Vergara, 2021), a process that has been reinforced by excluding planning legislation and policy, which have tried to impose socio-spatial base on racial and socioeconomic status in peripheral countries (Rolnik, 1997). These apartments, in turn, exhibit a spatial behaviour that competes with areas of higher centrality, whether at the global or local scale. In the first case, very central locations indicate proximity to heavily trafficked roads and, for that reason, are defined by attributes that exclude individuals with higher housing payment capacity, such as noise and atmospheric pollution and congestion. In the second case, mobility restrictions constrain the population of cheaper apartments to reside near centres which satisfy their daily needs, forming what is referred to here as spatial confinement.
The group of medium-priced apartments (between R$ 300 000 and R$ 600 0008) exhibits the highest correlations with accessibility at both scales, although it is in an intermediate position in terms of FK centralities. It can be said that the first effect results, on the one hand, from the high cost of land in more accessible areas, hindering the use of such locations for more modest residential purposes. On the other hand, highly integrated environments are repellent to the portion of the population capable of bearing high housing costs, as they seek more secluded locations.
The spatial pattern of high-priced apartments, on the other hand, confirms, in the capital of Rio Grande do Sul, the trend of self-segregation by elites (Corrêa, 2013, a widespread phenomenon in the global south postmodern metropolises (McFarlane, 2018). While the correlation between the prices of such properties and global accessibility is intermediate when compared to other classes, all other measures, such as connections, demonstrated the weakest relations. This finding suggests that, despite a higher degree of freedom regarding the choice of residential location for individuals or families living in these apartments, they avoid settling in areas characterized by aspects commonly associated with high accessibility and centrality, such as density, diversity of uses, intense pedestrian and vehicular movement, noise etc.
Lastly, this study confirms the capability of configurational measures to capture spatial differentiation in greater detail than geometric distances to the CBD, corroborating Chiaradia et al. (2009). Furthermore, using density correlations instead of measures of point-located phenomena allowed for the consideration of the presence or absence in the surroundings (concentration/dispersion) of attributes that interfere with the studied associations, as previously suggested by Porta et al. (2009). In this regard, although linear associations were applied, future studies may explore other types of statistical correlations and other centrality metrics. Moreover, the temporal limitation of real estate data collection, coinciding with the post-COVID-19 pandemic period and market fluctuations related to the national elections in 2022, may have biased the analyses, and the conclusions require support from future studies. Finally, in general the obtained correlations were not high, which indicates that other factors play a role in apartment prices formation. In this regard, we reinforce that our aim was to analyse just the location configurational attributes. Other studies should apply, for instance, hedonic price models (Xiao, 2017) to control the influence of other factors.