Serviços Personalizados
Journal
Artigo
Indicadores
-
Citado por SciELO
-
Acessos
Links relacionados
-
Similares em SciELO
Compartilhar
Ciência e Técnica Vitivinícola
versão impressa ISSN 0254-0223
Ciência Téc. Vitiv. v.25 n.2 Dois Portos 2010
Predicting probability to purchase insurance contracts in the Chilean wine industry: a logit and probit comparative analysis
Germán Lobos1, Jean-Laurent Viviani2, Berta Schnettler3, Natalia Muñoz4, Ángela Reyes4
1Ph.D. Universidad de Talca, Facultad de Ciencias Empresariales (FACE), Escuela de Ingeniería Comercial, 2 Norte 685, 3465548 Talca, Chile. Tel: 56 71 200330; globos@utalca.cl
2Ph.D. Université Montpellier 1 Institut des Sciences de LEntreprise et du Management (ISEM), Centre de Recherche sur le Management et les Marchés (CR2M), Espace Richter - Bât. B - Rue Vendémiaire - CS 19519 - 34960 Montpellier cedex 2, France. Tel: 33 499 130245; jviviani@univ-montp1.fr
3Ph.D. Universidad de La Frontera, Facultad de Ciencias Agropecuarias y Forestales, Departamento de Producción Agropecuaria, Avenida Francisco Salazar 01145, 4780000 Temuco, Chile. Tel: 56 45 325655; bschnett@ufro.cl
4Ingeniero Comercial. Universidad de Talca, Facultad de Ciencias Empresariales (FACE), Escuela de Ingeniería Comercial; namunoz@utalca.cl, areyes.ic@gmail.com
SUMMARY
In the less developed countries the use of market instruments for risk management is still limited. The main purpose of this study is to identify the variables that have an infl uence on the probability of using insurance contracts in the wine industry of Chile. Binomial logit and probit models were applied using a sample of 84 fi rms. Our results indicate that the probability of purchasing insurance depend on wine market access and insurance contracts risk management (p<0.01), total surface planted with vineyards, wine prices access, sanitary risk and expected hedging insurance contracts (p<0.05). The global correct prediction was estimated (83.33%) using Receiver Operating Characteristic (ROC) curve analysis. One important conclusion is that viticulturists are more willing to buy insurance contracts if they perceive that these will allow an adequate management of risk.
Additional key words: binomial model, wine market access, risk management, ROC curve
Probabilidade de predição para comprar contratos de seguro na indústria de vinho Chilena: uma análise comparativa logit e probit
RESUMO
Nos países menos desenvolvidos o uso de instrumentos de mercado para a gestão de risco é ainda escasso. O objectivo principal desta investigação é identificar as variáveis que influenciam sobre a probabilidade de utilização de contratos de seguros na indústria de vinho chilena. Aplicaram-se modelos logit e probit usando uma amostra de 84 empresas. Os nossos resultados indicam que a probabilidade de adquirir seguros depende do acesso a mercados para o vinho (p<0,01), da superfície total plantada com vinhedos, acesso a preços do vinho, riscos sanitários e a cobertura esperada dos contratos de seguros (p<0,05). A predição global do modelo (83,33%) foi estimada usando a análise da curva Receiver Operating Characteristic (ROC). Uma importante conclusão é a de que os viticultores estão dispostos a contratar seguros se perceberem que tais contratos permitem gerir adequadamente o risco.
Palavras-chave: modelo binomial, acessos a mercados para o vinho, gestão de risco, curva ROC.
INTRODUCTION
In the last years the discussion about the availability of market instruments for risk management in agriculture has intensified. One central aspect of the discussion is the design of financial coverage instruments for the management of risk reduction, which is expressed basically in the high volatility of the principal risks that agricultural producers face. A recent study by Lobos and Viviani (2010) indicates that for Chilean viticulturists the main risks sources are the exchange rate, wine price, climatic changes, yields and sanitary risks. Even though the Chilean market of risk management instruments is still incipient, there exist some instruments available, as agricultural insurance policy and forward contracts (interest rates and exchange rate). This limited development is made worse by the fact that this is a sector in which there exist information asymmetries (i.e., moral hazard and adverse selection).
One relevant characteristic in the provision of goods and public services is the existence of incomplete markets, which has negative effects on the economic efficiency. In the agricultural private markets it is possible to fi nd this market failure, in terms of the relative shortage of instruments to face risk and uncertainty. According to Skees and Barnett (1999), as the level of covered risks is clearly less than the socially optimal amount, there is necessarily a loss of efficiency in the allocation of resources.
The limited development of the main financial coverage instruments, such as insurance contracts, loans, futures and derivatives, is usually used as an argument to justify a greater intervention of the government in agriculture. According to Meuwissen et al. (1999), as the government has an influence on the farmers decisions through the legal frame, it becomes a co-responsible for the potential losses, so justifying its participation with public instruments of risk reduction or through aids when the risk has more systemic characteristics.
Different authors (Hardaker et al., 2004; Meuwissen et al., 1999; Just and Pope, 2002) suggest that the types of insurances usually available in agriculture are insurances on expected yields, insurances on prices, revenue insurance, net income insurance, insurances against catastrophic losses and basic coverage against income crisis. In general, for the correlated agricultural risks are more adequate the latest instruments for the management of risk, such as forward, futures and derivatives (Carpenter, 2005), while on the other hand, for the less correlated risks the more traditional instruments are applicable (Meuwissen et al., 1999).
The bivariate and multivariate analysis techniques, such us logit, probit and tobit (Tobin, 1958), are widely used in diverse disciplines of scientifi c research such as psychology, economics and finance, politics, law, environment, biology, biotechnology, agriculture, chemistry, health, software engineering and education. In finances the literature is concentrated in predicting the probability of business failure in different financial markets (Johnsen and Melicher, 1994; Lennox, 1999; Westgaard and Wijst, 2001; Grunert et al., 2005; Bandyopadhyay, 2006; Chi and Tang, 2006). In some more specific sectors the logit, probit and/or tobit models have been used to predict the probability of getting health insurance or life insurance (Hopkins and Kidd, 1996; Sapelli and Torche, 2001; Barrett and Conlon, 2003), to predict the buying decisions in a market (Goldberg, 1995; Banerjee, 2004) or to predict the probability of success of a new product (Kandemir et al., 2006), to predict the selection of urban transportation means (Amador et al., 2005), to assess the impact of human and fi nancial capital on small businesses profi tability (Coleman, 2007), and to predict decisions related to the labor market (Aituvh and Kimhi, 2006; Benjamin and Kimhi, 2006).
The studies related with the probability of getting health insurance or life insurance (Nielsen and Mayer, 2000; Tan et al., 2009) suggest that the income of the person or households is statistically significant and influences positively on the probability of getting these insurances. In other words, higher-income consumers were more likely to purchase life insurance policy. In the case of acquisition of non-life insurers Meador et al. (1986) concluded that the variable having the most significant relationship to the probability of acquisition in the negative value of the return on total investment.
Relatively little research has been conducted that specifically investigates the demand for crop insurance in the context of a revenue insurance program (Mishra and Goodwin, 2003; Goodwin et al., 2004; Sherrick et al., 2004; Shaik et al., 2008). The literature review shows that the demand for crop insurance is inelastic (Goodwin et al., 2004), but the elasticity for choices between yield and revenue insurance is found to be relatively more elastic (Shaik et al., 2008).
In the case of decision-making in agriculture in their works of Shaik et al. (2008) indicates that the variables that influence more significantly the probability of acquiring of insurance are the expected return to insurance (sign is positive), average expected price (sign is negative), acreage under irrigation (sign is positive). In other studies it is suggested that the statistically signifi cant variables are the producers experience and the relative production of the farmer versus the production of the whole industry (Huber, 2005), the amount of farm-related debt, education level, and a history of receiving emergency disaster relief payments (Smith and Baquet, 1996), upon expected premiums to insurance and the variance of returns (Knox and Richards, 1999). The studies suggested that producers risk aversion is not statistically significant (Chambers and Quiggen, 2002; Shaik et al., 2008).
More specifically in a case of the wine industry econometric applications have been developed to detect problems of information symmetry in the prices of fi ne wines (Thode et al., 2002), analysis of the placebo effect of wine (Priilaid, 2006), and models to explain the hedonic prices of Vitis finifera and wine based on certain attributes of grapes (Golan and Shalit, 1993) or of the wine (Angulo et al., 2000; van Rensburg, 2004; Priilaid and van Rensburg, 2006; Troncoso and Aguirre, 2006). The models of discrete selection applied to the wine industry are basically circumscribed to the preferences and wine buying decisions of consumers (Morey et al., 2002; Skuras and Vakrou, 2002; Ho and Gallagher, 2005; Rodríguez et al., 2009). Finally, Lobos and Viviani (2008) developed a model of insurance contract in the Chilean wine industry, but restricted to the logit modeling for a sample of 104 enterprises.
This investigation is an extension of the previous work and its contribution lies in the comparison of discrete selection models (logit and probit) applied to the Chilean wine industry and the willingness of the producers to get instruments (insurance) of financial coverage. The methodology used consist in relating binary categorical variables considering one in particular as dependent on the others, through regression functions of its logarithms (McFadden, 1974; Altman et al., 1981; Jobson, 1992). The main purpose of this research is to identify the variables that have an influence on the probability of using insurance contracts in the wine industry of Chile. Binomial logit and probit models were applied using a sample of 84 firms.
MATERIAL AND METHODS
The sample
The data used in this study were compiled between January and October of 2007 through the application of a questionnaire on the sources of risk in the Chilean wine industry (for details, see Lobos, 2009). The questionnaire has seven sections and it was applied to enterprises located in most of the wine producing valleys of Chile. Each measurable variable was captured through the indicators, which were measured using Likert type scales of five and seven points. In other cases open and closed questions were used (dichotomic and multiple options), and interval and nominal scales.
Section 1 refers to the commercial activity of the enterprise, the number of permanent workers, the perception of the wine grower concerning debt level, the negotiating conditions of the wine selling contracts, the access to price information in the domestic and international markets, and the ease of access to wine buyers. Section 2 helps to have an approximation of the degree of aversion to risk of the wine producers and of the importance they assign to the different types of risk they face and on the perception concerning the impact on the enterprise of the risks associated to the variability of wine prices and climatic changes.
The information about the new financial instruments for risk management as well as the knowledge and use of these instruments are presented in section III. Section IV captures information about the degree of use of insurance, the opinion of the wine producers about the adequacy of insurance as instruments for the management of risk and the expectations regarding the ideal characteristics of these instruments. Section V captures information about public instruments for risk management, the degree of knowledge and of use of said instruments, but impact on the financial situation of the enterprise, as well as of the desirable characteristics of the new instruments. The personal profile of the wine growers is viewed in section VI. The age, participation in the companys capital, investment in the stock exchange, sources for the acquisition of consultancy as modifying factors of attitude in face of the risk for winegrowers.
In this study a sample of 84 enterprises was used, somehow less than the one used in the works of Lobos and Viviani (2008) and Lobos (2009). The data was processed using SPSS v 15.0.
Dependent and explanatory variables
With the objective of contrasting the influence of the principal explanatory variables over the decision of using insurance contracts, the models logit and binomial nominal probit were considered, in which the use of insurance contracts was introduced as dependent variable, and various socioeconomic variables and sources of risk were introduced as explanatory variables (see Table I).
TABLE I
Definition of the dependent and independent variables in the logit and probit models
Definição das variáveis dependentes e independentes nos modelos logit e probit
Establishing the mathematical models
All binary choice models, except the linear probability model, are usually estimated by the method of maximum likelihood (Greene, 1999; Wooldridge, 2006). The maximum likelihood method was proposed initially by Fisher (1922) and it is a classical estimation method of the parameters associated with density functions or random variables probability. Every observed indicator variable i y is considered as the individual realization of a binomial process which probabilities vary from one test to the other depending on each vector of independent variables (Maddala, 1996). A Bernoulli trial is a probabilistic experiment that can have one of two outcomes, success (x=1) or failure (x=0) and in which the probability of success is p (Evans et al., 2000). We refer to p as the Bernoulli probability parameter.
Let yi be a random variable with probability function f ( y;β ) , where β is an unknown parameter. Let Y1 = y1, Y2 = y2, ., Yn = yn be the observed values in a random sample of size n. Given the supposition of random sample, the joint distribution of y1,y2,..., yn is simply the product of the individual density functions . The likelihood function is defined as the joint density function of the random sample:
The likelihood function is a random variable, since it depends on the result of the random sampling y1, y2,..., yn and on the unknown parameter β . The maximum likelihood estimator (MLE) of βis the value of β that maximizes the likelihood function L (Y i; β) . Usually, it is more convenient to maximize the logarithm of the likelihood function (log-likelihood function):
Our interest is focused on the distribution of Y conditioned on a vector of explanatory variables x, lets say x1, x2 ,..., xk . The density function f (Yi; β) can be written as which depends on k unknown parameters β1, β2 ,..., βk that are necessary to estimate. Replacing in the expression (2):
To obtain the maximum likelihood estimators , the k partial derivatives of the likelihood function L (Yi; β ) must be made equal to zero and solve the resulting system, as indicated:
In the particular case of a binary choice model, we are interested in the response probability:
In expression (5) x is a vector of explanatory variables and β' is a vector of parameters that include the intercept. The function G takes only values between zero and one 0 < G (z) < 1, where z is a real number obtained from . In the logit model, the function G is the cumulative distribution function (CDF) of the standard logistics, expressed as:
In the probit model, the function G is the cumulative distribution function (CDF) of a normal random variable, expressed as an integral:

The logit and probit models can stem from a latent variable underlying model (Wooldridge, 2006). Let y *be a non observed variable, or latent variable, determined by:
The indicator function l(.) is a binary variable of results, where y takes the value one when y* > 0, and zero when y* ≤0 . It is assumed that e is independent of x and that it follows a standard logistic distribution (logit model) or normal typified distribution (probit model). In addition, it is assumed that e is distributed symmetrically around zero, which implies that 1-G(-z) = G(z) for every real value of z.
The response probability of y is obtained as:
Note that this previous expression is coincident with (6) and (7) of the standard logistics and the normal typified logistics, respectively.
Goodness-of-Fit Tests
To evaluate the goodness-of-fi t of the models, different statistical tests were used. McFaddens R2 is also known as the likelihood-ratio index. It compares the likelihood for the intercept only model to the likelihood for the model with the predictors (McFadden, 1973). In the discreet selection models, the McFaddens R2 does not have a direct interpretation, and it is considered that the best model is the one that presents a greater statistical value.
Akaikes Information Criterion (AIC) is not a test of the model in the sense of hypothesis testing; rather it is a tool for model selection or test between models (Akaike, 1974). Given a data set, several competing models may be ranked according to their AIC, with the one having the lowest AIC being the best. When estimating model parameters using maximum likelihood estimation, it is possible to increase the likelihood by adding parameters, which may result in overfitting . The Bayesian Information Criterion (BIC), or Schwarz Criterion, resolves this problem by introducing a penalty term for the number of parameters in the model. Given any two estimated models, the model with the lower value of BIC is the one to be preferred (Schwarz, 1978). The Hannan-Quinn Criterion (HQC) is an alternative to AIC and BIC criterions. However HQC statistic usually punishes a model with more parameters more than the AIC, but not as severely as the BIC (Hannan and Quinn, 1979).
Similarly, goodness-of fit evaluation of the models can be done by means of Likelihood Ratio statistics (LR) Omnibus tests is a test of the null hypothesis that adding the gender variable to the model has not significantly increased our ability to predict the decisions made by our subjects. The -2LL (-2 Logarithm of the Likelihood) measures how poorly the model predicts the decisions the smaller the statistic the better the model. Under the null hypothesis that the model fi ts perfectly, -2LL has a chi-square distribution, with (N-p) degrees of freedom, where N is the number of cases and p is the number of estimated parameters (Hosmer y Lemeshow, 1989).
On the other hand, Hosmer and Lemeshow (1980) proposed a Pearson a chi-square statistic based on a grouping of the estimated probabilities. The R2 Cox & Snell can be interpreted like R2 in a multiple regression, but cannot reach a maximum value of 1. The adjusted Nagelkerke (1991) R2 can reach a maximum of 1.
Finally, it is possible to evaluate the predictive power of the logit and probit models using Receiver Operating Characteristic (ROC) curve analysis (Lloyd, 1998, 1999; Cai and Pepe, 2002; Zhou and Castelluccio, 2003). The ROC curve represents, on a coordinate system, the sensitivity and the specificity for different cut-points. he sensitivity is the proportion of cases classified as 1 and that in effect are 1 and specificity is the proportion of cases classified as 0 and that in effect are not 0 for cut-point 0.5. The area under the ROC curve can then be interpreted as the probability that in the presence of a couple of observed cases like 1 and 0 the test would classify them correctly (Hanley and McNeil, 1982).
General characteristics of the companies in the sample
It is worth noting that the formal Chilean wine industry is composed of 230 enterprises with very homogeneous characteristics among them in terms of vine planted surfaces, volume and value of sales, number of permanent workers, inversion in advertising, market power in the domestic wine and grape market, among others. As a reference, less than 10 companies have more than 1 000 planted hectares, less than 30 companies have more than 300 planted hectares and less than 50 companies have more than 100 planted hectares. In short, the big wineries are relatively few in number, whereas the main group is composed of the intermediate and small size wineries, which compete intensely in the domestic market. On the other hand, if we consider the number of proprieties with wine vines, there are over 14 000 in Chile, of which a little more than 13 600 have less than 50 hectares, that is, around 97%.
The sample includes wineries located in the main wine producing valleys of Chile: Aconcagua (8%), Maipo and Rapel (13%), Curicó (11%) and Maule (54%). The companies included in the sample have a total of 27 885 ha planted with vines, which represent 27% of the total surface with wine grapes for 2008 in Chile. 51 companies showed sales for less than US$ 1 million and 29 declared sales for more than US$ 1 million. 21% of the small wineries have used public programs or instruments of development, while on the other hand 79% of the large wineries have used them (see Table II).
TABLE II
Characteristics of the wine-growing companies included in the sample
Características das empresas vitícolas incluídas na amostra
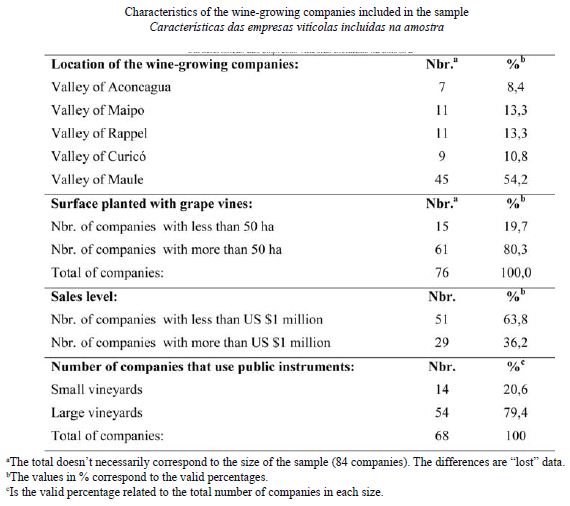
RESULTS
The main purpose of this study is to identify the variables that have an influence on the probability of using insurance contracts in the wine industry of Chile, based on the influence that a set of socioeconomic variables and the winegrowers own perceptions have on the willingness to take insurances. The explanatory variables that finally turned out to be significant are related mainly to the perceptions of the winegrowers: ease of access to prices and wine markets, perception of the sanitary risk, perception of the insurance contracts and expected coverage. Only the socioeconomic variable concerning the surface planted with wine grapes turned out to be significant in the models.
Logit and probit models
The results of the multiple regression models are shown in Table III. Parameters estimated and z-statistics of all the variables are presented. The sign on TSurfaceV is positive and significant at the 5% level. This result suggests that the winegrowers are more willing to take insurance contracts when the surface planted with wine grapes is large (more than 50 ha). The sign on WPricesA is negative and significant at the 5% level. This result suggests that the winegrowers perceive a lesser need to take insurance contracts when they estimate that the access to references of the price of wine is easy. The sign on WMarketA is positive and significant at the 1% level. This result shows that when winegrowers estimate that the access to wine market information is easy, they are more willing to take insurance contracts. The opposite signs of the coefficients of the variables WPricesA and WMarketA show an apparent contradiction. However, the variable WPricesA refers specifically to the access to wine price information and if the winemaker estimates that the access to this type of information is easy, then it is less probable that he will be willing to use, for instance, a wine price insurance. On the other hand, the variable WMarketA refers to the access of market information, which is wider than the price information since it can consider the access to strategic information like, for instance: variables that have an influence on the consumers decision as to which wine to buy, what are the tastes and preferences of the consumers, what are the commercialization mode of wines, what are the competitions commercialization channels, how are the prices determined in times of wine offer and how much are the prices reduced related to the normal price. Then, since the risk and uncertainty associated to market information spans over different sectors, a greater willingness to use insurance can be explained when the winemaker has easier access to market information, because this information is associated with different risk and uncertainty generating sources.
TABLE III
Results of the multiple regression models estimated via Maximum Likelihood (ML) method
Resultados dos modelos de regressão múltipla estimados pelo método Maximum Likelihood (ML)
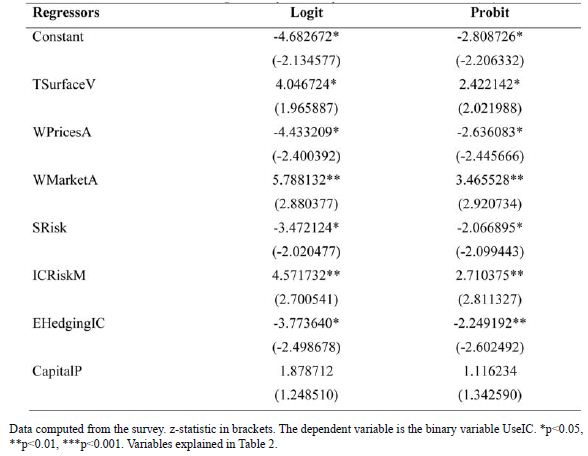
The sign on SRisk is negative and significant at the 5% level. This result shows that when winegrowers perceive that the sanitary risk is a significant source of risk, they are less willing to take insurance contracts.
The sign on ICRiskM is positive and significant at the 1% level. In other words, winegrowers are more willing to take insurance contracts when they estimate that the insurance contracts allow them to manage adequately the risk they face. The sign on EHedgingIC is negative and significant at the 5% level. This result indicates that winegrowers are less willing to take insurance contracts when the expected coverage is high. Finally, the sign on CapitalP is positive and not significant. This result, even though it is not statistically significant, suggests that winegrowers are more willing to take insurance contracts when the winegrowers participation in the companys capital is high (greater than 50%).
Goodness-of-fit evaluation: logit and probit models
The results of the evaluation of the goodness-of-fit statistics of the models suggest that the probit model has a greater degree of efficiency in explaining the willingness to use insurance contracts by the Chilean winegrowers (see Table IV). The pseudo McFaddens R2 (the highest value) and the AIC, BIC, HQC criteria (the lowest values) suggest that the probit model is more suitable than the logit model to explain the willingness to take insurance contracts. The LR and -2LL statistics indicate that the logit and probit estimations are statistically significant to the value p<0.001. The value chi-square calculated is not significant for Pearsons chi-square test and Hosmer-Lemeshow (HL) for the logit and probit models, indicates that the fit of both models is adequate to predict values that are not significantly different from the observed values. The high values in Cox & Snell R2 and adjusted R2 Nagelkerke are associated with a good fit of the logit model.
TABLE IV
Goodness-of-fi t evaluation of the models estimated via Maximum Likelihood (ML) method
Avaliação do ajustamento dos modelos estimados pelo método Maximum Likelihood (ML).
Expectation-Prediction evaluation and ROC curve
The results shown in Table V indicate that the comparison of the forecasted results with the data observed through a classification table implies that the global correct prediction is 83.33% for logit and probit models. On the other hand, the sensitivity (85.71%) is greater than the specificity (81.10%) for the cut-point of 0.50. The area under the ROC curve suggests that there is a probability of 83.10% that considering two companies, one using insurance and the other not, the test w ill classify them correctly. Fig. 1 shows the ROC curve for the logit and probit models estimated.
TABLE V
Expectation-Prediction evaluation for binary specification of the models estimated via Maximum Likelihood (ML) method
Avaliação da espectativa-previsão para especificações binárias estimadas pelo método Maximum Likelihood (ML)
DISCUSSION
The major virtue of the study reported here is that it is the first empirical research of vineyards purchase intentions with respect to risk management insurance. The study is necessarily exploratory, but like most exploratory studies, the results are based on a sub-optimal sample. The sample used here is small (N=84), confined to recognized vineyards, and fairly homogenous in terms of characteristics such as production processes, location and markets for the wine. This study has also shown the usefulness of the discreet selection models (logit and probit) to help identify the fi rms individual characteristics that have an influence on the probability of using insurance contracts as instruments of financial coverage for the management of the principal risks that Chilean winegrowers face. The descriptive analysis allows inferring about the influence that a set of socioeconomic variables and the winegrowers own perceptions have on the willingness to purchase insurances. The estimators of the probit model are related to approximately 0.6 of the logit estimators, close to the proportion of 0.625 that the literature suggest (Amemiya, 1981; Maddala, 1996).
In general, the sign of the coefficients confirm the previous perception about the factors that influence positively about the probability of purchasing insurance. This is there is a greater disposition to use insurance in the case of the larger vineyards, when winegrowers estimate that the insurance contracts allow them to manage adequately the risk and when the winegrowers participation in the companys capital is high. The findings of Shaik et al. (2008) about the positive relationship between the disposition to purchase insurances and the acreage under irrigation seem to be similar to the positive relationship between the willingness to take insurance and the size of the vineyard measured as the surface planted with vines. The significance of the winegrowers participation in the companys capital is consistent with the eventual existence of an Agency Problem, or a conflict of interest arising between shareholders and managements because of differing goals.
Some factors that have a negative influence on the probability of taking insurances also agree with the previous perception. For example, when winegrowers perceive that the access to the price of wine references is easy (i.e., when there exists perfect information in competitive markets for the wine). In an opposite sense, when winegrowers perceive that the access to the wine price references is difficult (i.e. when there is uncertainty about the expected prices) they will be more willing to use insurance, which seems consistent with the work of Shaik et al. (2008) in the sense that farmers perceive a need to purchase insurance when expected prices are low.
The positive relationship between the probability of taking insurance and the size of the plantations, in which the former could be considered as a proxy of the wealth variable (stock), suggest that the vineyards that have a greater economic value will be more ready to take insurances. The same positive relationship can be observed between the willingness to purchase insurance and the winegrowers participation in the ownership of the company, although this last variable was not significant. However, the aforementioned relationships lead in the same direction than the findings of Nielsen and Mayer (2000) and Tan et al. (2009) for the purchase of personal coverage, this is, a positive relation between higher-income consumers (or grater wealth in present value) and purchase of life insurance policy. Unlike the work of Miador et al. (1986) that included as explanatory variable the return on total investment for the case of acquisition of non-life insurers, in the present study were not included the explanatory variables related to the financial situation of each company. It was only tried with the variable sales income (in millions of dollars), which turned out to be non significant.
The variables related to the experience of the producer, the relative production of the company, amount of farm-related debt, education level and the variability of yield, included in the Works of Smith and Baquet (1996) and Huber (2005), were considered in the models, but they turned out to be non significant. The producers risk aversion was included in our estimations, but it was not significant, as reported in the works of Chambers and Quiggen, (2002) and Shaik et al. (2008).
Surprisingly, winegrowers are less willing to take insurance contracts when the expected coverage of the contracts is high, contrary to what the works of Knox and Richards (1999) and Shaik et al. (2008) suggest. However, this apparent contradiction could be explained due to the fact that the coverage and the upon expected premiums to insurance are not aligned with the real needs or expectations of Chilean winegrowers. The current offer of insurances corresponds to standardized instruments that can be taken by any company and are not specific to a particular industry. In fact, Chilean winegrowers would like to find an insurance contracts offer that would have a greater coverage of the agriculture risks (i.e. drought, diseases, pests), with lower premiums, less complex and more accessible (i.e. that they could be taken over the phone, at fairs, at winegrowers fairs and in congresses). However, our results also show that producers are more willing to take insurances if they perceive that these contracts allow for an adequate management of risk.
The results of the goodness-of-fit indicate that in all the evaluated models of regression a good global fit is achieved, with a preference towards the probit model over the logit. Nevertheless, both the indicators of the classification table and the area under the ROC curve allow to deduce that the global predicting power of the logit and probit models is high.
ACKNOWLEDGEMENTS
The authors acknowledge financial support from the Dirección de Investigación (DI) of the Universidad de Talca to the project nº E000382-2009 (Enlace Fondecyt), under the title Modelando la percepción de los riesgos en la industria vitivinícola chilena.
REFERENCES
Aituvh A., Kimhi A., 2006. Simultaneous estimation of work choices and the level of farm activity using panel data. European Review of Agricultural Economics, 33, 49-71.
Akaike H., 1974. A new look at the statistical model identification. IEEE Transactions on Automatic Control, 19, 716-722.
Altman E.I., Avery R.B., Eisenbeis R.A., Sinkey J.F., 1981. Application of Classification Techniques in Business, Banking and Finance. J.A.I. Press, Greenwich CONN, USA.
Amador F.J., González R.M., Ortúzar J.D., 2005. Preference heterogeneity and willingness to pay for travel time savings. Transportation, 32, 627-647.
Amemiya T., 1981. Qualitative response models: a survey. Journal of Economics History, XIX, 1483-1536.
Angulo A.M., Gil J.M., Gracia A., Sánchez M., 2000. Hedonic prices for Spanish red quality wine. British Food Journal, 102, 481-493.
Bandyopadhyay A., 2006. Predicting probability of default of Indian corporate bonds: logistic and Z-score model approaches. The Journal of Risk Finance, 7, 255-272.
Banerjee A., 2004. A brand share prediction model based on several disparate sources of data: an empirical model of detergent choice in Mumbai, India. Asia Pacifi c Journal of Marketing and Logistics, 16, 3-22.
Benjamin C., Kimhi A., 2006. Farm work, off-farm work, and hired farm labour: estimating a discrete-choice model of French farm couples labour decisions. European Review of Agricultural Economics, 33, 149171.
Barrett G.F., Conlon R., 2003. Adverse selection and the decline in private health insurance coverage in Australia: 1989-95. Economic Record, 79, 279-296.
Cai T., Pepe M.S., 2002. Semiparametric receiver operating characteristic analysis to evaluate biomarkers for disease. Journal of the American Statistical Association, 97, 1099-1107.
Caldato S.L., Durlo M.A., Longhi S.J., 2000. Análisis de la ocurrencia de Ocotea porosa (Lauraceae) a través del método de regresión logística. Bosque, 21, 27-36. [In Spanish]. Carpenter R. 2005. Temas regulatorios y legales de los seguros por índice. Taller Regional BID Innovaciones en la gestión del riesgo de la producción agrícola en América Central, Antigua,Guatemala, 9-12 de mayo de 2005. [In Spanish].
Chambers R.G., Quiggin J., 2002. Optimal producer behavior in the presence of area-yield crop insurance. American Journal of Agricultural Economics, 84, 320-334.
Chi L.C., Tang T.C., 2006. Bankruptcy prediction: application of logit analysis in export credit risks. Australian Journal of Management, 31, 17-27.
Coleman S., 2007. The role of human and financial capital in the profitability and growth of women-owned small fi rms. Journal of Small Business Management, 45, 303-319.
Cox D.R., Snell E.J., 1968. A general defi nition of residuals (with discussion). J R Stat Soc., 3, 248-275.
Fisher R.A., 1922. On the mathematical foundations of the theoretical statistics. Philos Trans Roy Soc London, 222, 309-368.
Golan A., Shalit H., 1993. Wine quality differentials in hedonic grape pricing. Journal of Agricultural Economics, 44, 311-321.
Goldberg P.K., 1995. Product differentiation and oligopoly in international markets: the case of the U.S. automobile industry. Econometrica, 63, 891-951. [ Links ]
Goodwin B.K., Vandeever M.L., Deal J.L., 2004. An empirical analysis of acreage effects of participation in the Federal Crop Insurance Program. American Journal of Agricultural Economics, 86, 1058-1077.
Grunert J., Norden, L. Weber M., 2005. The role of non-financial factors in internal credit ratings. Journal of Banking and Finance, 29, 509-531.
Hanley J.A., McNeil B.J., 1982. The meaning and use of the area under a receiver operating characteristic (ROC) curve. Radiology, 143, 29-36.
Hannan E.J., Quinn B.G., 1979. The determination of the order of an autoregression. Journal of the Royal Statistical Society, 41, 190-195.
Hardaker J.B., Huirne R.M.B., Anderson J.R., Lien G. 2004. Coping with Risk in Agriculture. CABI Publishing, Cambridge, USA.
Ho F.N., Gallagher M.P., 2005. The impact of wine tasting on wine purchases: evidence from Napa, California. International Journal of Wine Marketing, 17, 44-53.
Hopkins S., Kidd M.P., 1996. The determinants of the demand for private health insurance under medicare. Applied Economics, 28, 1623-1632.
Hosmer D.W., Lemeshow S., 1980. A goodness-of-fi t test for the multiple logistic regression model. Communications in Statistics, A10, 1043-1069.
Huber M., 2005. Factors infl uencing the purchase of cotton and rice crop insurance. M.S. dissertation, Texas A&M University - Commerce, Texas, USA.
Knox L., Richards T.J. 1999. A two-stage model of the demand for specialty crop insurance. American Agricultural Economics Association, Summer Meeting. Nashville, Tennessee 1999.
Jobson J.D., 1992. Applied Multivariate Data Analysis. Categorical and multivariate methods. Springer-Verlag, New York.
Johnsen T., Melicher R.W., 1994. Predicting corporate bankruptcy and financial distress: information value added by multinational logit models. Journal of Economics and Business, 46, 269-286.
Just R.E., Pope R.D., 2002. A Comprehensive Assessment of the Role of Risk in U.S. Agriculture. 600 p. Kluver Academic Publisher, Norwell, MA.
Kandemir D., Calantone R., Garcia R., 2006. An exploration of organizational factors in new product development success. Journal of Business & Industrial Marketing, 21, 300-310.
Lennox C., 1999. Identifying failing companies: a re-evaluation of the logit, probit, and DA approaches. Journal of Economics and Business, 51, 347-364.
Lloyd C. J., 1998. Using smoothed receiver operating characteristic curves to summarize and compare diagnostic system. Journal of the American Statistical Association, 93, 1356-1365.
Lobos G., Viviani J.L., 2010. Description des perceptions des sources de risque des producteurs vitivinicoles. Évidence empirique pour le Chili. Économie Rurale, 316, 48-61. [In French].
Lobos G., 2009. La gestion du risque dans lindustrie vitivinicole du Chili : modélisation logistique et simulation des risques specifiques. Doctoral thesis, Université Montpellier 1. [In French].
Lobos G., Viviani J.L., 2008. Un modèle logit binominal de souscriptiondassurances : une application à lindustrie vitivinicole chilienne. Économies et Sociétés, Série Systèmes agroalimentaires, 30, 2253-2269. [In French].
Maddala G., 1996. Introducción a la econometría. 2ª ed. Prentice- Hall, México. 715 pp. [In Spanish].
McFadden D., 1973. Conditional logit analysis of qualitative choice behavior. pp.105-142. In: Frontiers in Econometrics, Zarembka P. (ed.), Academic Press, New York.
Meador J.W., Madden J.P., Johnston D.J., 1986. On the probability of acquisition of non-life insurers. Journal of Risk and Insurance, 53, 621-643.
Meuwissen M.P.M., Huirne R.B.M., Hardaker J.B. 1999, Income insurance in European agriculture. In: European Economy, European Commission, Directorate-general for economic and financial affairs, Reports and Studies, n°2, Luxembourg. pp. 1-15.
Nagelkerke N.J.D., 1991. A note on a general definition of the coefficient of determination. Biometrika, 78, 691-692.
Mishra A., Goodwin B.K., 2003. Adoption of crop versus revenue insurance: a farm-level analysis. Agricultural Finance Review, 63, 143-155.
Morey R.C., Sparks B.A., Wilkins H.C., 2002. Purchase situation modelling in wine selection: an evaluation of factors in an Australian context. International Journal of Wine Marketing, 14, 41-64.
Nielsen R.B., Mayer R.N., 2000. Why do people buy cancer insurance? An exploratory study. Advancing the Consumer Interest, 12, 16-22.
Priilaid D.A., 2006. Wines placebo effect. International Journal of Wine Marketing, 18, 17-32.
Priilaid D.A., Van Rensburg P., 2006. Non-linearity in the hedonic pricing of South African red wines. International Journal of Wine Marketing, 18, 166-182.
Rodríguez M.C., Cáceres J.J., Guirao G., Cano V.J., 2009. Individual profi les and wine consumption patterns in Tenerife. A multinomial logit model. Spanish Journal of Agricultural Research, 7, 759-769.
Sapelli C., Torche A., 2001. The mandatory health insurance system in Chile: explaining the choice between public and private insurance. International Journal of Health Care Finance and Economics, 1, 97-110.
Schwarz G.E., 1978. Estimating the dimension of a model. Annals of Statistics, 6, 461-464.
Shaik S., Coble K.H., Knight T.O., Baquet A.E., Patrick G.F., 2008. Crop revenue and yield insurance demand: a subjective probability approach. Journal of Agricultural and Applied Economics, 40, 757-766.
Skees J.R., Barnett B.J. 1999, Conceptual and practical consideration for sharing catastrophic/systemic risks. Review of Agricultural Factors infl uencing farmers crop insurance decisions. American Journal of Agricultural Economics, 86, 103-114.
Skuras D., Vakrou A., 2002. Consumers willingness to pay for origin labelled wine: a Greek case study. British Food Journal, 104, 898-912.
Smith V., Baquet A.E., 1996. The demand for multiple peril crop insurance: evidence from Montana wheat farms. American Journal of Agricultural Economics, 78, 189-201.
Tan H.B., Wong M.F., Law S.H., 2009. The effect of consumer factors and firm efficiency on Malaysian life insurance expenditure. International Journal Business and Society, 10, 59-73.
Thode S.F., Taylor L.W., Maskulka J.M., 2002. Information asymmetries in the pricing of fine wines. International Journal of Wine Marketing, 14, 5-13.
Tobin J., 1958. Estimation of relationships for limited dependent variables. Econometrica, 25, 24-36. [ Links ]
Troncoso J.L., Aguirre M., 2006. Price determinants of Chilean wines in the US market: a hedonic approach. Spanish Journal of Agricultural Research, 4, 124-129.
Van Rensburg P., 2004. An econometric model for identifying value in South African red wine. International Journal of Wine Marketing, 16, 53-75.
Westgaard S., Wijst N.V., 2001. Default probabilities in a corporate bank portfolio: a logistic model approach. European Journal of Operational Research, 135, 338-349.
Zhou X., Castelluccio P., 2003. Nonparametric analysis for the ROC areas of two diagnostic test in the presence of nonignorable verification bias. Journal of Statistics Planning and Inference, 115, 193-213.
(Manuscrito recebido em 16.10.10 . Aceite para publicação em 09.12.10)