I. Introduction
Airbnb is a hospitality service that allows people to rent their unused rooms or entire apartments through computer-mediated transactions. It was originated in 2008 in the United States and since then it has expanded to 100 000 cities in 191 countries and regions worldwide. According to October 2019 data, it has more than 7 million listings worldwide and on average over 2 million people stay on Airbnb per night (Airbnb, 2020). It is not the only short-term rental service in the market; however, it is certainly the largest service that has approximately twice as many listings as its nearby competitors (for example, Homeaway, Holidu and Housetrip). It generates more annual revenue (around $38 billion) than the most famous hotel chains, such as Hilton and Marriot International (Molla, 2019). Initially, it began with the idea of renting spare rooms in private homes as a way to generate extra income, however, now it has become an actual alternative to the traditional accommodations (Larpin et al., 2019). Airbnb describes its mission as
to create a world where people can belong through healthy travel that is local, authentic, diverse, inclusive and sustainable (…) to economically empower millions of people around the world to unlock and monetize their spaces, passions and talents and become hospitality entrepreneurs’. (Airbnb, 2020)
However, who is the real beneficiary of this platform is still a matter of debate (Quattrone et al., 2016). During the last decade, Barcelona and Lisbon have witnessed an unprecedented growth of Airbnb. The unrestrained expansion of Airbnb in the residential areas of some specific neighbourhoods has disrupted the housing market and increased spatial inequalities in both cities. Recently, the COVID-19 pandemics has slowed the expansion of Airbnb in both cities by reducing the movement of people from one place to another.
Previous research has focused on the impact of Airbnb on tourism (Fuentes & Navarrete, 2016; Ioannides et al., 2018; Kadi et al., 2019), housing market (Cocola-Gant & Gago, 2019; Garcia-López, 2020; Horn & Merante, 2017; Lee, 2016), local businesses (Blázquez-Salom, 2019), hotel industry (Dogru et al., 2018; Heo et al., 2019; Oskam & Boswijk, 2016), gentrification (Cocola-Gant, 2016, 2021; Gravari-Barbas & Guinand, 2017; Lees, 2003), and urban land use regulation (Dalir et al., 2020). In terms of spatial distribution, some researchers have demonstrated that the concentration of Airbnb listings in some specific neighbourhoods creates spatial inequalities and over-crowdedness in big tourist cities, that often have large disparity among the rich and the poor, and over utilised public services (Adamiak et al., 2019; Eugenio-Martin et al., 2019; Quattrone et al., 2016). Recently, some studies have focused on the spatial distribution of Airbnb in selected cities (Lagonigro et al., 2020; Lestegás et al., 2019), but still no attempt has been made to study the extent and determinants of the spatial penetration of Airbnb at the territorial unit level in different neighbourhoods of Barcelona and Lisbon. This article responds to this question and allows us to understand the geographical logic behind the distribution of Airbnb accommodations and its impact on spatial inequalities in both cities. It also scrutinizes the claims made by the proponents of Airbnb that it contributes to the improvement of the most deprived neighbourhoods by bringing tourism related business activities. Ultimately, it also provides valuable information to policy makers concerned with the regulation of short-term rentals. With a clear picture of the extent and determinants of the spatial penetration of Airbnb accommodations, policy makers can frame a better policy to protect the rights of the native population and the long-term rental market in different neighbourhoods of both cities.
Using data from the Inside Airbnb (2020), 2011 Census of population and housing, municipal registers (2011-2019), and Open Street Maps, this article first measures and analyses the degree of penetration and spatial clustering (with LISA statistics) of Airbnb listings in Barcelona and Lisbon; second, it explores the spatial, socioeconomic, demographic and cultural determinants of the spatial clustering of accommodations offered on Airbnb in both cities; and finally, it examines the impact of COVID-19 pandemics on the spread of Airbnb in both cities.
II. Background
Over the last decade, the sharing economy has witnessed a remarkable growth, especially in the transportation (BlaBlaCar, Glovo, and Uber) and tourist accommodation (Airbnb, Homeaway, and Houset) sectors (Forno & Garibaldi, 2015; Fraiberger & Sundararajan, 2015). Among short-term rentals, Airbnb has captured a large share of business worldwide and emerged as the biggest supplier of accommodations for short-term users. As the demand for Airbnb accommodations depends upon the location of different points of interest (tourist attractions, business centres, areas with cultural and political interests) in the host cities, most of the Airbnb listings tend to concentrate in some central parts of the host cities. This tendency to concentrate in the residential areas of some specific neighbourhoods has potential to create new and increase existing spatial inequalities in the host cities. Furthermore, the rapid conversion of the long-term rentals in the working-class neighbourhoods into commercial tourist apartments through Airbnb exacerbates the shortage of long-term rentals, which eventually displace poor native people from their traditional neighbourhoods (Barata-Salgueiro et al., 2017; Cocola-Gant & Gago, 2019; López-Gay et al., 2021).
Recently, COVID-19 pandemics has severely disrupted the Airbnb activity around the world (Bugalski, 2020; Dolnicar & Zare, 2020). The business model of Airbnb depends on the short-time movement of people for work or tourism. The lockdowns imposed in different countries following the COVID-19 pandemics caused a considerable decline in tourism and work-related public gatherings (conferences, business fairs and expositions), which reduced the demand for short term rentals all over the world.
Previous studies on the spatial distribution of Airbnb listings have analysed their location at different scales and its relationship with other elements, such as tourism resources, socio-demographic profile of the resident population, housing market, cultural aspects, and the hotel offerings. Some researchers have studied the spread of Airbnb at the national level (Cesarini & Nechita, 2017; Strommen-Bakhtiar & Vinogradov, 2019), while others focused at the municipal or city level (Adamiak, 2018; Boros et al., 2018). In their study of eight US cities, Quattrone et al. (2018) found that Airbnb listings tend to cluster in central neighbourhoods and in areas occupied by creative classes. Dudas et al. (2017) showed the concentration of Airbnb offerings based on the population’s age, the available housing stock, and the closeness of tourism resources in New York City.
In the European context, Airbnb listings have tended to concentrate in neighbourhoods that have undergone processes of commercial and tourist gentrification (Roelofsen, 2018). In their study of the spatial penetration of Airbnb in London, Quattrone et al. (2016) found that the growth of Airbnb started in central areas as expected by tourist demand, but then extended to the socio-economically deprived peripheral areas of the city. Domènech et al. (2019) showed the importance of the location of ski resorts and second homes in determining the spatial distribution of Airbnb in Switzerland.
In Spain, Adamiak et al. (2019) studied the spatial distribution of Airbnb listings at national level. In Barcelona, Gutiérrez et al. (2017) showed a centre-periphery pattern of Airbnb distribution, in which in the centre Airbnb competes with other tourist accommodations, but in the periphery, it complements the supply of short-term rentals. Similarly, Arias Sans and Quaglieri Dominguez (2016) showed a positive correlation between the presence of hotels and the Airbnb penetration in different parts of Barcelona. Yrigoy (2019), found that the concentration of Airbnb listings is determined by the cultural heritage of the historic centre of Palma de Mallorca city.
In Portugal, Cocola-Gant and Gago (2019) followed the changes in a central street of one of the most traditional neighbourhoods in Lisbon, Alfama, and found a massive shift towards urban requalification and short-term rental. Lestegás et al. (2019) explored the relationship between the spatial concentration of short-term rentals in Lisbon’s historic centre and the phenomena of uneven development and tourism gentrification. Franco et al. (2019) showed that the increase in housing prices as a function of Airbnb concentration is higher in the historical centres and touristic areas in the cities of Lisbon and Porto.
Barcelona is the financial capital and the second most populous city of Spain. It was one of the first cities in Spain where Airbnb started its business in 2009. According to Statistai (2018), it receives approximately 9 million annual tourists, which makes it a perfect market for Airbnb. In 2019, Barcelona had the highest number of Airbnb listings in Spain, i.e., 20 404 active listings in September 2019. Similarly, Lisbon, which is the capital and one of the main tourist cities in Portugal, had the highest number of Airbnb listings in Portugal, i.e., 18 277 in September 2019 (table I). The number of Airbnb listings in both cities have increased sharply during the last decade due to the economic crisis (2008-2013) and the austerity measures adopted by the central governments in both countries. Although Barcelona has more than three times the population in Lisbon, the number of Airbnb listings are very close in both cities.
Table I Key facts on population and Airbnb activity in Barcelona and Lisbon.
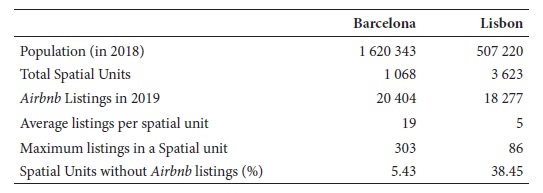
Source: Inside Airbnb (2019); Municipal registers (2018)
In this article, we explore the extent of spatial penetration of Airbnb at territorial unit level in different neighbourhoods of Barcelona and Lisbon and its main determinants in both cities. Additionally, we analyse the impact of COVID-19 pandemics on the number and spread of Airbnb listings and the typology of the accommodations offered on Airbnb in both cities.
III. Data sources and methodology
1. Data sources
Airbnb data was obtained from Murray Cox’s website Inside Airbnbii, which is an independent initiative and not associated with or endorsed by Airbnb or any of their competitors. The website periodically performs web-scraping of the official Airbnb website and publishes snapshots of Airbnb listings in all main cities around the world. For this article, data of all Airbnb listings in Barcelona and Lisbon cities have been downloaded in September 2019 and December 2020. A procedure of data validation was performed by selecting random listings in each city and double checking both their presence on the original Airbnb platform, and the accuracy of their locations. Each listing provides a large amount of information including: host and room identification (a unique number identifies each host and accommodation); location of accommodation (neighbourhood and neighbourhood groups); type of room (entire homes; private rooms and shared rooms); geographic location (latitude and longitude coordinates); details of the accommodation (number of bedrooms; bathrooms and maximum number of guests); availability (the number of days available); price (nightly/weekly or monthly price); and number of reviews (posted by the users on the website).
In order to explain the varying spatial penetration of Airbnb in selected cities, four different sets of variables were used: spatial, demographic, structural and cultural (table II). The variables were obtained from the 2011 Spanish and Portuguese censuses, Spanish continuous population register, Portuguese resident population estimates and other open sources. The first three sources provide population and socio-economic data at census tract (Barcelona) and sub-section (Lisbon) level, which is the smallest territorial unit of analysis available in Spain and Portugal.
In the spatial variables, distance to city centre (dist), points of interest (poi), number of hotels available in a territorial unit (hotel) and access to transport services, i.e., bus or metro stops (trans) are included as explanatory variables. In previous studies, distance to the city centre is considered as one of the most important determinants of the presence of Airbnb in an area (Quattrone et al., 2016). The places closer to the city centre are likely to have a higher number of Airbnb listings and vice-versa. For simplicity and homogeneity, the TripAdvisor website is used to identify the central points of the two cities, which are: Plaça de Catalonia in Barcelona and Praça Dom Pedro IV in Lisbon. For each city, it is the shortest distance in meters between the central point and the centre of the territorial unit under study. The presence of points of interest (geographic features that might be of interest for tourists or short-term visitors, such as historical monuments, restaurants, town halls, post offices, cinemas, adventure parks, stadiums, etc.) in an area is expected to increase its tourist appeal and the number of Airbnb offerings. Open Street Map data is used to count the number and find the location of points of interest in all territorial units. The latitude/longitude coordinates for all points of interest that fall under the following categories: accommodation, attractions, eating and drinking, retail and sports, and entertainment for each selected city are extracted. The availability of hotels in an area is considered an indicator of the potential tourism attraction of that area. The data about the number and geographical location (latitude/longitude) of hotels in all cities was downloaded following the categories: hotel, bread and breakfast, motels, and pensions from the Open Street Maps. Finally, in the spatial variables, easy access to public transport is also considered to increase the number of Airbnb listings in each area. Normally, tourists or other short-term visitors prefer to stay in areas that are well connected with public transport to save some money and time. It concentrates the Airbnb listings around the metro lines or bus stops. The Open Street Maps was used to count the number and geographical location of metro and bus stops available in each territorial unit of the selected cities.
In the demographic variables, population density (popden), proportion of young people between 20-34 years (young), proportion of single person households in a given area of the selected cities (singleph) are used. The density is calculated by dividing the number of people living in a territorial unit by its surface area. In previous studies, high population density is considered to have a crucial impact on the spread of sharing economy services (Fraiberger & Sundararajan, 2015; Quattrone et al., 2016). The variable young, which shows the age structure of the resident population in different neighbourhoods, is expected to have a significant impact on the penetration of Airbnb due to the higher use of modern technology among the younger population (Florida, 2002); the neighbourhoods with high proportion of young population are more likely to have higher number of Airbnb listings compared to other neighbourhoods with high share of old population. Lastly, the variable singleph is also expected to facilitate the spread of Airbnb, since, it is more feasible for a single person to share spare rooms of his/her apartment, as for a family household can be a difficult choice.
In the structural variables, the proportion of unemployed people (unemployment), the average age of housing stock (housecon), average house-rent (houserent), average house-size (housesize), the proportion of vacant apartments (vachouse) and the tenancy regime (owner) are included as explanatory variables. In previous studies, the neighbourhoods with a high proportion of unemployed population are expected to have a high number of Airbnb listings (Quattrone et al., 2016). Similarly, the areas with high average house-rents are expected to have more Airbnb listings, as normally these areas are more attractive to tourists and have better access to other public services (Quattrone et al., 2016). The high proportion of small apartments (less than 60m2) in a neighbourhood can decrease the number of rooms offered on Airbnb, since small apartments have no spare rooms to rent, however, it can increase the number of full apartments available for rent in Airbnb. The high proportion of vacant apartments is considered to have a positive impact on the penetration of Airbnb, by increasing the supply of accommodations that can be rented in Airbnb. Lastly, the high proportion of rented apartments in an area shows its potential as a good market for rental accommodations, which suits Airbnb business model. In addition, the people who have rented an apartment for a long stay are more likely to share their apartment with short-term visitors, compared to the house-owners, who use them as their principal residence (Quattrone et al., 2016).
In the cultural variables, the proportion of residents born in the municipality (native) and the proportion of people with university degrees (education) are included in the study. These variables are used as a proxy to examine the access of population to modern technology, social media sites and sharing economy in different parts of the selected cities (Quattrone et al., 2018). The variable native has tended to have a significant influence on the socio-economic configuration of the specific neighbourhoods, which in turn affects the number of listings in these neighbourhoods. In previous studies, the areas with a high proportion of native population have stated to have a small number of Airbnb listings (Quattrone et al., 2016). Similarly, the high proportion of more educated people is expected to positively affect the penetration of Airbnb in selected cities, and vice-versa (Quattrone et al., 2018).
2. Methodology
The methodological approach was designed in three steps. First, in order to measure the level of spatial penetration of Airbnb (clustering) in the selected cities, the Local Indicator of Spatial Association (LISA) index, which can be seen as the local equivalent of Moran’s I (Anselin, 1995) is applied. It is used to identify whether there are observed similar or alien values agglomerated in a local area. It can be used to measure the degree of correlation between area i and other areas. Since the data is available at the territorial unit level, the variable number of Airbnb listings in territorial units’ is used to perform this analysis. The local value of a LISA is computed as:
is the average number of listings in the number of listings in different territorial units. A local Moran’s I greater than 0 indicates that a high value is surrounded by high values or a low value is surrounded by low values. A value smaller than 0 indicates that a low value is surrounded by high values or a high value is surrounded by low values.
Following the first law of geography, “everything is related to everything else, but near things are more related than distant things” (Tobler, 1970, p. 236), it is hypothesized that territorial units with higher or lower number of Airbnb listings will be clustered in certain areas of selected cities. The parameter for conceptualizing spatial relationships between territorial units was “queen contiguity”, which ensures that all the entities have a maximum number of neighbours possible in all directions. For each location, LISA values allow for the computation of its similarity with its neighbours and to test its significance. Five scenarios may emerge: first, locations with high values of Airbnb with similar neighbours (high-high), also known as hot spots; second, locations with low values with similar neighbours (low-low), also known as cold spots; third, locations with high values with low-value neighbours (high-low), potential spatial outliers; fourth, locations with low values with high-value neighbours (low-high), potential spatial outliers; and fifth, locations with no significant local autocorrelation. These specific configurations can be first identified from a Moran scatterplot showing observed values against the averaged value of their neighbours. Once a significance level is set, values can also be plotted on a map to display the specific locations of hotspots and potential outliers.
Then, regression analysis has been carried out to identify the main determinants of the spatial penetration of Airbnb listings in the selected cities. Because of the Poisson distribution of the dependent variable, i.e., the number of Airbnb listings by territorial unit in all selected cities, Count Data Models (CDMs) have been applied (fig. 1). These models are especially useful when the analysed events are recorded at a highly disaggregated territorial level and a significant number of spatial units have zero occurrence of dependent variable, a situation known as “zero problem”. However, the existence of territorial units with zero listings is relevant for our study as it allows us to identify the independent variables that can explain the absence of Airbnb listings in certain areas of the selected cities. In our study, the number of territorial units in each selected city is large and in some territorial units zero Airbnb listings are reported, which justifies the use of CDMs. The performed spatial analysis (LISA indicator) has shown that there is a high spatial clustering of Airbnb listings in specific areas of selected cities. Hence, to solve the problem of over dispersion and zero-inflation, a negative binomial regression model (NBM) has been applied. Additionally, the choice of model has been supported by the results from Akaike’s Information Criteria (AIC) and Bayesian Information Criteria (BIC). With a view to the general features of this estimation procedure, NBM assumes between-subject heterogeneity and that the process is the same for both zero and non-zero counts (Domènech et al., 2019).
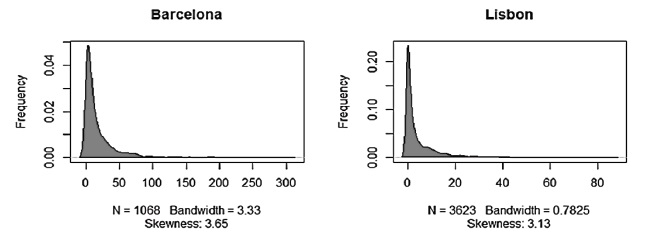
Fig. 1 Density of the dependent variable (Airbnb listings) for Barcelona and Lisbon. Source: Inside Airbnb (2019), extracted from Count Data Models
Taking the territorial unit as a statistical unit and the number of Airbnb listings per territorial unit as dependent variable, two general non-linear NBM have been applied (McCullagh & Nelder, 1989). All the variables shown in table II have been included as explanatory variables. Correlation among them has been checked (fig. 2) and multicollinearity (Cameron & Trivedi, 2005) has also been controlled with satisfactorily low values of the variance inflation factors (VIF). Following the previous studies, the threshold for VIF was set at 3 (Pan & Jackson, 2008; Rogerson, 2001). Some explanatory variables have shown high correlation.
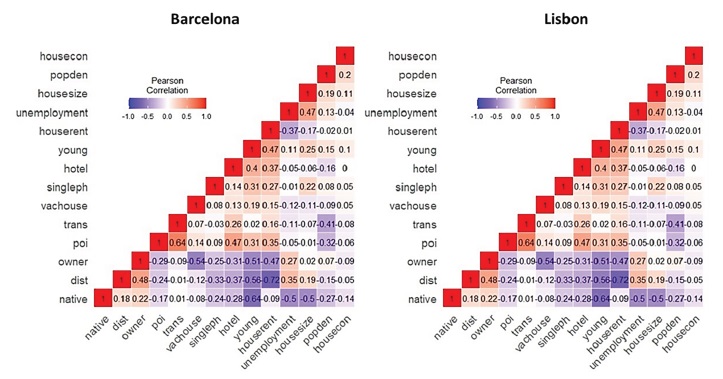
Fig. 2 Correlation among explanatory variables for Barcelona and Lisbon. Colour figure available online. Source: Inside Airbnb (2019); Municipal registers (2018); Census 2011; Open Street Maps (2019)
Two regression analyses have been implemented for both cities to solve the problem of multicollinearity. In the first model, all variables are included; in the second model, one of the explanatory variables (in the pair of highly correlated variables) has been excluded. It ensures that we correctly capture the effect of each independent variable on the dependent variable. It also allows us to see which model presents better goodness of fit indicators. The regression models are formulated as:
IV. Spatial penetration of airbnb in barcelona and lisbon
1. Spatial clustering of Airbnb
The big red spots (high-high cluster) on both maps and high values of the LISA indicator show the spatial clustering of Airbnb accommodations in the central neighbourhoods of Barcelona and Lisbon (fig. 3).
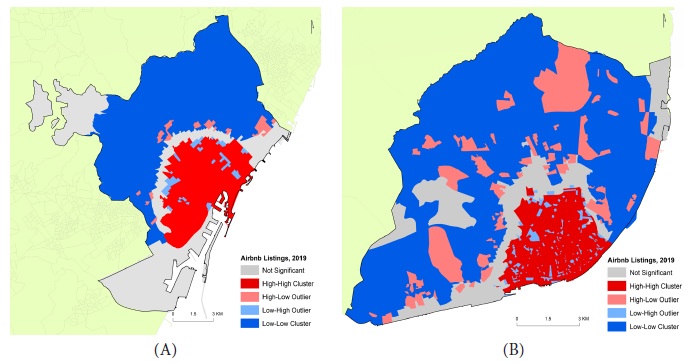
Fig. 3 Local Moran’s spatial autocorrelation indicator with the variable Airbnb listings per territorial unit in Barcelona (A) and Lisbon (B). Colour figure available online. Source: based on Inside Airbnb (2019)
It confirms our assumption that the spatial penetration of Airbnb is highly uneven in both cities. Barcelona has a high value of Moran’s I, i.e., 0.79, which shows the existence of different high-high or low-low clusters of Airbnb listings in different neighbourhoods of the city. In Barcelona, the Airbnb listings were concentrated in the historical down-town areas. These areas are already known for their tourist attractions, businesses, and cultural heritage (López-Gay et al., 2021). In 2019, 58.7% of the total listings in Barcelona were concentrated in the central neighbourhoods of la Dreta de l’Eixample (10.2%), el Raval (8.7%), el Barri Gotic (6.7%), Sant Pere, Santa Caterina i la Ribera (6.1%), el Poble Sec (5.5%), l’Antiga Esquerra de l’Eixample (5.5%), la Sagrada Familia (5.4%), la Vila de Grácia (5.4%), and Sant Antoni (5%). All these neighbourhoods form a very big high-high cluster in the historical centre and coastal areas of the city. These neighbourhoods have a large number of historical monuments, religious places, business houses, and government offices that attract annually a large number of short-term visitors. At the same time, the peripheral neighbourhoods of Baro de Viver (0.01%), Can Peguera (0.01%), Canyelles (0.02%), Torre Baro (0.02%), Ciutat Meridiana (0.03%), la Trinitat Nova (0.05%), and la Vall d’Hebron (0.05%) had below 0.1% of the total Airbnb listings in Barcelona. These neighbourhoods form a low-low cluster in the northwest of the city.
Similarly, Lisbon with the Moran’s I value of 0.55 also shows spatial clustering of Airbnb listings in the central historic parts of the city. In 2019, 77.9% of the total Airbnb listings were concentrated in the central parishes of Santa Maria Maior (20.9%), Misericórdia (17%), Arroios (12.9%), Santo António (8.2%), São Vicente (8%), Estrela (6.1%) and Avenidas Novas (4.2%). These parishes form the historical and the business centres of Lisbon and are known for their cultural heritage and tourist attractions (Lestegás et al., 2019). At the same time, the outer parishes of Santa Clara (0.1%), Carnide (0.3%), Benfica (0.5%), Marvila (0.5%), Beato (0.6%) and São Domingos de Benfica (0.96%) had less than one percent of the total listings in Lisbon.
2. The main determinants of spatial clustering of the Airbnb listings
The first model for Barcelona and Lisbon shows that in the spatial determinants, the distance to the centre of the city had a highly significant negative impact on the penetration of Airbnb in both cities; however, the impact was stronger in Barcelona than in Lisbon. It means as one moves far from the centre of the city the number of Airbnb listings decreases. It was expected as short-term visitors prefer to stay close to the centre of the city, which are often well equipped to serve their needs. The presence of the points of interest for tourists and other short-term visitors had a positive impact on the penetration of Airbnb in both cities, which is easy to understand as these tourist spots attract thousands of tourists annually, who are the main clients for short-term rentals. The presence of hotels has a less significant negative impact on the penetration of Airbnb in Barcelona. However, it has no impact on the spread of Airbnb listings in Lisbon. Finally, the easy access to the public transport services facilitates the spread of Airbnb in Lisbon, but in Barcelona its impact is statistically insignificant.
In the demographic determinants, the high population density had a highly significant negative impact on the penetration of Airbnb in Barcelona and a highly significant positive impact in Lisbon. It means in Barcelona, the territorial units with high population density had a smaller number of Airbnb listings and, on the contrary, in Lisbon subsections with high population density had a large number of listings. The concentration of young people had shown no statistically significant impact on the penetration of Airbnb in Barcelona, but it had a statistically significant negative impact on Airbnb listings in Lisbon. Since the areas with high concentration of young people could theoretically explain a high number of listings in Lisbon this effect can be the result of neoliberal housing policies and housing financialization (Mendes et al., 2019) that overlooked the young people. Lastly, both cities had shown a highly significant positive impact of the concentration of single person households on the penetration of Airbnb. However, in Lisbon its impact was stronger and highly statistically significant than Barcelona, which can be justified by the (mis)matches between an aged population and an oversupply of large houses (Garha & Azevedo, 2021).
In the structural determinants, the proportion of unemployed people had a statistically significant negative impact on the penetration of Airbnb in Lisbon. On the contrary, its impact in Barcelona was statistically insignificant. Every increase in the average house-rent had a positive impact on the supply of Airbnb accommodations in Barcelona, which means neighbourhoods with high average monthly rents have high penetration of Airbnb. On the contrary, it had a statistically insignificant impact on the spread of Airbnb in Lisbon. The high proportion of small apartments had a highly significant positive impact on the penetration of Airbnb in Barcelona and a negative impact in Lisbon. The high proportion of old housing stock had a negative impact on the spread of Airbnb, while it has no impact in Lisbon. The high proportion of vacant apartments had a statistically significant positive impact on Airbnb in Lisbon. However, its impact was statistically insignificant in Barcelona. Lastly, the high proportion of people who own the house where they live (owners) had a statistically significant negative impact on the penetration of Airbnb in Lisbon. In Barcelona its impact was statistically insignificant.
Lastly, in the cultural determinants, the concentration of native population in some traditional neighbourhoods had a negative impact on the penetration of Airbnb in both cities, with stronger impact in Barcelona than Lisbon. The high proportion of people with university education had a statistically significant positive impact on the penetration of Airbnb in Lisbon. However, in Barcelona, its impact was not statistically significant.
As we have seen in the correlation plots (fig. 2), there are some independent variables that are highly correlated. To avoid the problem of multicollinearity, we have calculated the VIF for each independent variable used in the first models for all selected cities. The highly correlated pair of variables (with VIF value more than 3) are identified in each first model and one of the variables from the highly correlated pairs is dropped to run the second model. In Barcelona, high correlation is found between the variables native and young (VIF of >3). Therefore, in the second model, the variable native was dropped. It increased the significance of the variables young and unemployment, as determinant factors that positively affect the penetration of Airbnb. In Lisbon, high correlation is found between the variables singleph and dist (VIF of >3), therefore, in the second model, the variable singleph is dropped. It decreases the significance of the variable young, which was significant in the first model. In addition, it increases the significance of housecon and houserent as determining factors that positively affect the penetration of Airbnb in the city. The AIC, BIC, and the Log-Likelihood values of both models show a significant improvement in the goodness of fit of the second model, which justifies the dropping of one variable from the couples of highly correlated independent variables (table III).
V. Impact of COVID-19 pandemics on Airbnb listings
The recent COVID-19 pandemics has a very significant impact on the spread and penetration of Airbnb in Barcelona and Lisbon. Airbnb’s business model depends on the short-term movements of people from one city to another for work or tourism. The recent pandemics has significantly curtailed the movement of people around the world. It resulted in a considerable fall in the demand for short-term accommodations. This fall in demand forced people to withdraw their apartments from the Airbnb website. In Barcelona and Lisbon, the number of total listings has reduced from 20 404 and 18 277 in September 2019, when the COVID-19 pandemics began to spread around the world, to 19 641 and 14 332 in December 2020, respectively (fig. 4). In Barcelona, the reduction in the number of Airbnb Listings was 3.7%, while in Lisbon it was 21.6%. This decline was spatially concentrated in the central neighbourhoods of both cities. In Barcelona, the neighbourhood of Raval (13.3%), Poble Sec (13.3%), la Vila de Gracia (9.1%) and Sant Antoni (8.3%) have recorded the highest loss of Airbnb Listings. Similarly, in Lisbon the parishes of Misericórdia (22.3%), Arroios (25.1%), Santa Maria Maior (14.1%), Santo António (20.3%) and Estrela (24.9%) have registered even higher loses in comparison with neighbourhoods of Barcelona. In Lisbon, not a single parish has witnessed an increase in the number of Airbnb listings, while in Barcelona, the neighbourhoods of la Dreta de l’Eixample (5.1%), Sant Gervasi-Galvany (23.1%) and el Barri Gotic (4.2%) have recorded an increase in the number of Airbnb listings.
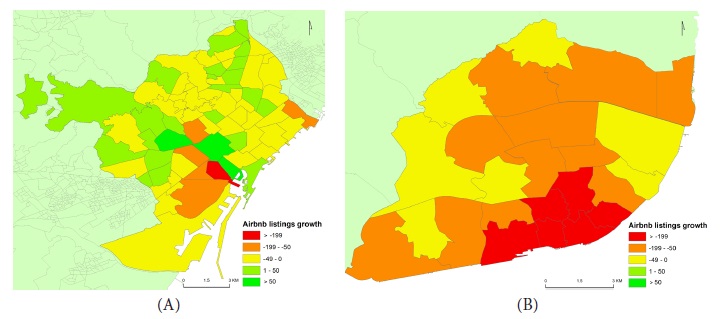
Fig. 4 Impact of COVID-19 on the number of Airbnb listings in (A) Barcelona and (B) Lisbon. Colour figure available online. Source: Inside Airbnb (2019)
In addition to the decline in the number of total listings, the composition of the apartments offered on Airbnb has also witnessed a considerable change during the last year (figs. 5 and 6). In Barcelona, the highest decline is registered in the number of private rooms; at the same time, the number of listings of full apartments has increased considerably in all central neighbourhoods. It shows that the main idea of Airbnb - sharing home with others - has failed in Barcelona due to COVID-19 pandemics. People in Barcelona are not willing to share their homes with strangers due to the risk of COVID-19 infection. But still, the number of entire apartments in Airbnb listings, in which the risk of infection is low, has increased. Contrary to this trend, in Lisbon the number of all types of accommodations in Airbnb listings has decreased, with a small exception of shared rooms in Arroios and Alcântara parishes.
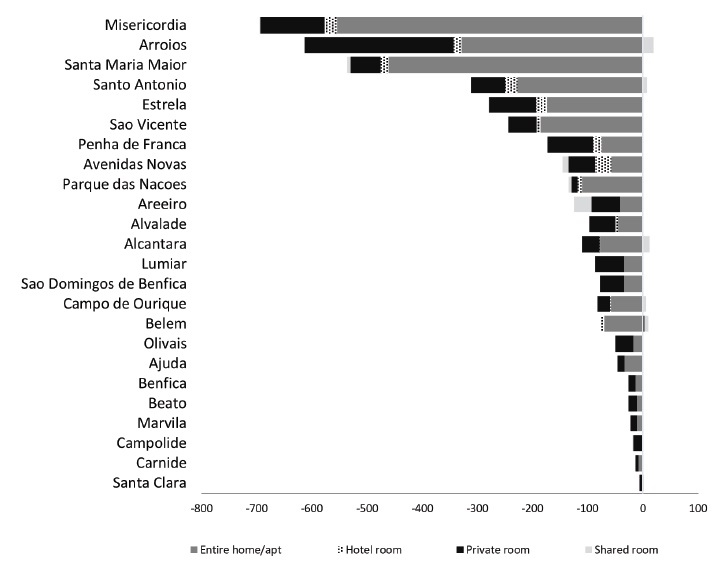
Fig. 5 Evolution of the typologies of accommodations offered on Airbnb by Lisbon`s parishes, 2019-2020. Source: Inside Airbnb (2019)
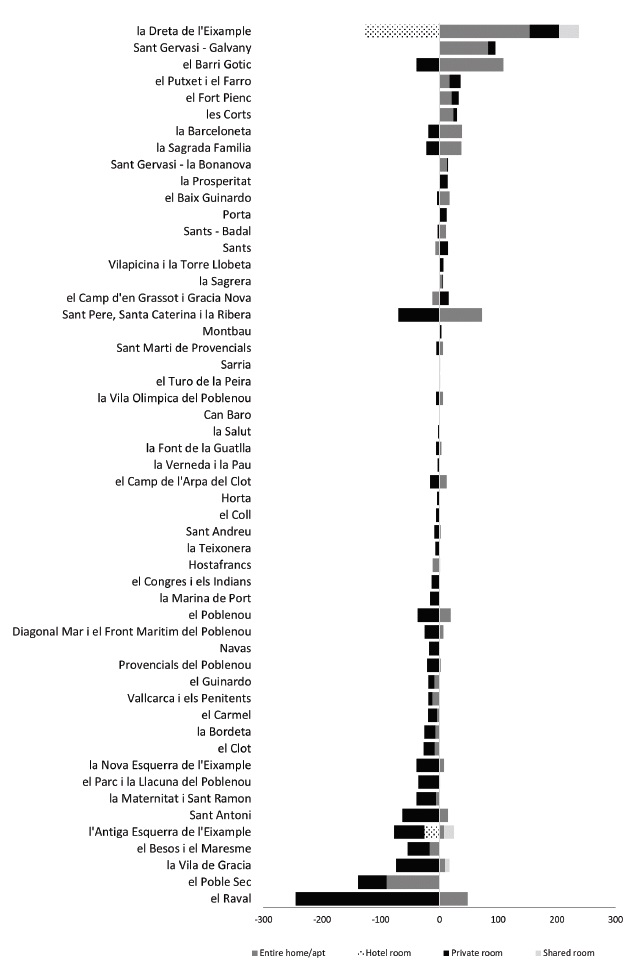
Fig. 6 Evolution of the typologies of accommodations offered on Airbnb in Barcelona, 2019-2020. Source: Inside Airbnb (2019)
VI. Concluding remarks
The result of spatial analysis demonstrates that most of the Airbnb accommodations are concentrated in the central parts of the selected cities. These areas are traditionally known for their high tourism potentials, and always remained favourite destinations for the national and international tourists. It directs the flow of revenue to these neighbourhoods and refutes the claim of the Airbnb’s proponents that it brings tourism to the peripheral neighbourhoods of the cities, which traditionally do not receive tourists. In this article, we empirically proved that Airbnb concentrates tourism revenue in some areas that are already overwhelmed with tourists. Therefore, the benefits of Airbnb do not reach the socioeconomically marginalized and vulnerable neighbourhoods of both cities. These neighbourhoods do not receive any increase in tourism, rather, they suffer from the ill effects of touristification of their local businesses, city centres and public services. As a result, Airbnb activity is deepening existing and creating new spatial inequalities in Barcelona and Lisbon.
The regression models have highlighted the effect of different determinants underneath the spatial distribution of Airbnb listings. The results indicate nine features with a positive impact on the penetration of Airbnb in the residential areas in Barcelona and in Lisbon: short distance to the city centre, concentration of the points of attraction for tourists, low population density, large houses, large proportion of single-person households, high tenancy rates, high average household income, large proportion of highly educated people, and high share of immigrants. Conversely, the large proportion of natives in the total population and high home-ownership rates reduce the spread of Airbnb activities in these two cities.
The COVID-19 pandemics has curtailed the growth of Airbnb in Barcelona and in Lisbon. Since there are still many restrictions on the movement of people from different countries, it is expected that Airbnb will incur more losses in the coming times. The central neighbourhoods of both cities have registered loss in the number of Airbnb listings due to the pandemics The typology of accommodations offered on Airbnb has also changed, with a considerable decrease in the number of shared and private rooms in all neighbourhoods and an increase in the number of entire houses in some neighbourhoods. Some researchers have claimed that the native people in the central neighbourhoods are happy with this contraction of Airbnb and they are expecting a return of the short-term rentals to long-term rentals in the coming months. In previous research, Airbnb was criticised as a force of disruption for the housing market, now it is suffering from the same type of disruption due to pandemic in both cities.
Airbnb has also affected the lives of ordinary citizens by transforming their houses into commercial assets that can be rented for some extra income. It makes people lose the sense of neighbourhood or attachment to their neighbourhoods and commercializes everything. The high presence of tourists in residential areas increases congestion and competition for already scarce public services.
These results signal towards an urgent need for regularisation of Airbnb to make it a spatially just service. Some of the steps have already taken by the local governments in both cities to control the adverse effects of Airbnb (i.e., Ley de Propiedad Horizontal [Horizontal Property Law] de 2019 in Barcelona and Regulamento Municipal do Alojamento Local [Municipal Regulation of Local Accommodation] de 2019 in Lisbon) and recently COVID-19 pandemics has contributed to the decline of Airbnb activity in both cities. In Lisbon, the Renda Segura programme 2020 allows private landlords to rent their homes to the municipality which will then rent them out at lower prices. Although the take-up of this programme is far below expectations, it could be an instrument of change towards correcting the distorted market.
In the future, it will be interesting to see how the restrictions imposed by the local governments effects the penetration of Airbnb in different parts of the host cities and how the Airbnb makes itself more spatially just service that in addition to multi-hosts also benefits the poor small house-owners living in marginalized neighbourhoods of the selected cities.
Author contributions
Nachatter Singh Garha: Conceptualization; Methodology; Software; Formal analysis; Investigation; Data curation; Writing - original draft preparation; Writing - review and editing; Visualization. Alda Botelho Azevedo: Conceptualization; Validation; Formal analysis; Investigation; Resources; Data curation; Writing - review and editing; Supervision; Project administration; Funding acquisition.