1. Introduction: Is language sexist?
Language is the ability that human beings possess to acquire and use the more than 6500 languages that exist in the world. Thanks to this ability, speakers are not only able to communicate with each other, but also to represent the world around them. This is why our way of thinking and our way of exchanging information are inseparable.
Current literature in neuropsycholinguistics has shown that language may influence various domains of cognition such as memory, attention, and categorisation (for a review see Ibarretxe-Antuñano & Valenzuela 2021). This implies that our way of describing, naming and addressing others also reflects how we see and understand the world. The interplay between language and cognition underscores the importance of conscientiously observing how we articulate the world around us in our communication. This practice is crucial not just for understanding how others perceive reality and from which standpoint, but also for reshaping our own thought processes. It allows us to actively address and dispel preconceptions, stereotypes, or biases that may be ingrained in our thinking, even when we are unaware of them at times.
Language, however, is not an independent mirror of the world: it also constructs and shapes it; language reflects what society is at any given moment. It is is a social construct and, as such, is subject to historical, social and cultural changes (Barker & Galasinski 2001).
These changes in language are everywhere. A few decades ago, terms such as cyberbullying and nomophobia would have been unimaginable in the absence of the so-called Internet revolution. Even today, they may remain unclear for people or societies far removed from the digital world and social networks. Language contributes to classifying and interpreting experience, to constructing and representing identities, and to organising social relations (Butler 2004). In fact, the use or the absence of certain words contributes to the (in)visibilisation and to the recognition and identification of individuals.
Not in vain, in recent decades, society has claimed the need for language to accompany many of the social changes that have taken place. The evolving presence of women in public life and their changing roles has significantly influenced language, prompting speakers to reconsider their linguistic habits. This shift aims to align their speech with the desired egalitarian reality - e.g., the redefinition of alcaldesa (mayor.FEM) from being just ‘the mayor’s wife’ to ‘the mayor herself’, or the incorporation of feminine forms in professions such as jueza (judge.FEM), doctora (doctor.FEM), or ingeniera (engineer.FEM). It is in this context that the concept of “sexist language” emerges, that is, when one gender mark is preferred over the other and, as a result, the presence and contributions of one of the sexes in a particular issue or field is downplayed (Sánchez-Apellániz 2009).
However, the question that immediately arises is this: Is language truly sexist then? Indeed, language is not inherently sexist since all individuals possess the same cognitive tools. Therefore, languages that serve as communication systems cannot be inherently sexist either. What can truly assume a sexist character is the way in which their speakers employ those languages: only speakers and their conscious, and sometimes unconscious, use of their languages is sexist. Hence, it is more convenient to speak of “sexist communication” and its nemesis solution as “inclusive communication”, i.e., a communication that seeks to use language to celebrate human diversity and make people’s rights visible (Alario et al. 2000; Suardiaz 2002; i.a.).
However, sexist communication does not only arise when certain formal elements of a particular language such as gender marking are used. Sometimes, the sexist biases arise implicitly, that is, when the choice of a certain linguistic structure to report an issue or event hides or highlights certain information. This article focuses on the possible biases that arise in the use of language beyond explicit gender marking and their impact on the treatment of language in Artificial Intelligence (AI). The first part reviews sexist biases that arise due to the use of explicit gender-marked linguistic structures (section 2) and those triggered by pragmatic inferencing in unmarked structures (section 3). The second part explores the consequences that these biases may bring into the world of AI, especially in relation to tasks such as programming virtual assistants, machine translation, and sentiment analysis. The language of study in this article is Spanish, a language with gender-marked morphology; however, some of the descriptions and, above all, conclusions are applicable to any language in general with or without explicit marking.
2. Sexist biases in gender-marked linguistic structures
In the field of linguistics, a bias occurs when a speaker employs certain linguistic structures with the intention of discriminating against a particular group in society. This discrimination can be rooted in factors such as sex, race, religion, or any other distinctions based on a particular physical or cognitive condition. In sexist communication, issues such as the use of the generic masculine in Spanish have been highly debated in society. In the past few years, most of the debate has focused on whether the use of this generic masculine triggers sexist biases and on proposing alternative ways to address these situations in a more inclusive manner (see Medina Guerra 2016; RAE 2020).
Unfortunately, much of this social debate on the inclusive masculine shows unawareness of what sexist communication is all about. On the one hand, from a formal perspective, the difference between biological sex and grammatical gender is sometimes blurred (Harris 1991). Sex is a biological trait inherent in some living beings, objectively delineated through hormonal and reproductive characteristics. Grammatical gender is a linguistic resource available in some languages to classify word categories such as nouns, thus facilitating certain syntactic dependency relations. Sometimes grammatical gender coincides with biological sex as in el niño alto (det.MAS.SG child.MAS.SG tall.MAS.SG) vs. la niña alta (det.FEM.SG child.FEM.SG tall.FEM.SG), but this is neither a universal nor a compulsory trait.
There are many languages around the world that lack explicit gender marking (e.g., Basque), as well as languages whose noun classifiers are categorised by means of factors unrelated to sex; noun classes require an understanding of the speakers’ worldview (e.g., Dyirbal) (Corbett 1991; Aikhenvald 2016). In languages where gender is explicit, its assignment and gender types are not necessarily the same. This is why the Autumn is masculine in Spanish but neuter in Greek, and the system is binary (masculine/feminine) in Spanish but ternary (masculine/feminine/neuter) in German, Polish, and Greek. In fact, these differences happen even in genetically-closed languages: the Morning is feminine in Spanish (la mañana), but masculine in Aragonese (lo maitín).
Although gender assignment is not sexist per se, recent literature on Spanish and other gender-marked languages (Menegatti & Rubini 2017; Gygax et al. 2019; Stetie & Zunino 2021) has shown that the choice of a certain gender marking as the generic form may actually trigger certain sexist biased interpretations. The gender the word takes influences the processing of the word and its information. In other words, the use of the masculine as a generic renders women and other non-masculine communities invisible and helps maintaining societal stereotypes such as the division of professions: highly-qualified or stamina/strength-demanding jobs for men (médicos ‘medical doctors’, catedráticos ‘professors’, soldadores ‘welders’, bomberos ‘fire-fighters’, etc.), and assistance and care service jobs for women (enfermeras ‘nurses’, cuidadoras ‘care-givers’, limpiadoras ‘cleaners’, etc.).
Moreover, explicit uses of sexist communication extend beyond gender marking. On many occasions, the specific use of a female/masculine related word conveys a sexist message that reflect societal stereotypes, gender constructs, and evaluative connotations. There are many examples: the pejorative meaning in machirulo ‘tomboy; butch’ and nenaza ‘sissy, mummy’s boy’, absent in their underived words macho ‘male’ and nena ‘little kid.feminine’; the request to provide information about marital status (compare, señora ‘Mrs.’ vs. señorita ‘Ms.’ and señor ‘Mr.’; the positive associations for expressions related to men and male attributes but negative for their female counterparts (esto es la polla [this is the dick] ‘to be awesome’ vs. esto es un coñazo [this is a cunt.big] ‘to be boring’; zorro ‘fox; sly’ vs. zorra ‘vixen; slut’), etc. This list clearly shows the need to discuss sexist communication and find solutions for inclusive language beyond gender assignment.
3. Sexist biases in unmarked gender structures
The sexist biases discussed in Section 2 are well-known by society as well as by scientists; they have been reported in mass media and investigated in different research actions. However, on many occasions, it is the lack of any specific explicit gender or sex mention that contributes to the inference of the sexist bias. These cases are sometimes so natural and ubiquitous that speakers generally are unaware of them. They become even more biased and harmful because these linguistic structures may have an influence on the way we process the world through language, create categories or groups, build knowledge and remember things. Covert sexist communication is powerful because it helps speakers shape their worldview as shown in the following two case studies.
3.1. Two categories for people, two expected ways to behave in society: Kids’ T-shirts and other everyday products
One of the cognitive abilities that humans develop from birth is the ability to categorise, i.e., to group together “elements” that are perceived to be similar. This similarity is based on how many characteristics the candidates for category membership share with the prototypical member, which is assumed to be the best exemplar of the category. In prototype categorisation (Rosch & Lloyd 1979), the characteristics that define the category arise from the categoriser's encyclopaedic knowledge, i.e., the knowledge gained from the categoriser's own experience of the world.
This cognitive ability also applies to language. When we hear a word or group of words, we immediately recall what we know about that word: its meaning in the broadest sense. We retrieve not only its linguistic descriptive meaning but also its contextual meaning and all the information about the category to which the word belongs. For example, the words “be calm, share love and keep smiling” trigger a wellness framework in which calmness, love, and smiles are the feel-good characteristics of wellbeing. If we add to those words the sentence “Inspire others and let’s make the future fantastic”, this wellbeing framework is geared towards helping others. The words “enjoy the vibes”, “be true to yourself” and “create your own future”, on the other hand, send a different message. Here, the wellbeing framework is actually activated for one’s own benefit: it is the list of things a self-made person should do to be happy and successful.
Taken alone, these words are harmless. However, when they are used consistently to refer to two different groups of people, they are not so neutral. They help to categorise one group as the "guardians of well-being" and the other, as the "leaders of the world". This is one of the findings revealed by a study of the messages printed on children's T-shirts. In their study, Pérez-Hernández and Ibarretxe-Antuñano (2022) analysed over 600 T-shirts from the 2022 Spring/Summer collections of a wide range of high street and top designers. They found asymmetries, both at the formal level (structural complexity, types of words, etc.) and at the conceptual level (content), in the text and images that appear on girls’ and boys’ T-shirts.
Messages on boys’ T-shirts had much more text (complex sentences), used the first person and, through advice and suggestions, promoted self-esteem, leadership, individual identity, self-improvement, hedonism, thinking about the future, etc. Messages on the girls’ T-shirts, on the other hand, contained little text (single words), used the impersonal form and advised, through requests, to encourage empathy, sacrifice, calmness, kindness and the common good. Figure 1 shows some examples.
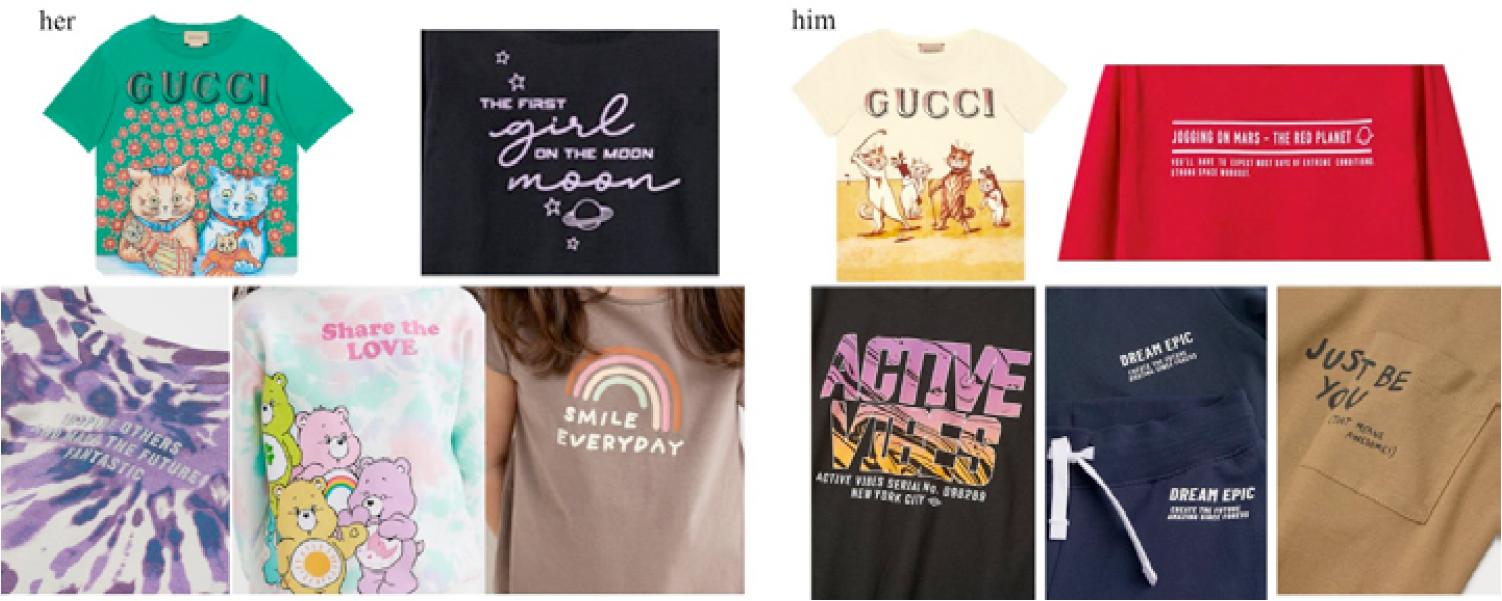
Source: Pérez-Hernández & Ibarretxe-Antuñano’s (2022), Sexist T-shirt corpus.
Figure 1 Covert sexist biases in children’s T-shirts
What is happening with T-shirts is not unique. The same differences can be seen in the messages on other everyday items such as personal care and hygiene products. As shown in Figure 2, women’s skincare creams often carry anti-ageing (forever young) messages. They focus on the benefits that their use can have in terms of concealing the passage of time (antimanchas ‘anti-spot’, antiarrugas ‘anti-wrinkle’) and re-vitalising the skin. Men’s skincare creams, however, emphasise vitalisation (not re-vitalisation) and modernity instead. What these products do is nourish the skin, they are hidratante ‘moisturising’ and, as one personal care brand said in their 2018 ad campaign1, “we can't promise you’ll look twenty years younger but you’ll get better with age”. Similarly, gels and shampoos are described differently depending on the target consumer: for her, they are dreamy products that bring calm and escape; for him, they are energising products, ready for action and sports.
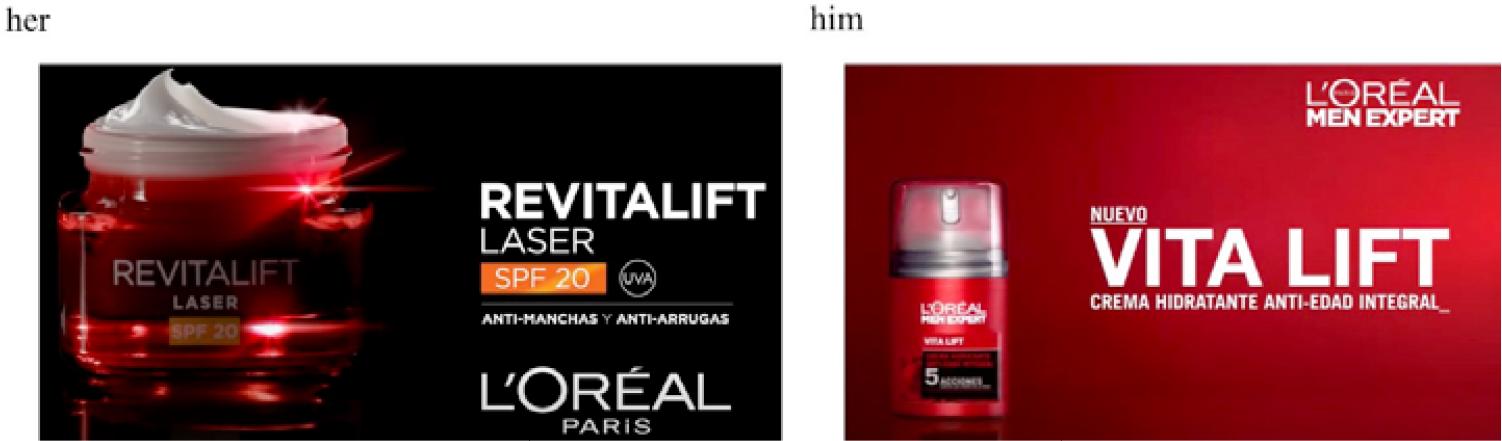
Source: L’Oréal, 21/01/2024 (https://www.loreal-paris.es/revitalift/laser/crema-dia-antiedad-50ml); L’Oréal, 21/01/2024; (https://www.youtube.com/watch?v=cdUOjXqbTuk&t=5s)
Figure 2 Covert sexist biases in skincare products
These examples may seem anecdotal at first sight, but they are not. The seemingly neutral choice of words in these products activates specific “conceptual frames”, i.e., particular shared knowledge structures about the world around us. These frames directly influence the meanings and inferences we attach to the information we receive. In short, this information contributes to categorise women and men not only as different groups, but also as having different goals in life, different functions in society, and different physical needs.
3.2. Overt expressions, highlighted information and missing intentions: mass media headlines and reports
Far from being limited to describing the world, language provides a framework that influences how we interpret and judge what happens in it. The choice of words and their conceptual frames become even more crucial when they are used in the mass media, in newspaper headlines and news reports. The choice of these words in portraying or reporting on characters and events has the power to change the story, to focus our attention on certain facts and hide others, and consequently, to make inferences and value judgements. Knowledge and control of how reality is presented can change public opinion as shown in the examples analysed in what follows.
3.2.1. What we take for granted about people's physical-emotional function and status
One of the most common sexist biases is the assumption of a person's role in life and society and their ability to fulfil that role. In the case of women, this role is traditionally associated with motherhood and homemaking. The headline in Figure 3 reflects what happens when a woman does something other than raise children. This headline was published in Crónica Directo, an online magazine, and refers to Laura Escanes, a well-known Spanish influencer.
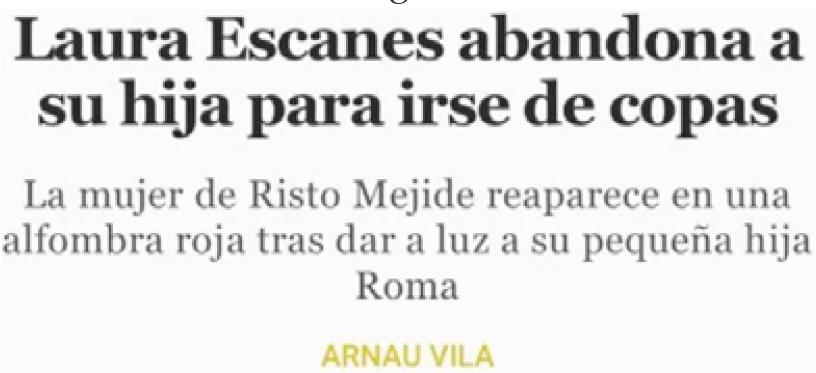
Source: Originally published in Crónica Directo, 19/11/2019; it is now available at https://www.publico.es/tremending/2019/11/20/machismo-machista-y-repugnante-deberia-ser-denunciable-el-articulo-que-asegura-que-laura-escanes-abandono-a-su-hija-para-irse-de-copas/
Figure 3 Sexist bias: feminine role ♦ linguistic bias: verb + final sentence
The choice of a third-person causative verb (abandona ‘abandons’) together with a purpose clause (para irse de copas ‘to go out for drinks’) implies a deliberate and premeditated voluntary act, i.e., this person is responsible for the daughter’s abandonment and is guilty in two ways: firstly, because she is not fulfilling the social role of a woman (parenting) and secondly, because the reason is unethical (partying). The subtitle explains the real situation - it is a business event - but this information is no longer relevant. The “ludic” conceptual frame has already been activated and this woman is no longer categorised as a working person, but as a bad mother.
Two other classic sexist biases are the question of age and the attribution of certain types of emotions. As the discussion on skin care creams showed, age is perceived differently by men and women: age is negative for women, who should stay young, but positive for men, who become more mature. In the case of emotions, in addition to the strong/rational vs. weak/emotional sex distinction, women's emotions are characterised as uncontrolled (i.e., hysteria). Both sexist biases are illustrated in the headline in Figure 4. Together with an image of Queen Letizia of Spain, the headline, published in the digital newspaper El Español, alludes to these two sexist prejudices: women’s excessive concern with ageing and looking good (no son los 47 años ‘it’s not the 47 years [of age]’) and their inability to control their emotions (está de los nervios [is of the nerves] ‘[she] is on edge‘); a lack of emotional control that, thanks to the plural form in nervios ‘nerves’, is close to an emotional breakdown. The headline, which explicitly addresses high-ranking women by their first names, reinforces the ‘apparent’ futility of the reasons for the reported distress with the choice of the words lío ‘mess, tangle, fuss’ and debut ‘premiere, début’ to refer to the complex political situation and the beginning of the princess’s public activity, respectively. These two words activate conceptual frames that are alien to the real professional activity reported in the news: the romance (lío amoroso ‘love affair’) and the show business (debut artístico ‘artistic premiere’).
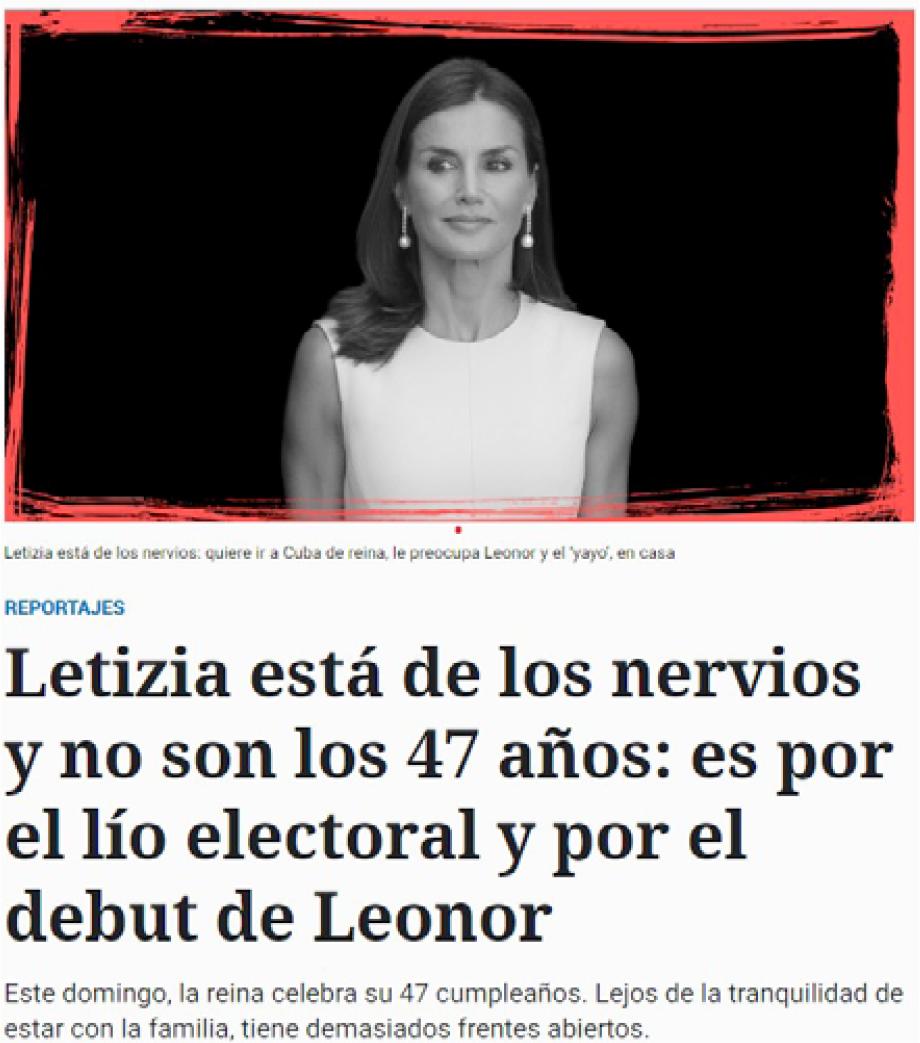
Source: El Español, 14/09/2019 (https://www.elespanol.com/reportajes/20190914/letizia-nervios-no-anos-electoral-debut-leonor/428957667_0.html).
Figure 4 Sexist bias: physical-emotional female ♦ linguistic bias: nouns
3.2.2. The intentionality of reported actions
Agentivity in Spanish refers both to the type of involvement that the agent of a given action may have and to whether that agent performs the action intentionally or accidentally (Ariño-Bizarro 2023). Several linguistic structures are available in Spanish to highlight or hide the agent:
(1)a. Active structure ♦ agent highlighted
El dueño vende el piso
‘the owner sells the flat’
b. Reflexive passive structure ♦ agent unimportant
Se vende piso
‘the flat is sold’
c. Passive structure ♦ agent unimportant
El piso fue vendido rápidamente
‘the flat was sold quickly’
At the same time, Spanish is also rich in resources that mark the degree of involvement of the agent in the action (Ibarretxe-Antuñano 2012):
(2) a. Full intention
Juan ha tirado un vaso
‘John throw away a glass’
b. Intentional but with care
Juan dejó caer el vaso
‘John let the glass fall’
c. Not intentional but happened
Se le ha caído el vaso a Juan
‘John has unintentionally let the glass fall’
All these resources to specify agentivity and intentionality are important in all contexts but even more so in those of sexual harassment and violence, as in Figure 5. The choice of a structure has the power to assign responsibility to the female victim or to exonerate the male perpetrator.
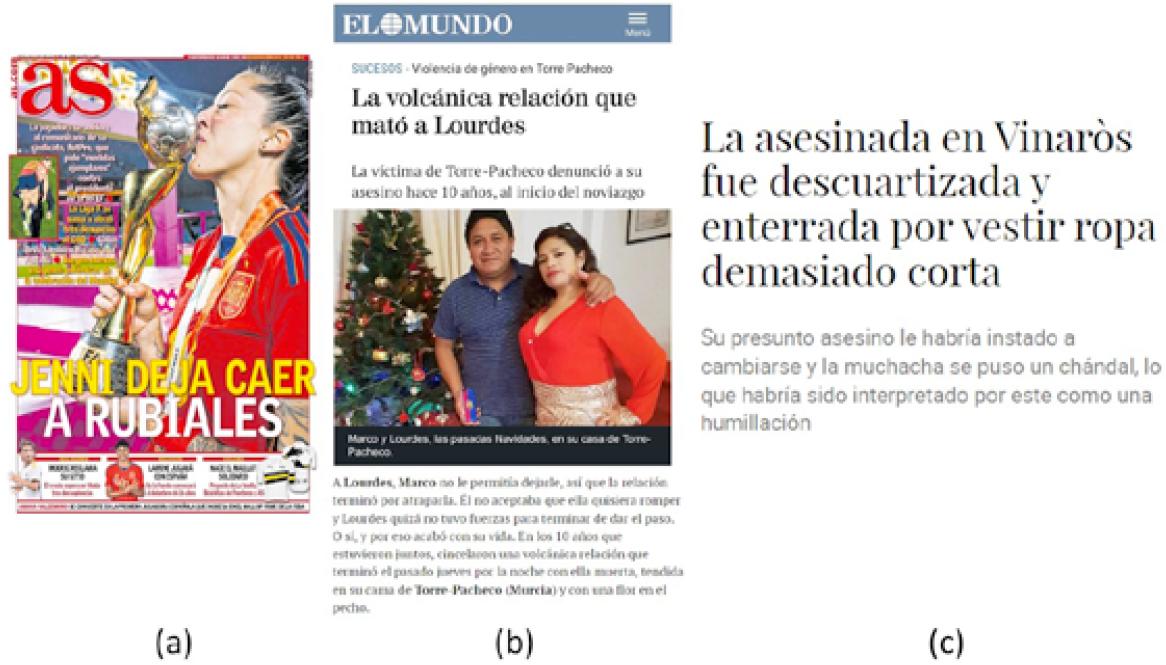
Source: (a) As, 24/08/2023 (https://twitter.com/diarioas/status/1694469882371342452); (b) originally published in El Mundo, 11/05/2019, the news is now available at https://www.pikaramagazine.com/2019/12/machismo-y-medios-lo-peor-de-2019/; (c) El Confidencial, 08/04/2019 (https://www.elconfidencial.com/espana/2019-04-08/la-asesinada-en-vinaros-fue-descuartizada-y-enterrada-por-vestir-ropa-demasiado-corta_1930390/).
Figure 5 Sexist bias: intentionality ♦ linguistic bias: morphosyntactic structures
Figure 5.a corresponds to the front page of the sports newspaper As when the ex-president of the Spanish Football Federation, Luis Rubiales, kissed the professional player Jennifer Hermoso without consent (24/03/2023). Apart from the image of the player’s kissing the trophy, which recalls the origin of the aggression (the non-consensual kiss), and the asymmetrical way of addressing the protagonists (nickname vs. surname), the choice of the morphosyntactic structure, dejar caer ‘let fall’, reveals the sexist bias: (i) a clear agent, Jenni; (ii) an action that requires will, intention, but also care on the part of the agent (see examples in (2) above), and (iii) an object that suffers the effect of the action, Rubiales. All these elements help to exonerate the person responsible for the aggression (Rubiales) and make the player responsible for what may happen to the president in the future.
Figure 5.b reproduces an excerpt from a report on a sexist crime published in the Spanish national newspaper El Mundo (11/05/2019). The relative clause in the headline foregrounds the active agency of the murder. However, the choice of the noun phrase la volcánica relación ‘the volcanic relationship’ as the intentional agent of the murder not only emphasises the explosive (and therefore, already violent, passionate, and unstable) nature of the relationship, but also hides the real agent of the murder, the husband. In other words, the blame goes to an uncontrolled non-human agent. An uncontrolled force that the victim, due to her natural feminine weakness, did not even try to put under control in time (Lourdes quizá no tuvo fuerzas para terminar de dar el paso ‘Lourdes perhaps did not have the strength to finish taking the step’). A death that ended not as the result of an action (a murder), but as a foreseeable state as the past participles muerta ‘dead’ and tendida ‘lain’ suggest (una volcánica relación que terminó el pasado jueves con ella muerta, tendida en su cama ‘a volcanic relationship ended last Thursday night with her dead, lying in her bed’).
The headline from the online newspaper El Confidencial (08/04/2019) in Figure 5.c uses a periphrastic passive clause, a type of structure that draws attention to the patient. It has a patient subject (La asesinada de Vinarós ‘the murdered [woman] of Vinaroz’), the verb ser ‘to be’ with the participle of the lexical verb (fue descuartizada y enterrada ‘was dismembered and buried’), and a complement introduced by the preposition por (por vestir ropa demasiado corta ‘for wearing clothes that were too short’). The consequences of using such an infrequent structure in this headline are clear: the murderer is hidden and the deliberate actions of quartering and burying become fortuitous and accidental. Furthermore, the use of a clause introduced by the preposition por, the preposition for passive agent clauses, raises the reader’s expectation that the person who carries out these actions will be mentioned at the end of the headline. However, this expectation is disappointed as the preposition leads to a causal clause instead.
In short, these headlines did not use explicit gender-biased words: sexist biases arise from the choice of structures. These narrate the events in a way that (i) removes or intensifies responsibility and (ii) justifies the reasons for (in)action. The result is a diminution of the true nature of the crimes committed and their perpetrators.
4. The impact of covert biases in artificial intelligence
Although sexist biases persist in some of the tools and mechanisms that feed natural language processing in AI today, AI has worked hard to weed out these cases and promote a more diverse feed for its robotic algorithms, programs, and designs. However, unmarked cases remain a challenge for AI.
4.1. Voice assistants and gender stereotypes
The first group of unmarked sexist biases has to do with the prejudices that AI designers bring to their products. One illustrative example: the voice programmed for customer service virtual assistants is usually female - from the internationally popular Apple’s Siri, Amazon’s Alexa, Microsoft’s Cortana, to the locally-known Lola at the University of Murcia, the train company Renfe’s Irene or the airline Vueling’s Eva in Spain.
The problem with the choice of gender for these virtual assistants is not so much that it is a female voice; there are also male voices for similar assistance roles (e.g., Max, AXA insurance company agent for roadside help). The problem lies in the perpetuation of a double bias: women are good caregivers, receptionists, and information assistants but when it comes to technology and handy work, it is a man’s world. The choice of these voices for these functions is not arbitrary. The developers follow customers’ preference: female voices are considered more pleasant and preferred for tasks requiring advice, while male voices are judged more appropriate for technical information2.
However, even if potential clients prefer these voices, it is in AI’s hands to help revert this sexist bias. A solution has been to implement gender neutral voice assistants such as Blue, the BBVA’s virtual assistant (Baeza 2020). The defining personality features of this system took into account what users from four different Spanish-speaking countries (Argentina, Colombia, Spain and Mexico) considered important for such a service: coherent, ethical, responsible, and gender neutral.
4.2. Machine translators and gender bias
Another key area for AI is machine translation and the challenge of resolving gender ambiguity. Google’s translator is often cited as an example of the sexist bias that starts in the data and is later replicated by the technology.
One of the first sexist biases identified in machine translators such as Google was the ascribed gender in translations from gender-neutral languages (English) to explicitly gendered languages (Spanish) as in Figure 63.
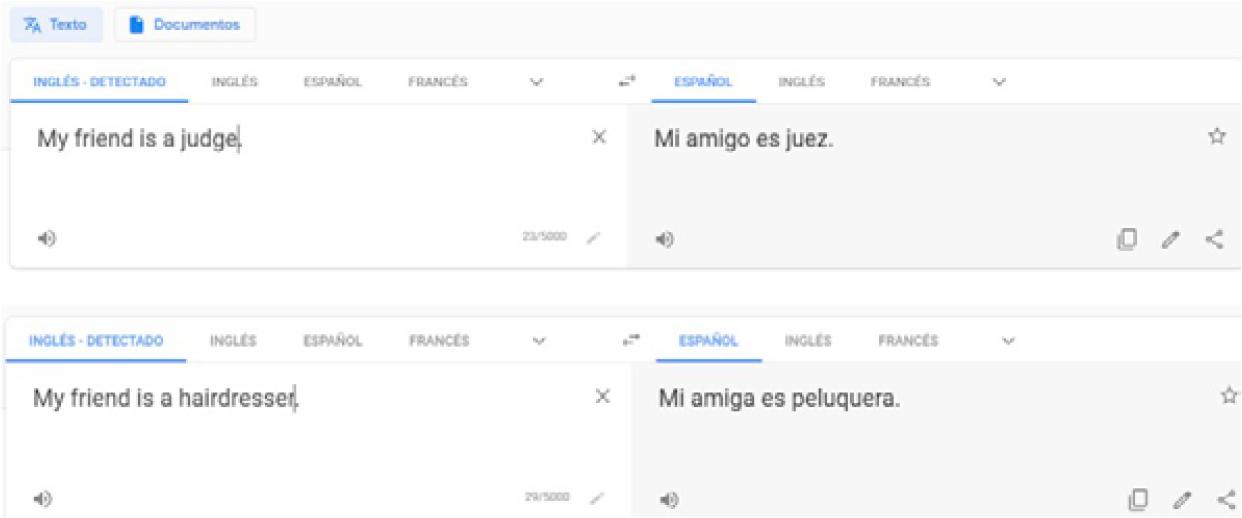
Source: La traducción como Inteligencia Artificial: el futuro aún presenta sesgo de género, 14-05-2020 (https://blogthinkbig.com/sesgo-de-genero-traduccion-ia).
Figure 6 First translation stages in Google
A gender-neutral word like friend in English became amigo ‘male friend’ or amiga ‘female friend’ based on the friend’s occupation: male friends were judges and female friends were hairdressers. Of course, these translations were the result of what the algorithm had learned, and that learning depends on what was fed into the machine (Johnson 2018). This bias was one of the first problems to be addressed and, nowadays, Google translator provides a different solution as shown in Figure 7. The system gives you all possibilities and the reader is the one that has to choose the most appropriate (Kuczmarski 2018; Monti 2020; López-Medel 2021).
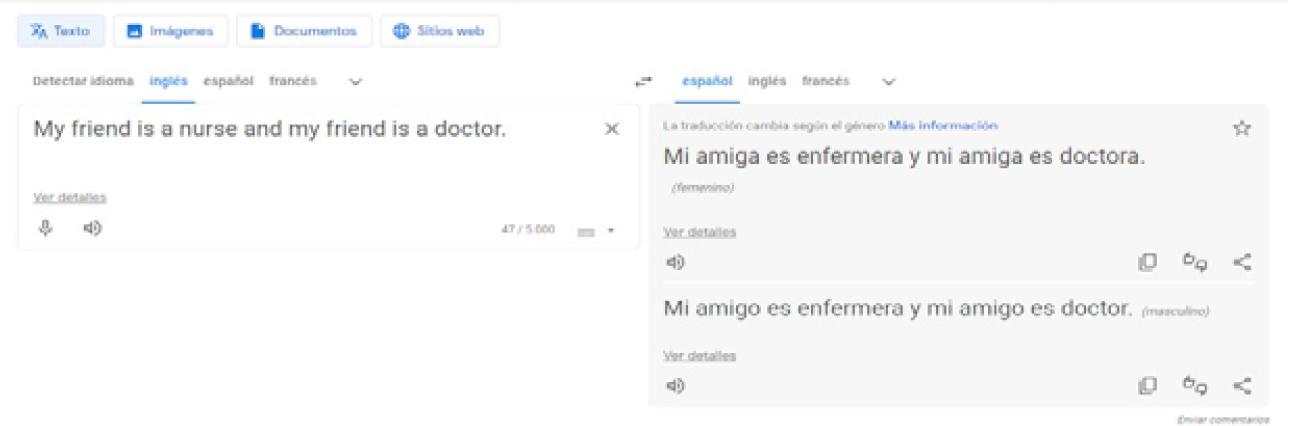
Source: Own source, 23/06/2023 (https://translate.google.es/).
Figure 7 Current Google translations with gender options
It is undeniable that this has led to an improvement in the translation options offered (despite the grammatical agreement mistake - amigo ‘male friend’ requires masculine agreement, enfermero ‘male nurse’ instead of enfermera ‘female nurse’). However, covert sexist biases as those in Figures 8 and 9 are still unsolved. The problem lies, once again, in an asymmetrical grammatical requirement: English clauses always require explicit subjects, whereas Spanish clauses do not. The choice of the subject pronoun she or he in the English translation is based on contextual information: the person who prepares dinner and spends time on personal care is bound to be a woman, and the handy person who likes football is bound to be a man.
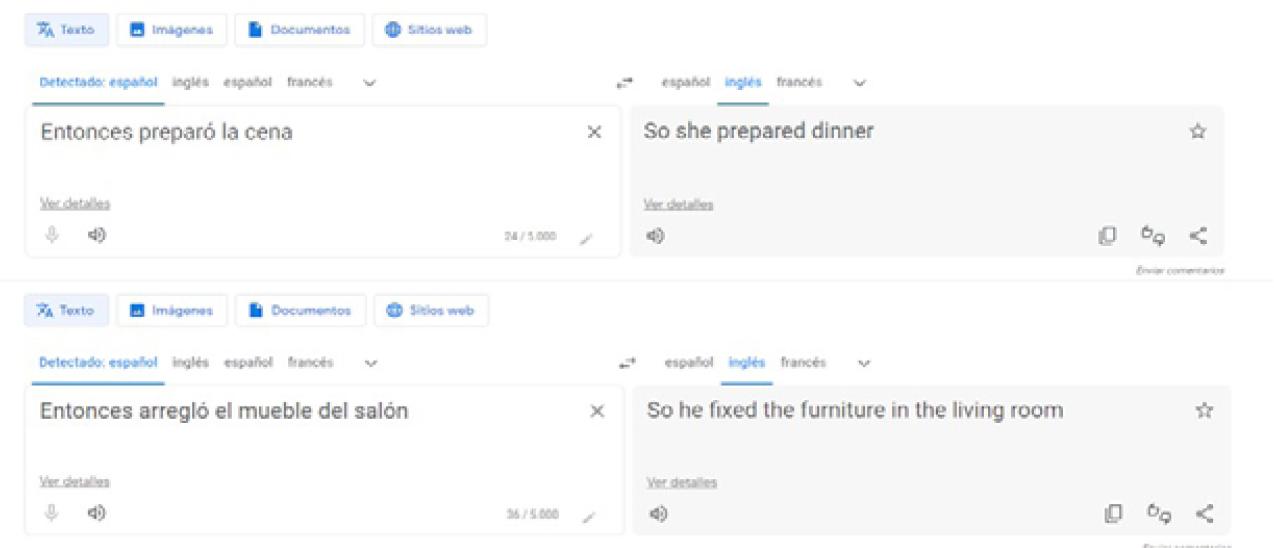
Source: Own source, 23/06/2023 (https://translate.google.es/).
Figure 8 Google translations: sexist biased based on household chores
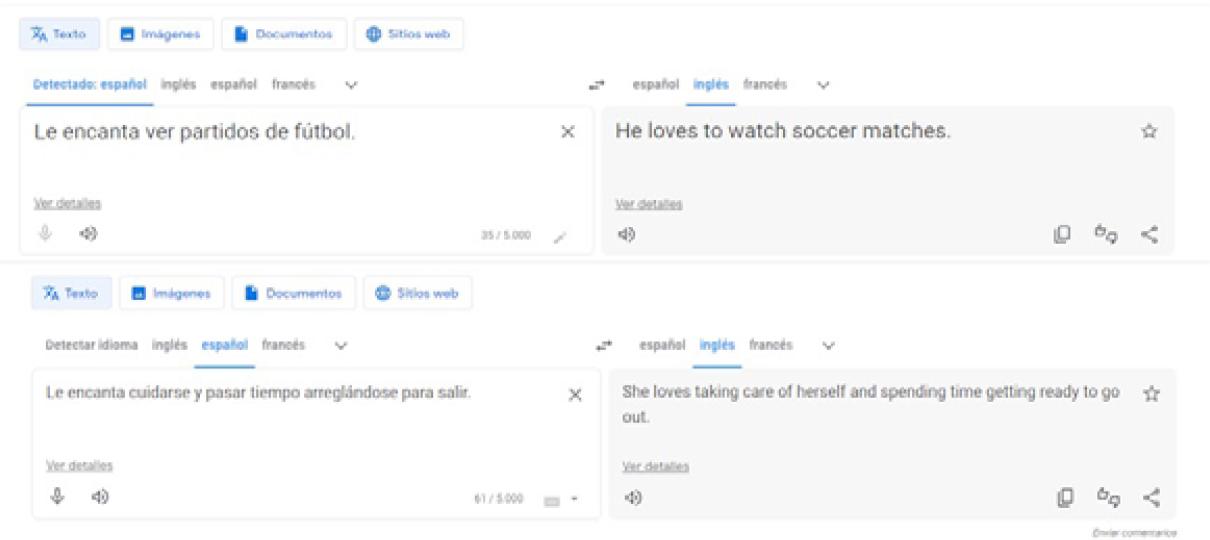
Source: Own source, 23/06/2023 (https://translate.google.es/).
Figure 9 Google translations: sexist biased based on hobbies
Translation systems have now improved their performance, in particular by focusing on promoting fairness and reducing bias in machine learning. However, the challenge is to feed these systems with a wider range of examples in order to cover the real cultural and linguistic diversity of the world there is still a long way to go (Nur Fitria 2021).
4.3. Lexicons and biased sentiment analysis
The last area concerns the sentiment analysis tools in IA. Sentiment analysis, also known as emotion analysis or opinion mining, is a natural language processing (NLP) technique used to determine and evaluate emotions, attitudes, opinions and sentiments expressed in text, whether in the form of opinions, comments, reviews, social media posts, emails or other types of written content (Liu 2015). The main goal of sentiment analysis is to understand the general attitude of a text or dataset in relation to a specific topic.
To work with sentiment analysis tools, it is common practice to create “lexicons”. These are sets of words and terms that have been previously tagged with their polarity, i.e., whether a word expresses positive (e.g., the word birth), negative (e.g., the word death) or neutral (e.g., the word table) sentiments. Some lexicons (Strauss & Allen 2008; Hinojosa et al. 2016) may also include information about the intensity of the polarity (e.g., high positive sentiment for birth) and/or specific emotions associated with the words (e.g., birth is associated with joy).
Despite the usefulness of these lexicons, the way they are built is problematic and the origin of many sexist biases. One of the first problems is the lack of adaptation to the formal and conceptual needs of the target language. For most of the AI tools, the reference language is English and the procedure is simply to machine-translate the English lexicons into other languages (Mestre-Mestre & Díez-Bedmar 2022). As discussed in Section 4.2, machine translation falls short in spotting and avoiding sexist biases, especially in those cases where the gender is not explicitly marked. However, the problem here is to assume that every language works as English and that all speakers share the same worldview, that is, all cultures and societies are guided by the same principles and values as English-speaking countries (Ariño-Bizarro & Díez-Bedmar forthcoming). The result of this process is a collection of sentiment lexicons with ingrained sexist biases. For example, looking at the iSal2vm lexicon (Plaza-del-Arco et al. 2018) it is easy to find examples where gender is assigned to words based on assumed sexist roles. Adjectives such as bueno ‘good’, guapo ‘handsome’ and carismático ‘charismatic’ are in masculine. One might argue that these are examples of the inclusive masculine, but this argument is not valid when words such as histérica ‘hysterical’, cuidadora ‘carer’ and maquilladora ‘make-up artist’ are also found on the same list.
Another crucial problem with these lexicons is the validation procedure. In most cases, these word lists are not validated by external informants, i.e., speakers of the target languages, and even when they are, the basic scientific requirements of this type of psycholinguistic validation (e.g., number and diversity of participants) are usually omitted (Ariño-Bizarro & Díez-Bedmar forthcoming). The result is a lexicon that reflects the values of only a small proportion of the population - that is, the values of the developers, who tend to be male, white and Western.
Another example from the iSal2vm lexicon illustrates this bias. Terms such as giving birth are grouped into a single emotion: joy. The same happens with concepts such as abortion, which is only included in the category sadness. No one denies that the experience of bringing a new life into the world might be rewarding and joyful, but the actual moment of birth is not necessarily joyful for speakers of different sexes, ages and social contexts. For a woman, childbirth can be joyful, but also painful, because it is a demanding natural process. Similarly, an abortion can be sad, but also risky for the woman’s life. Word-meanings are grounded in our experience and as such, these words in lexicons need to be validated by as many diverse people as possible.
5. Conclusions
The debate about sexist biases in language and communication is an issue of undeniable relevance. While it is true that the use of inclusive language is central to avoid sexist communication, it is essential to recognise that these issues go far beyond the use of a generic masculine form. They also extend to various forms of communication that can perpetuate gender stereotypes, which then permeate and are perpetuated in AI.
Sexist language in the mass media can have a profound impact on perceptions of gender roles and how we view people and their achievements. These messages not only reinforce harmful stereotypes, but also limit equal opportunities and perpetuate inequalities.
In AI, sexist biases have also crept into natural language processing algorithms and models through a vicious circle of biased information recruitment or a lack of adequate empirical validation. These biases can have significant consequences, from mistranslations to biased sentiment analysis. It is imperative that the AI research and development community strive to identify and mitigate these biases to ensure gender equity in AI applications.
Awareness is the first step towards improvement. Education, the promotion of inclusive communication practices, and the implementation of policies and standards that address sexist bias and promote diversity and gender equality, is the only way towards a more equitable society.