1. Introduction
With almost 2.9 billion active users, Facebook (the platform) is the largest social media in the world. In 2020, people spent 38 minutes per day on the platform on average. The average time has been declining for years now, but it is still evident that Facebook is an integral part of many people’s lives. People use it to stay in contact with their family, friends, and colleagues, and also share their life events, ideas, and values with other people. But the platform has also been criticized for various things, including aggressive collection of personal data, publishing and spreading of fake news (Rai, 2021), incitement of violence (Miles, 2018), and even war crimes (Mackintosh, 2021).
Facebook inc. (now Meta Platforms) has been in the eye of the storm since, Frances Haugen, one of its employees’ disclosed tens of thousands of company-related internal documents to the Securities and Exchange Commission and The Wall Street Journal in September 2021. The documents showed that Facebook has had trouble dealing with growth, disinformation, and moderation. The New York Times declared in “As Facebook grew, so did the hate speech, bullying and other toxic content on the platform.” (Frenkel et al., 2018) Researchers, journalists and activists have stated that the platform has been used as a propaganda instrument in countries such as Myanmar, Afghanistan and Ethiopia (Frenkel et al., 2018; Mackintosh, 2021; Scott, 2021). Some of the problems have been identified by Facebook inc.’s internal research teams, but many of these warnings have been ignored by the company’s executives.
In recent years, many platform designers have come forward about some of the design choices regarding user engagement. One designer admitted that the systems they have worked on cause addiction by design and exploit negative “triggers” (P. Lewis, 2017). Ex-Google design ethicist Tristan Harris has explained that Facebook triggers our base impulses with clever user interface design such as notifications and “Like” buttons (Bosker, 2016). Confessions made by the designers is also supported by the research done on the topic - social media is a platform where negative content is distributed farther and faster (Vosoughi et al., 2018). Algorithms that are built on incentive structures and social cues amplify the anger of users on social media platforms (Fisher & Taub, 2018). These “design tricks” are referred to as dark pattern designs - design choices that modify the design space or manipulate the information flow. These design choices deliberately “trick” users to make things they did not intend to do.
This paper explores two research questions:
RQ1: What type of dark pattern designs Facebook inc. has applied to their site’s recommendation algorithm and News Feed?
RQ2: How does Facebook’s public relations (PR) contradict with their internal work culture and user interface design?
The importance of this research stems from the fact that Facebook inc.’s social media platforms Facebook and Instagram have already become an integral part of our everyday life. Instagram has 1.4 billion monthly users, whereas in case of Facebook this number is as high as 2.9 billion. In addition to people discussing day-to-day topics, they are also used by companies of all sizes for communication, advertising and informing of (potential) clientele.
The design of platforms and software shapes and affects our behavior in digital spaces (Munn, 2020). This research adopts a design-centric approach, and the initial hypothesis is that many of the design choices made by Facebook inc. and introduced in this study are deliberate and prioritize user engagement over safety. Many of these choices are also in conflict with Facebook inc.’s mission statement and core values. Yet, as the leaked documents show, these adjustments were accepted by the company executives and done knowingly and willingly.
This work supplements the work that has already been done in both dark pattern design and on the effects of active use of social media platforms on both individual and societal level. In most examples and references to Facebook inc., this study references to documents leaked by the whistleblower Frances Haugen. As the Facebook Files (also referred to as Facebook Papers) have not been made available for academics, this work often references to news articles regarding the topic. At the time of the writing, Gizmodo is working together with several universities to make the files public (Dell et al., 2021).
2. Related work
Dark patterns
The term “dark patterns” was first introduced by Harry Brignull on the website darkpatterns.org (Brignull, 2018). He defined it as “tricks used in website and apps that make you do things that you didn’t mean to, like buying or signing up for something.” After this the phenomenon was also targeted by academia, who have tried to come up with an official definition ever since. For example, Brignull (Brignull, 2018) and Waldman (Waldman, 2020) called them design “tricks”, whereas Bösch (Bösch et al., 2016) referred them as “misleading” interfaces. What comes to the interface designer, Gray et al. (Gray et al., 2020) said that with dark patterns, the designer abuses their domain-specific knowledge of human behavior. Already back in 1998, Fogg defined a similar concept with “persuasive technologies”, where the designer could intentionally influence users. (Fogg, 1998) A common problem in the literature regarding dark patterns is the lack of specificity. For example, what constitutes a trick? What makes user interfaces misleading?
Dark patterns have been used and studied in multiple domains, including in video games (Zagal et al., 2013), mobile apps (C. Lewis, 2014), and even in home robotics systems and their “cuteness” (Lacey & Caudwell, 2019). Dark patterns have also been studied in the context of user privacy. For example, Bösch et al. (Bösch et al., 2016) introduced a pattern called Bad Defaults, where “the default options are sometimes chosen badly in the sense that they ease or encourage the sharing of personal information.” A Norwegian watchdog group blamed Facebook and Google for using dark patterns that pushed the users toward less privacy (Forbrukerrådet, 2018). After the introduction of GDPR, cookie banner notices become ubiquitous in the web. Utz et al. (Utz et al., 2019) studied a random sample of these banners, and found that over half of them contained at least one dark pattern, including privacy-unfriendly default choices, hidden opt-out choices and preselected checkboxes for allowance of data collection.
Mathur et al. (Mathur et al., 2019, 2021) have suggested a set of shared higher-level attributes as an attempt to organize the dark pattern literature. These attributes are listed in Table 1, and more extensive descriptions can be found in Mathur et al. (Mathur et al., 2021). Asymmetric, covert, or restrictive patterns, or those that involve disparate treatment, modify the set choices available to users, thus attempting to influence their decisions. Deceptive and information hiding patterns influence user’s decisions by manipulating the information that is available or visible to them.
Table 1 Higher-level dark pattern attributes grouped based on how they modify the user’s choice architecture.
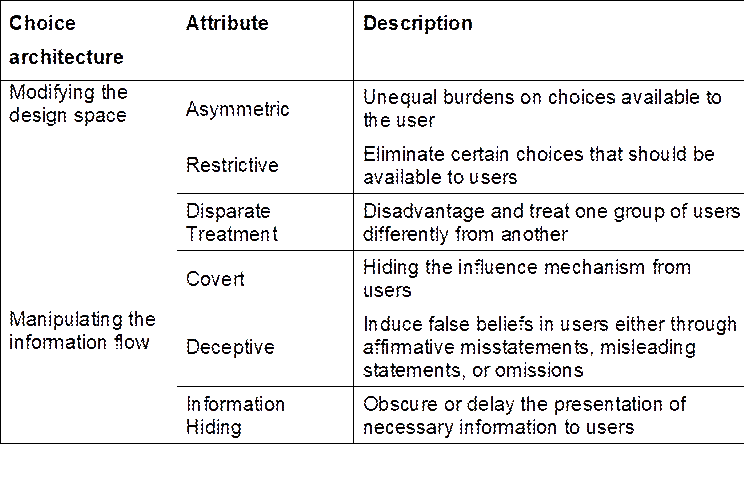
Source 2021
The grouping by Mathur et al. and other research on dark patterns has missed, is the attribute where the user is manipulated for further engagement with the platform. Overall engagement is one of the most important metrics when evaluating a success of a platform, and several dark pattern techniques have been applied to increase it. These design choices are often done by manipulating the information flow, and they attempt to increase the total engagement by appealing to basic human emotions such as happiness, disgust, and anger. The reason why dark patterns are so effective because they make a user’s behavior and actions feel organic and appear like an exercise of their free will.
Facebook, News Feed and engagement
In the case of Facebook, this manipulation of information flow is often evident in the News Feed. In the early days of Facebook, the site was billed as an “online directory” of sorts - each user would have their own page with information about their education, hobbies, relationships, etc. The first version of the Feed was implemented in 2007. This Feed view showed mostly status updates by your contacts and not much else. In 2011 Facebook released the Timeline that moved away from directory structure and into more dynamic way of presenting information (Albanesius, 2014). At some point the official name of the Feed was changed to News Feed, and it became the central part of the platform. It became the first thing that the users saw when they entered the website or used the Facebook mobile app. Farhad Manjoo, a New York Times journalist, stated that for many users, “Facebook is the Feed and the Feed is Facebook”. The main function of the feed is to gather information from various pages and profiles automatically and show this information to its users. This algorithm provided the users with information that would be otherwise too overwhelming to find and at the same time created a convenient and personalized News Feed for each individual user.
What also changed, was that Facebook no longer presented information chronologically, and since 2009 Facebook has used algorithms to organize and decide what is shown to the user and what is not (see Figure 1). This change was explained by an analyst Benedict Evans: “If you have 1500 or 3000 items a day, then the chronological feed is actually just the items you can be bothered to scroll through before giving up.” (Evans, 2018) But for Facebook inc., there was also another reason for this shift: engagement. Now the algorithm was prioritizing content with higher engagement score, thus attempting to engage the user for longer periods of time.
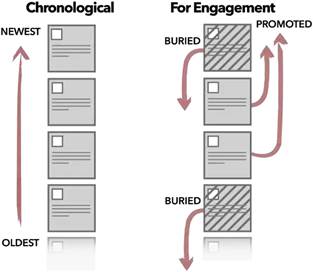
Figure 1 Facebook has replaced chronological ordering of content to an algorithm-based, dynamic model that focuses on engaging its users (Rose-Stockwell, 2018).
Even though the change may have increased the total engagement, it also brought all kinds of problems. The researchers at Facebook found out in 2018 that the algorithm was feeding the users with divisive content that provoked strong reactions to gain the user’s attention and increase their time spent on the platform. (Horwitz & Seetharaman, 2020) This research was shelved by the Facebook inc. executives. This is only one example of how Facebook inc.’s own internal research and executive-level decision-making have been in conflict. More of these examples are introduced in the later chapters.
For the recommendation algorithm to work, it needs some type of information about the user. Previous research and reports have shown that Facebook collects vast amounts of data from its users, both while using the platform and outside of it (Singer, 2018). But it also tracks and analyzes how the user interacts with the content from the News Feed. In 2009, Facebook inc. added the iconic “Like” button on their platform. This was a new way for users to interact with status updates, comments, photos and basically all type of content found on the site. These “likes” could then be shown on the News Feed of your Facebook friends and contacts, enabling people to share their preferences with others.
Additional reaction emojis, “Love”, “Haha”, “Wow”, “Sad”, and “Angry”, were added in 2016. Facebook’s algorithm was then programmed to use these emoji as parameters and signals to push emotion-heavy content to the users. These new emoji were five times more “valuable” than regular “Likes”. (Merrill & Oremus, 2021) The rationale behind this was that content that prompted strong reactions kept users more engaged on the platform. This change led to many unexpected consequences, which will be discussed in the later chapters. In addition to the weighting based on people’s reactions, Facebook has more than 10 000 signals that are used by the algorithm to determine if a content can engage the user. As the News Feed is the core element of the platform, the company has not revealed any information about these signals. (Merrill & Oremus, 2021) This information has so far been only obtained from the leaked internal documents.
Our research argues that in Facebook’s case, dark pattern design increases the overall user engagement (and thus profitability) at the cost of user safety. Next, we discuss the ideas of hate speech, polarization and information bubbles that are closely related to this phenomenon.
Hate Speech, polarization, and information bubbles
As the data online has increased dramatically, also the amount of hate speech online is on the rise (Pacheco & Melhuish, 2020). Detection and removal of online hate speech can be broadly divided into two approaches: a technical, algorithm-based, and non-technical, human-based. The first one attempts to tackle this problem by developing models and algorithms that can identify and remove any content that is considered problematic. Large sums of money have been invested in training these models to be efficient, and for example Facebook inc. has spent 13 billion dollars on “safety and security” on their platform. (Mackintosh, 2021) These models have also been a topic of research in academia (e.g. Vidgen & Derczynski, 2020; Corazza et al., 2020; Salminen et al., 2020). This process is often iterative and iterations are commonly based on various large data sets (Vidgen & Derczynski, 2020). This arms race between the toxic communicators and self-teaching algorithms is still ongoing, but at the moment it seems that the virulent humans have an upper hand. It is commonly understood that the lack of understanding of basic human emotions makes it difficult for algorithms to detect and remove hate speech efficiently, and machines have hard time understanding for example racial histories and power dynamics (Kallioniemi, 2021).
The second approach involves the use of humans as content moderators, and it emphasizes that the problem is only solvable by other humans. The defenders of human curation and moderation claim that algorithms will always have trouble in identifying the different contexts and complexities of human language (let alone multiple languages). But this approach has its problems, too. One of them is bias - for example on Reddit, users are heavily focused on sources that reflect their own political leanings (Soliman et al., 2019). Second problem is related to the heavy toll this type of work can have on one’s mental health. Reviewing content that potentially contains hate speech, vitriol and/or graphic images will most probably be harmful long-term, especially when this type of reviews are conducted on day-to-day basis. In addition to the harmful content, the reviewers are also often under pressure because of demanding performance targets and deadlines (Newton, 2019).
Recent U.N. report stressed that “Online hate is no less harmful because it is online” and that “with the speed and reach of its dissemination, can incite grave offline harm and nearly always aims to silence others” (Kaye, 2019). Munn (2019) has speculated that social media platforms form a kind of pipeline for radicalization with the content that they offer. These types of content often aim to invoke strong emotional reactions and have a strong moral charge. By commenting on a controversial topic, they aim to establish two opposed camps, thus leading to polarization of populations.
Emotionally charged imagery and headlines capture the user’s focus, and emotional reactions like anger are extremely good at engaging them. Munn (2020) has theorized that sharing this content may be a way to offload these emotions by removing their burden on the individual level. Social media platforms enable the sharing of content with only a few clicks, and these sharing chains often result to what Rose-Stockwell calls “outrage cascades” or “viral explosions of moral judgment and disgust” (Rose-Stockwell, 2018). Crockett concluded the phenomenon as follows: “When outrage expression moves online it becomes more readily available, requires less effort, and is reinforced on a schedule that maximizes the likelihood of future outrage expression in ways that might divorce the feeling of outrage from its behavioral expression.” (Crockett, 2017)
3. Methodology
This study adopts a design-centric approach, and it seeks to understand and find a connection between Facebook’s engagement-driven design choices and their consequences in form of prioritizing toxic content, including hate speech, disinformation, and fake news. Design choices, especially on prominent and popular platforms shape our behavior in digital space, and these platforms are thoroughly planned, evaluated, and developed with particular intentions in mind. Thus, a platform can be considered as a set of “core design problems” (Tura et al., 2018, Table 1). This method examines Facebook’s interface design and design choices, and this is done by examining the official internal documents also known as the “Facebook Papers”.
Frances Haugen, an ex-employee of Facebook leaked these documents to the United States congress, and the redacted versions were then reviewed by a consortium of news organizations. The Facebook Papers consisted of presentations, research papers, internal discussions and strategy memos and presented a view into how Facebook executives make decisions for the company. The news organizations that initially reported on the issue were The Wall Street Journal, Protocol, The New York Times, The Washington Post, POLITICO, NBC News, CNBC, CNN, The Verge, Gizmodo, Wired, Associated Press, NPR, The Financial Times, Bloomberg, The Atlantic and The Reuters. In the first phase, all Facebook Papers related material published by these organizations were read and analyzed. In the second phase, other prominent and related articles coming up with a Google search for “Facebook Papers” were read and analyzed. From this analysis we could organize a summary of the leaks.
This summary was then further analyzed through the context of dark pattern design. A scoping review of the dark pattern design academic literature was conducted by compiling a dataset of papers by searching the ACM Digital Library, arXiv, and Google Scholar for academic work that referenced terms “dark patterns”, “dark pattern design”, “anti-pattern(s)”, “deceptive design pattern(s)”, “FoMo design(s)”, “manipulative design” and “manipulative design pattern(s)”. These keywords were considered to be a representative dataset of related work on the topic. These papers were then filtered, retaining work that discusses dark pattern design in the context of user design, and have been published in an academic journal.
4. Results
In this chapter we analyze different examples design choices on Facebook, and how they have affected the users of the platform but also the internal work culture at Facebook inc. Many of these examples refer to the documents leaked by Frances Haugen.
Polarizing content and Facebook’s News Feed
In 2018, Facebook inc. changed its algorithms deciding what content is prioritized on Facebook’s News Feed. Their goal was to prioritize “meaningful social interactions” (MSI for short) between friends and family. The idea behind MSI was to assign values to “likes”, comments on posts, reshares and other interactions on the platform. The algorithm change was executed after rigorous planning - the company ran surveys on more than 69 000 participants in five different countries, asking them about their preferred content on the platform. These findings were then used for “fine tuning” the recommendation algorithm.
In November 2018 an internal research document titled “Does Facebook reward outrage? Posts that generate negative comments get more clicks” concluded that the number of negative comments on a link resulted in more clicks on said it. The document stated that “Ethical issues aside, empirically, the current set of financial incentives our algorithms create does not appear to be aligned with our mission." (Metz, 2021) The change in recommendation algorithm affected publishers, political parties, and individual users alike. For example, in Poland one political party’s social media team made an estimate that MSI resulted in 80% of negative comments on each post. In Spain, many political parties were worried how this change would affect democracy in long-term. There were also personal anecdotes on how this switch to negativity has affected people’s friends and family (Metz, 2021).
Facebook vice president of engineering, Lars Backstrom, defended their algorithm by saying that “like any optimization, there’s going to be some ways that it gets exploited or taken advantage of. That’s why we have an integrity team that is trying to track those down and figure out how to mitigate them as efficiently as possible.” (Keach & Horwitz, 2021) Facebook inc.’s integrity team suggested several changes to the recommendation algorithm that could potentially reduce the rewarding of outrage and lies, but many of them were resisted by Mark Zuckerberg because they might decrease the overall engagement on the platform.
Hate speech and disinformation on Facebook
Based on the Facebook Papers, the company has real trouble in moderating hate speech and harmful content. Facebook cut the number of human curators focusing on filtering out hate speech on the platform (Seetharaman, Deepa Horwitz & Scheck, 2021). The company then decided to use AI and algorithms to identify and censor this type of content, but this turned out to be less than ideal solution. The AI struggled to identify content such as first-person shooting videos and racist rants. It even had trouble in identifying a difference between cockfights and car crashes. The documents revealed the frustration of Facebook’s engineers, and one senior worker claimed that “The problem is that we do not and possibly never will have a model that captures even a majority of integrity harms, particularly in sensitive areas.” (Seetharaman, Deepa Horwitz & Scheck, 2021). Another Facebook team concluded that the company’s algorithms managed to remove 3 to 5 percent of hate speech from the platform, and 0.6 percent of content that violated the company’s own policies against violence and incitement. In 2020, Facebook’s chief technology officer claimed that Facebook’s AI successfully detects 97 % of the hate speech that is on the platform. (Schroepfer, 2021)
Facebook’s VP of Integrity, Guy Rosen has stated that instead of content removal, the more important factor is prevalence. He stated in an interview that “Prevalence is the most important metric, and it represents not what we caught, but what we missed, and what people saw.” (Rosen, 2018) This was also emphasized in a blog post by Rosen (Rosen, 2021). There were two recent examples where these statements where not evident: First example comes from Facebook’s internal research conducted in India. In February 2019, the company set up a test account to test how their recommendation algorithm’s function and what type of content do they offer to the users (Rai, 2021). This test user followed only pages or groups recommended by the Facebook algorithm or that were encountered through those recommendations. In just three weeks, the test profile’s feed was filled with graphic, violent imagery, and fake news. In the internal report, one of the employees wrote that “I’ve seen more images of dead people in the past 3 weeks than I’ve seen in my entire life total.” (Rai, 2021)
The second example comes from Instagram, another platform of Facebook inc. In order to examine how Instagram can potentially affect the mental health of teenagers, U.S. Senator Richard Blumenthal together with people from his office set up a fake Instagram profile to pose as a 13-year-old girl. They used this account to follow some easily findable accounts that were associated with extreme dieting and eating disorders. "Within a day its recommendations were exclusively filled with accounts that promotes self-injury and eating disorders. That is the perfect storm that Instagram has fostered and created.", said Blumenthal in a senate hearing. (US Senate, 2021) As one data scientist at Facebook stated about removing hate speech: "We might just be the very best in the world at it, but the best in the world isn't good enough to find a fraction of it." (Seetharaman, Deepa Horwitz & Scheck, 2021) Facebook inc. has also used a whitelist that renders people immune from censorship algorithms. This list mostly consists of high-profile people, including celebrities, politicians, and journalists. One employee stated in an internal review that “Unlike the rest of our community, these people can violate our standards without any consequences.” (Horwitz, 2021)
Facebook has also had trouble with fake profiles. The number of fake profiles that have been removed from the platform are currently in billions (e.g. Reuters, 2021; Palmer, 2019). In addition to users, Facebook also has a huge problem with disinformation and misinformation - MIT Technology Review concluded that as of 2019, many popular Facebook pages were moderated from Kosovo and Macedonia, known “bad actors” in the 2016 US election. These so called “troll farms” reached 140 million Facebook users from US monthly and 360 million users globally per week. These included the largest Christian American page and largest African American page on Facebook. In October 2019, 15 out of 15 of the biggest Christian American Facebook pages were being run by troll farms. Based on the report, Facebook inc. has conducted several studies that disinformation and misinformation and increase in user engagement are closely related, but the company is still prioritizing this type of content in the user’s News Feed. (Hao, 2021b)
As the documents show, Facebook and their algorithms also has difficulties in filtering out hate speech and disinformation in non-English speaking countries, and especially in developing countries. This issue will be discussed in the following chapter.
Facebook in developing countries
Facebook inc. has faced many problems in developing countries, mainly due to lack of both employees and the lack of training data for algorithms. For example, Facebook’s algorithms struggle with basic Arabic language, and has tremendous trouble with various Arabic dialects. (Seetharaman, Deepa Horwitz & Scheck, 2021) One of the company’s engineers claimed that “As it stands, they have barely enough content to train and maintain the Arabic classifier currently-let alone breakdowns”. (Seetharaman, Deepa Horwitz & Scheck, 2021) In Afghanistan, Facebook inc. took action against 0.23% of the hate speech posts, mainly due to incomplete list of slurs spoken in Afghanistan.
A similar incident was evident during the regional elections in Assam, India. Assam has a large problem with violence against Muslims and other ethnic groups, and these actions are often incited on Facebook. Yet, Facebook inc. did not have an Assamese hate-speech classifiers, and out of the 22 official languages in India, only four are covered by the company’s algorithms. (Perrigo, 2019) Around 25% of India’s population does not speak at least one of these languages (or English) at all. This problem is augmented by the fact that India is one of Facebook’s fastest growing and most important overseas market (Rai, 2021).
In 2019 Facebook inc. set up an Indian test account to see how their own algorithms work on this important market segment. In 46-page research note one of the staffers involved with the test wrote that “I’ve seen more images of dead people in the past 3 weeks than I’ve seen in my entire life total”. The test was designed to focus solely on the recommendation algorithm and the News Feed, and in just three weeks the Feed was filled with anti-Pakistan hate speech, images of beheadings, nationalist messages, and fake and doctored photos. Again, the reason for this grim result lies in the lack of classifiers and training data. Most of the money Facebook inc. spends on moderation is focused on English-language content, even though the company’s largest growth comes from countries like India and Brazil. (Rai, 2021)
Internal documents also show that Facebook inc. struggled with civil war ridden Ethiopia (see Figure 2). Their internal ranking system ranked the country at the highest priority tier for countries that are in risk of conflict, but that the company did not have sufficient resources to curb the Ethiopia-related hate speech on Facebook (Mackintosh, 2021). The platform was actively used by militia groups such as the Fano for calls of violence against ethnic minorities. A leaked document showed that Facebook had difficulties in building algorithms to detect misinformation, disinformation and hate speech in Oromo or Amharic, which are the two most spoken languages in Ethiopia.
But before all this, Facebook inc. was accused of being complicit on the persecution of Rohingya Muslims in Myanmar during 2016 and 2017. Today, Myanmar stands accused at the International Court of Justice (ICJ) for committing a genocide on the Rohingya ( Justice, 2021 ). Facebook inc. was requested for “documents and communications from Myanmar military officials” and other information that was taken down but conserved by the social media giant. Facebook inc. rejected this request, claiming that it was “extraordinarily broad” and that it would "special and unbounded access" to private accounts (McPherson, 2020). This was in direct conflict with the company’s Human Rights Impact Assessment, where they stated that the company should “preserve and share data where it can be used to evaluate international human rights violations, and that the company publish data specific to Myanmar so that the local and international community can evaluate progress more effectively.” (Choudhury, 2020)
Facebook uses hate speech detection algorithms for 40 languages worldwide. In the rest of the world, Facebook relies on user reports and human moderators to police and remove hate speech. Human moderators do not regularly scan the site for harmful and restricted content but make the final decision if the reported content should be removed. Avaaz, a global advocacy group, reported that with clearest examples of Assamese hate speech on Facebook, the removal took anything from hours up to three months. Some of this hate speech remained on the platform. (Perrigo, 2019)
Choudhury (Choudhury, 2020) has speculated that Facebook inc. sides with oppressing regimes and governments to protect their business interests in these domestic markets which they dominate by a wide margin. For example, for many people in Myanmar, Facebook is the internet. Any kind of bans could bring in state regulations that would then affect the company’s profits. These policies could also affect the opinion of the general public.
5. Discussion
RQ1: What type of dark pattern designs Facebook inc. has applied to their site’s functionalities and what are their implications?
The Facebook Papers showed that Facebook has had trouble dealing with growth, disinformation, and moderation. Facebook has replaced many of its employees and curators with algorithms that filter content based on pre-defined identifiers. Based on their internal documents, these algorithms do not work as intended, and large numbers of hate speech prevails on the platform. Developing and changing algorithms on a scale as big as Facebook can have drastic consequences on individuals and even on countries. These consequences can become even more dramatic if there are no skilled human factors involved - this was evident in countries like Afghanistan, Ethiopia, and India (Frenkel et al., 2018; Mackintosh, 2021; Perrigo, 2019). Based on Kallioniemi (2021), algorithms have real challenges in understanding basic human emotions such as happiness, anger and sadness. Yet on Facebook the promotion of engaging content is almost solely based on these factors and shocking, controversial, and emotion-evoking content often rises to the top. Algorithm changes such as MSI have been criticized inside the company, too, yet the focus of the recommendation algorithm is still mostly on maintaining and increasing user engagement. A lot of the criticism has also been written off as an “optimization issue”. The problem with this type of thinking is that this optimization is happening on a live site with billions of users, and a lot of the information sharing is done by fake users and pages (Hao, 2021b; Palmer, 2019).
Mathur et al. (Mathur et al., 2021) suggested a collection of higher-level dark pattern attributes, and we suggest a new sub-attribute to this collection (Table 2). Information Promotion refers to the promotion of engaging content regardless of the validity or safety of the information it contains. This attribute is evident in Facebook’s recommendation algorithm, and it is driving the user engagement on the platform.
Table 2 Higher-level dark pattern attributes grouped based on how they modify the user’s choice architecture, with the added category of Information Promotion.
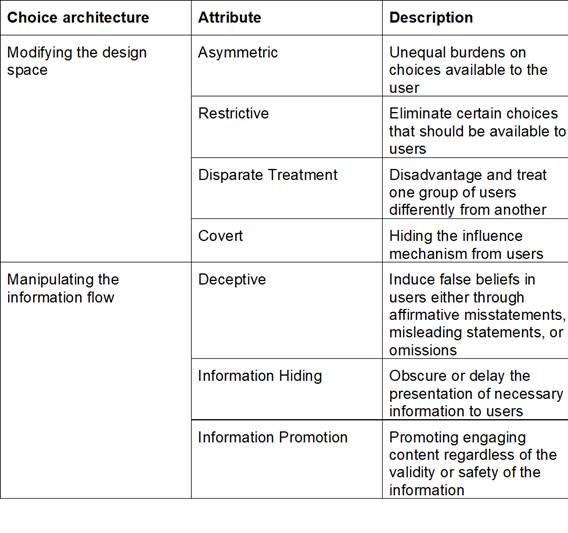
Source(Mathur et al., 2021)
To summarize, these are the following dark patterns that are used by Facebook Inc.:
RQ2: How does Facebook’s public relations (PR) contradict with their internal work culture and user interface design?
Many of the leaked documents show that Facebook inc. employees have repeatedly sounded the alarm on the company’s failure to act on important matters such as issues with the recommendation algorithm (Merrill & Oremus, 2021; Rai, 2021), spread of hate speech and fake news (Hao, 2021b; Munn, 2020; Palmer, 2019) and incite for violence (Choudhury, 2020; Mackintosh, 2021). The divisive nature of the recommendation algorithm was found out already in 2018, when Facebook inc.’s internal research found out and reported that the recommended content provoked strong reactions but also increased the time they spent on the platform (Horwitz & Seetharaman, 2020). One former Facebook AI researcher said that “study after study” confirmed that models that maximized engagement also increased polarization (Hao, 2021a). On many occasions (e.g. Keach & Horwitz, 2021; Wade, 2021) Facebook inc.’s executives have decided to act against the company’s internal reports, which may have also been the cause for many employees leaving the company (Hays, 2021). Internal memo has also shown that Facebook inc. has difficulties in hiring new engineers (Kramer, 2021). These problems are far from unique, but the recent scandals may have increased this problem in Facebook inc.’s case even further. Facebook also lost users for the first time in the social media platform’s history and at the same time had its biggest single-day loss yet (Dwoskin et al., 2022). Jorge et al. (2022) analyzed “digital well-being” tools that were rolled out by many tech companies after facing critique on the negative effect their platforms had on people. Facebook’s tool, called Your Time, quantified time spent on the company’s platforms and the goal was to help those who struggle with online addiction. But the problem is often not the time people spend on these platforms, but the type of content they consume.
Haugen is not the only ex-employee that has criticized Facebook and social media in general. Back in 2017, a former Vice President for User Growth of Facebook, stated that
“[t]he short-term, dopamine-driven feedback loops that we have created are destroying how society works: no civil discourse, no collaboration, misinformation, mistruth and it's not an American problem. This is not about Russian ads. This is a global problem. It is eroding the core foundations of how people behave by and between each other.” (Wong, 2017)
Other prominent ex-employees that have come out and criticized the company include Sean Parker (founding president), Roger McNamee (investor), Justin Rosenstein (engineer), Leah Pearlman (product manager), Yaël Eisenstat (head of “Global Elections Integrity Ops”), and Sandy Parakilas (operations manager). Their criticism has been part of the movement which has changed the Silicon Valley “techno-utopianism” into Silicon Valley dystopianism. For more extensive analysis on this subject, see Karppi & Nieborg (2021).
These recent leaks and the public discussion revolving around them have also caused many corporations to take measures to prevent events like this from happening again. For example, Microsoft is applying spyware, AI and machine learning for preventing its employees from leaking sensitive documents (Matyszczyk, 2021) and Facebook inc. has made their internal platform safety and election protection related message boards private instead of public, thus limiting the participants for open discussion. (Mac, 2021) It seems, that instead of creating a more open work culture, these leaks have caused the companies to close up and spy on their own employees even more.