Introduction
According to GLOBOCAN 2020, gastric cancer (GC) is the fifth most frequently diagnosed neoplastic condition worldwide, being the fourth most lethal,1 with a higher prevalence in the male gender.2 Its incidence exceeded one million new cases along 2020, with an estimated absolute mortality of 769.000 deaths,1 which is thought to be related to its delayed diagnosis.
One of the major prognostic factors for GC concerns disease’s stage at the time of diagnosis/surgical intervention, as deeper neoplastic invasion of the gastric wall is associated with worse outcomes.3 Initial GC staging requires both thoracic and abdominopelvic computed tomography (CT) scan and, in patients with suspected distant metastatic involvement, staging laparoscopy with concomitant cytological analysis of the peritoneal fluid is appropriate.4,5
Surgical resection is the first-line treatment for resectable GC. Nevertheless, in patients with locally advanced disease, it has been shown that perioperative treatment improves survival rates amongst patients with resectable GC when compared to surgery alone.6
In 2010, The European Working Group on Sarcopenia in Older People (EWGSOP) defined sarcopenia as a progressive syndrome characterized by general loss of skeletal muscle mass and overall strength, which may lead to adverse outcomes such as physical disability, worse quality of life and, ultimately, death.7 The EWGSOP states that both reduced muscle mass as well as decreased muscle function (strength or performance), are enough to diagnose sarcopenia.7
In 2018, the EWGSOP group assembled again (EWGSOP2) setting out a new definition for sarcopenia, modifying its diagnosis criteria. In this revised definition, sarcopenia is no longer solely connected with older individuals, and is now known to begin earlier in life.8 Reduced muscular strength is the primary criterion for sarcopenia, apart from being the most accurate indicator of muscle function nowadays. Sarcopenia is diagnosed if, besides reduced muscle strength, there is also decreased muscle quantity or quality.8
Currently, there is a wide array of techniques available for the diagnosis of sarcopenia, ranging from anthropometric and bioimpedance analyses to imaging methods.
With regards to fat volume and lean body mass calculation, bioimpedance analysis (BIA) constitutes a useful tool, as it provides an estimate of muscle mass based on whole-body electrical conductivity.8
On the other hand, anthropometric measurements (AM) are a valuable tool for the initial assessment of sarcopenia in children and young adults, specially in primary care settings where alternative techniques are often unavailable. However, AM value in the elderly is uncertain as progressive changes in fat deposits and skin flexibility provoked by aging, contributes to inaccuracies in body composition estimation, rendering it as an inaccurate method for older and obese patients.9
Finally, both CT, Magnetic Resonance Imaging (MRI) and Dual-energy X-ray Absorptiometry (DXA) are imaging methods that have been used to measure muscular mass.
Photon transmission at two different energy levels is the basis of DXA, resulting in differential attenuation of bone, fat and lean tissue. Predicated in this assumption, various attenuations visualized in tissues, at different X-ray energies, enable several measurements such as lean body mass (LBM), fat mass (FM) and bone mineral mass (BMM).10 The results are later extrapolated to the whole body.11
Both MRI and CT are regarded as gold-standards for non-invasive muscle quantity/mass evaluation.8 Even though the theoretical basis for MRI and CT measurements is the same, MRI has the major advantage of allowing body composition analysis without radiation exposure.11 In these imaging methods, the third lumbar vertebra (L3) landmark is often used in cross-sectional body composition analysis, and it is found to correlate with whole-body tissue measurements.12,13Both the psoas, paraspinal muscles (erector spinae, quadratus lumborum) and abdominal wall muscles (external and internal obliques, transversus abdominus, rectus abdominis) are visualized at this vertebral level, turning it into an excellent reference point for skeletal muscle measurement.11
Alternatively, visceral fat quantification has been suggested as an alternative diagnostic tool for sarcopenia, comprising measurements at several anatomic levels as it is known that sex, age and body level influence the amount of body fat. Nevertheless, several studies have failed to establish meaningful clinical associations between visceral fat measurements at different lumbar/mid waist levels and patient outcomes.11
In recent studies, sarcopenia was associated with worse long-term and short-term prognosis in patients with GC who underwent surgery.14,15Yet, discordant results regarding this topic have been reported, hence it is of major importance to pursue sarcopenia analysis in order to enable a better understanding of its impact on patients, ultimately allowing the establishment of newer strategies to reduce its incidence.
Methods
Population:
A retrospective, observational, longitudinal and descriptive analysis was conducted, comprising all adults with GC who underwent surgery in Hospital of Braga between 2008 and 2016, resulting in a sample of 147 patients. Patients who died within 30 days following surgery and patients with alternative cancers diagnosed in the following 5 years were excluded from this study. Patients without preoperative abdominal CT imaging were also excluded.
The collected data included gender, age, year of surgery, tumour-nodes-metastasis (TNM) staging, length of hospital stay, postoperative complications and overall survival (OS). Body’s composition encompassed total abdominal muscle area (TAMA) at L3 level and patients’ height (these two measures were accessed only to enable calculation of skeletal muscle index (SMI)).
SMI calculation and Image analysis:
Following clinical and laboratorial data collection, access was granted to the patients' thoracoabdominal-pelvic CT scans in order to get cross-sectional images of the inferior aspect of L3. These images were gathered and saved using DICOM (Digital Imaging and Communications in Medicine) format.
The “National Institutes of Health ImageJ®”16 software was used to calculate the TAMA, following image saving.
For every patient, images were firstly adjusted to their original grey colour (using the [-250, -250] Hounsfield Units (HU) threshold) as it allowed better visualization of anatomical structures16. Then, the outer muscle perimeter was manually drawn and the limits switched to [-29, +150] HU, the threshold for skeletal muscle, followed by outer muscle area determination.16,17An identical process was performed to determine the inner muscle area, as it is illustrated in figure 1.
In order to calculate the TAMA, the inner muscle area was subtracted from the outer muscle area and divided by 100, to obtain results in square centimetres. Later, patients’ SMI calculation was performed by dividing the TAMA by the square of their heights.16
For the 45 patients with unknown height (with no information in their electronic medical record), the SMI was determined using the mean height of each gender in the Portuguese population (women:1.63m; men:1.72m), reported in worldwide research from 2016.18
The sarcopenia cut-off used in this study was the Prado et al. cut-off, which defines sarcopenia as a SMI ≤38.5cm2/m2 for women and ≤52.4cm2/m2 for men.19
Statistical analysis:
Categorical variables are presented as absolute frequencies (n) and percentages (%). Quantitative variables were submitted to normality tests evaluation. Normally distributed variables are characterized by mean (M) and standard deviation (SD), while non-normally distributed data are presented as median (Mdn) and interquartile range (IQR).
Statistical tests used include:
To report survival analysis, we used the Kaplan-Meier method and the Long Rank test to look at OS - defined as the period of time between surgery and date of death.
Univariate and Multivariate Cox Regression were used to evaluate if explored variables were significantly independent risk factors for OS. Results are reported as Hazard Ratio (HR) and Confidence Intervals (CI).
Statistical significance was considered when confidence interval was 95% and p ≤0.05.
The statistical analysis was performed using IBM® Statistical Package for the Social Sciences (SPSS®) - version 26.0.
Results
Study population description:
As indicated above, a sample of 147 patients was selected for this study, of whom 60 (40.8%) were women and 87 (59.2%) were men, with a mean age of 66.03 years (SD=11.51). When we looked for sarcopenia in this study population, 32 patients (21.8%) were found to be sarcopenic. The average length of patients’ hospital stay was 10 days (IQR=4), ranging from 2 to 60 days.
When analysing tumours TNM staging, it was found that 4 (2.7%) tumours were classified as in situ, while 77 (52.4%) patients had stage I cancer, 36 (24.5%) had stage II and 30 (20.4%) were diagnosed with stage III cancer. Postoperative complications occurred in 52 (35.4%) of the 147 sampled individuals.
Regarding SMI, in women, a mean of 47.2 cm2/m2 was obtained with a SD of 1.13, whereas in men, a mean of 57.7 cm2/m2 with a SD of 0.86 was registered. In the entire sample, SMI was found to range between 24.97 cm2/m2 and 84.20 cm2/m2 (Table 1).
Association between sarcopenia, patient’s gender and postoperative complications
In the sarcopenic patient’s subgroup, eight (25%) out of 32 were women, while the remaining 24 (75%) were men, and a statistically significant association between gender and sarcopenia’s incidence was found (χ2(1) = 4.24; p=0.040; Φ =-0170) (Table 2). Furthermore, the occurrence of any postoperative complication during hospitalization (χ2(1)=10.3; p=0.001; Φ=0.265) also showed to be significantly different between sarcopenic and non-sarcopenic patients (Table 2). In contrast, there was no statistically significant difference between the two groups regarding age (t(145)= -1.59; p=0.114), either in sarcopenic (68.9±2.15) or non-sarcopenic subgroups (65.2±1.05) (Table 2). Figure 2 shows the difference on computed tomograms between a sarcopenic patient and a non-sarcopenic patient.
Table 2: Statistical analysis of the association between the presence of sarcopenia with the patient’s gender and the occurrence of any postoperative complications.

Association between sarcopenia and length of hospital stay
Considering the duration of hospital stay in both groups, we observed that the median in the sarcopenia group (Mdn=15.5; IQR =12) was 5.50 days longer than the non-sarcopenia group (Mdn=10; IQR=2), representing a statistically significant difference between the groups (U=651; p<0.001; r=-0.465) (Table 3).
Survival Analysis:
With regards to survival analysis, it was found that the OS of sarcopenic patients was lower than OS of non-sarcopenic ones, specifically with a mean of 74.1 months for the first group (Std = 8.15; CI [58.1; 90.1]) and 91.9 months for the second one (Std = 4.75; CI [82.5; 101]) (Table 4). Moreover, this difference in total OS time between groups was found to be statistically significant (χ 2 (1) = 4.88 p=0.027).
Table 4: Kaplan Meier analysis comparing the sarcopenic and non-sarcopenic patients’ overall survival.
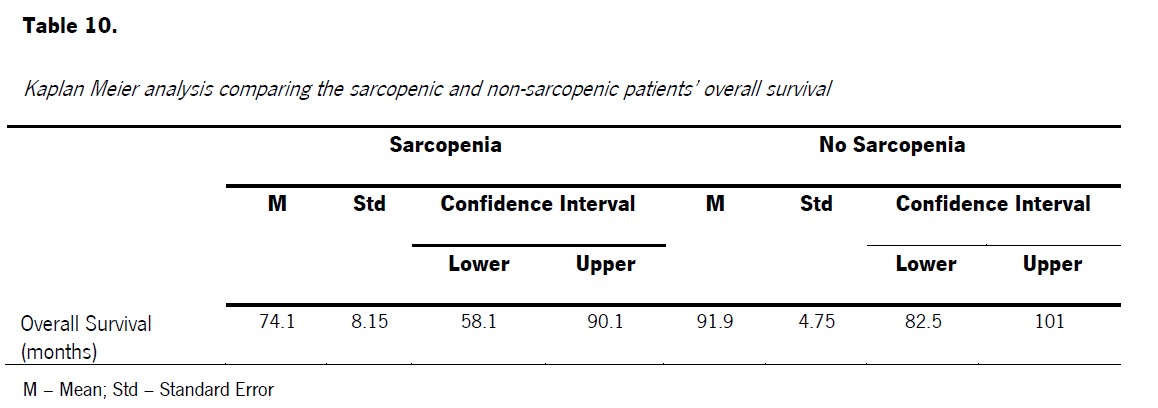
Several variables were associated with worse OS, including age (p<0.001), length of hospitalization (p=0.048), TNM stage (p<0.001) and sarcopenia (p=0.03). Of these, only age (p<0.001) and TNM stage (p<0.001) proved to be independent risk factors for worse OS, as length of hospitalization and sarcopenia are related to patient’s OS however they can’t be considered as independent risk factors. This means that each of these predictors effect was correlated when introduced into multivariate analysis. Statistically significant differences were not detected regarding gender (Table 5).
Discussion
The emphasis on sarcopenia and its effect on oncological patients has led to further research on the subject over the last few years.
Considering sarcopenia prevalence, our study documented a higher percentage of sarcopenic patients (21.8%) than Wang et al. (12.5%), Chen et al. (12.8%) and Zheng et al. (14.9%).22,23,24In contrast, our percentage of sarcopenic patients was lower than the ones reported by Tegels et al. (57.7%), Shi et al. (24.4%) and Zhuang et al. (41.5%).25,26,27This disparity is probably explained by the varying sarcopenia cut-offs applied in different analyses. Tegels et al., for example, employed the Martin et al. cut-off, which includes Body Mass Index (BMI) and sex-specific cut-off values.25,28This cut-off proved to be ideal for predicting survival in patients with advanced GC, but it couldn’t be applied to our study since we didn’t have access to the patients BMI.28 Therefore, we used the Prado et al. cut-off, which has been used in previous studies to assess the prognostic value of CT-based sarcopenia in solid tumours,28 in addition to being based on western population’s characteristics.27
In terms of clinical data, only the patients' gender was found to have a significant association with sarcopenia, implying that it is more common in men. This finding is consistent with several previous analyses.24.26,29,30
Looking at postoperative complications as well as length of hospital stay, previous studies revealed significant differences between sarcopenic and non-sarcopenic patients, which correlates with our results.22,23,26,31
In terms of survival, we observed that non-sarcopenic individuals had an average survival time 17.75 months longer than sarcopenic patients, which was found to be statistically significant (p=0.027), and in line with findings reported by Kudou et al., Zhuang et al., Kuwada et al., Tamandl et al..14,27,32,33When using univariate and multivariate analyses, we realized that, although sarcopenia had a significant impact (p=0.03) on outcomes, it cannot be considered as an independent risk factor for poorer OS (p=0.413). This particular finding differs from what was observed by Lee et al. and Kuwada et al. who noticed that sarcopenia could be held as an independent risk factor for worse OS, in addition to having significant impact on outcome.30,32 While Black et al. found no evidence of an association between sarcopenia and lower OS.34
The discrepancies detected when analysing different variables in various studies, highlight the need for further research in order to obtain more conclusive results, allowing implementation and adjustment to clinical practice of new approaches for sarcopenia prevention in oncological patients.
Our study had some limitations, as we did not have full access/availability to patients’ information, namely patients' heights, so in specific cases an average measure was used instead, which may have influenced sarcopenia diagnosis in these patients. Additionally, perimeters obtained from CT-scan for calculation of SMI were manually drawn, which allows for possible operator-dependent measurement errors. Finally, we also point out that this is a retrospective study, which precludes the use of other parameters included in sarcopenia definition, low muscle strength and low physical performance, which could have aided us in establishing more accurate diagnoses.
Conclusion
In conclusion, we would like to emphasize that sarcopenia, in patients with GC who underwent surgery, has an impact on postoperative complications, length of hospital stay and OS, albeit it cannot be considered an independent risk factor for poorer OS.
With this in mind, we believe that some interventions, such as personalized physical exercise and nutritional monitoring, should be considered while managing these patients in order to prevent sarcopenia development.
Since all patients with GC undergo preoperative staging CT, possibly sarcopenia assessment can become a common practice in this population, as several studies, including our own, have demonstrated its impact on patient’s outcomes. Bearing that in mind, we propose the creation of an artificial intelligence software to automatically measure sarcopenia, reducing potential operator-dependent errors and facilitating its use in clinical practice.