Serviços Personalizados
Journal
Artigo
Indicadores
Links relacionados
Compartilhar
Portugaliae Electrochimica Acta
versão impressa ISSN 0872-1904
Port. Electrochim. Acta vol.34 no.1 Coimbra jan. 2016
https://doi.org/10.4152/pea.201601063
Wear Analysis of Electroless Ni-P Coating Under Lubricated Condition Using Fuzzy Logic
Arkadeb Mukhopadhyay , Santanu Duari , Tapan K. Barmanb and Prasanta Sahoo*
Department of Mechanical Engineering, Jadavpur University, Kolkata - 700032, India
Abstract
Since its inception, the utilization of electroless nickel coatings in industries has increased by leaps and bounds, due to their excellent mechanical/electrical properties, hardness, high corrosion and wear resistance and low coefficient of friction. Their behavior in lubricated environments is an interesting subject of research and needs more attention. In the present study, the wear behavior of electroless Ni-P coating under lubricated condition has been investigated. Electroless Ni-P coating has been deposited on mild steel substrate. Characterization of the deposited Ni-P coating has been done using scanning electron microscopy (SEM), energy dispersive X-ray (EDX) analyzer and X-ray diffraction (XRD) technique. A prediction model for the wear depth of the deposits with varying normal load, sliding speed and sliding time has been developed using multiple regression analysis and fuzzy logic, which is a very efficient artificial intelligence technique for modeling and monitoring systems. Experiments are carried out according to Taguchi's L27 orthogonal array of experiments. The results obtained from the prediction models are seen to be in good agreement with experimental results. The applied normal load and sliding time are found to have a significant influence on the wear of electroless Ni-P coating. The wear mechanism was found to be mild abrasive in nature.
Keywords: Electroless Ni-P coating, Friction, Wear, Lubricated condition, Fuzzy Logic.
Introduction
With the development of electroless nickel (EN) plating technique in the middle of the twentieth century by Brenner and Riddell [1], a revolution swept into the field of surface coatings technology. An electroless bath consists of an aqueous solution of metal ions, reducing agent, stabilizers and complexing agents which operates within a certain range of pH and temperature.This is an autocatalytic process, wherein the cation of the metal to be deposited is reduced onto the substrate by the electrons released due to the oxidation of a reducing agent present in the aqueous solution. Once the initial layer of nickel is deposited, the process continues until the nickel source gets exhausted. The electroless deposits have enhanced mechanical and tribological properties. Moreover, they eliminate the use of electricity, and hence, a wide variety of surfaces can be coated with ease. One of the principal advantages of this method is that the deposit thickness obtained is uniform, i.e., a sharp edge and a blunt hole receive the same amount of deposition. The properties of EN coatings can also be varied by modifying coating bath parameters [2].
Electroless Ni-P coating is one of the most famous variants of the EN coating method, and has excellent tribological properties, such as high hardness, wear resistance, corrosion resistance, lubricity etc., and is widely used either as protective or decorative coatings in many industries, including petroleum, chemical, plastic, optics, printing, mining, aerospace, nuclear, automotive, electronics, computer, textile, paper, and food [3 - 5]. The hardness of the deposits depends largely on the phosphorus content and also on the heat treatment temperature [6 -9]. The hardness of as-deposited coatings varies from 500 to 700 HV0.1, as the content of P is varied from 3 to 14% by weight [8]. On heat treatment, the hardness again increases due to the formation of Ni crystallites and precipitation of nickel phosphides (Ni3P, Ni2P). Previous studies show that a record high hardness value of the as-deposited coating can be achieved for a phosphorus content of 7.97 atomic weight percent [10]. With the increase in the hardness value, the wear resistance also increases accordingly and, hence, can be used as a wear resistant coating in several applications. Sahoo [11] has obtained the optimal composition of coating bath parameters for minimum wear of the deposits by using Taguchi method. The optimal combination of tribo-testing parameters for minimum friction coefficient and wear under dry testing condition has also been obtained by Sahoo and Pal [12]. In another study it was revealed that the incorporation of Ni-P coating on 1018 carbon steel has lowered the kinetic coefficient of friction of the substrate, irrespective of its heat treatment condition [13]. The wear resistance and friction behavior of the deposits can be further improved by incorporating a third element such as Ni-P-W and Ni-P-Cu [14 -18]. PTFE and MoS2 particles are seen to significantly reduce the coefficient of friction of electroless Ni-P coating [19 22]. Hard particles such as diamond and Al2O3 have been incorporated successfully along with Ni-P to reduce the wear of the coatings [23 -26]. The choice of this additional element depends on the application for which it is required. The tribological behavior of electroless Ni-P coating improves significantly under the influence of a lubricant. The evolution of friction coefficient with sliding speed agrees well with classical Stribeck curves under bio-oil lubricated condition [18]. The addition of PTFE particles to Ni-P coating is particularly helpful in reducing the friction coefficient when boundary lubrication condition exists [27]. The optimal combination of tribo-testing parameters for minimum friction and wear of Ni-P coating under engine oil lubricated condition using Taguchi method was determined by Duari et al. [28].
On the other hand, fuzzy logic, an artificial intelligence technique, has proved to be a very useful tool for modeling and analysis of complex inter-relationships between process parameters and response variables. Fuzzy logic was first presented by Zadeh in the year 1965 [29]. The basis of fuzzy logic is the âlinguistic variableâ and it is efficient in dealing with uncertain and imprecise data. It tries to mimic human behavior in decision taking. Several manufacturing processes have been successfully modeled using fuzzy logic [30 -35].
From literature review it can be deduced that most of the studies related to electroless Ni-P coating has been done regarding the inspection of tribological properties and its optimization, phase transformation, corrosion resistance and the effect of incorporating a third element along with Ni-P on its tribological properties. The use of a comprehensive design of experiments technique along with an artificial intelligence based expert system can provide a quick solution to design engineers in situations where a practical approach while making quick decisions regarding the suitability of Ni-P coating for a particular application is infeasible. Hence, the objective of this study is directed towards the same goal, but under lubricated condition, since the tribology of mating surfaces is significantly affected under lubrication. An attempt has been made to model the complex relationship between tribo-testing parameters (applied normal load, roller speed and test duration) and wear of the deposits under lubricated condition - on a block-on-roller set-up using a second order regression equation and fuzzy logic -, and also to strike a difference between the prediction capabilities of both methods. Mild steel (AISI 1040) has been used as substrate material for the deposition of Ni-P coating. Surface morphology, composition and phase transformation analysis of the deposits has been done using scanning electron microscope (SEM), energy dispersed X-ray (EDX) analyzer and X-ray diffraction (XRD) analyzer. The planning of experiments has been done based on Taguchi's L27 orthogonal array for the collection of data. The obtained data were then used as knowledge base to formulate the 'if-then' rules of the fuzzy model, and also to generate a second order regression equation. Surface plots indicating the variations of wear depth considering the combined effect of the process parameters have been generated using the fuzzy model. Statistical analysis of variance (ANOVA) has been carried out on the experimental data to determine the significant parameters and their interactions in controlling the wear of the deposits. Finally, worn out surface of electroless Ni-P coating has been viewed under SEM to determine the prevailing wear mechanism under lubricated condition.
Regression analysis
In complex engineering problems, the response of a system is dependent upon several independent variables, and their interdependence is quite difficult to analyze and predict, which is necessary for the process control and optimization. If there is a single dependent variable or response y which depends upon k independent or regressor variables (x1, x2, x3, ..., xk), then the relationship between variables and the response can be characterized by a mathematical model called regression model [36]. If a linear function of the independent variables is found to be efficient in modeling the response with accuracy, then the approximating function is called a first order model and can be expressed as:

where, βj (j = 0, 1, ..., k) is known as the regression coefficient and ε represents the error.
But to accommodate the curvature in a system, a polynomial of higher degree, known as a second order model, which considers the interaction and square terms of the regressor variables, must be used. It can be expressed as [36]:

Developing an empirical model like the one mentioned above is quite easy and cost-effective, though representing a highly non-linear and complex system mathematically using regression method is quite difficult.
Fuzzy logic
Fuzzy logic analysis finds its application where information regarding a system, and variables affecting the same, are uncertain and vague. It is a mathematical modeling technique that mimics the reasoning process of human beings in terms of linguistic variables. The outcome is an expert system having the capability to model and take decisions like human intuition. Fuzzy logic method is based on the fuzzy set theory. In the classical set theory, the membership of an object is 0 or 1 i.e., irrespective of belonging or not to the set. On the other hand, a fuzzy set maps an object onto the unit interval. A fuzzy set assigns membership values between 0 and 1 to objects.
A fuzzy system consists of a fuzzifier, an inference engine, a knowledge base and a defuzzifier. A fuzzy logic controller has been depicted in Fig. 1.
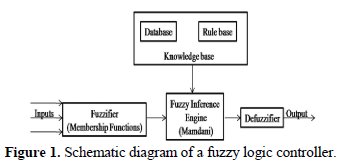
The fuzzifier converts the inputs to a fuzzy value using membership functions. The assignment of membership functions can be based on human intuition or some algorithmic operations such as genetic algorithm, neural networks, soft partitioning and fuzzy statistics [37]. Commonly used membership functions are triangular, trapezoidal, Gaussian and sigmoidal, out of which triangular is the simplest. The fuzzy inference engine then invokes the IF-THEN rules formulated from the knowledge base to generate a fuzzy output. For a three input and a single output, the IF-THEN rules take the following form:

where Ai, Bi, Ci and Di are the fuzzy subsets defined by the corresponding membership functions, i.e. μAi, μBi, μCi and μDi and i = 1 to n (number of rules).
The fuzzy multi-response output y0 is provided from the above rules by employing Mamdani's max-min inference operation. Inference results in a fuzzy set with membership function for the multi-response output and can be expressed as follows:

where ∧ and ∨ are the minimum and maximum operation, respectively. Finally, the fuzzy multi-response output μDi must be converted to a non-fuzzy value y0. This is done by the de-fuzzifier. In this study, the centroid defuzzification method has been selected, giving the area centre of the combined rules obtained in the output. The centroid of the combined rule outputs can be represented as:

The non-fuzzy value y0 gives the output in 'crisp' or numerical form.
Experimental details
Preparation of substrate and deposition of Ni-P coating
Mild steel (AISI 1040) with a dimension of 20 × 20 × 8 mm has been chosen as substrate material for the deposition of electroless Ni-P coating. The substrate is first freed of foreign matter and corrosion products by wiping and then rinsing it in deionized water. Then the substrates are given an etching treatment using 50% hydrochloric acid (for 1 min), and finally rinsed in methanol, followed by deionized water.
The electroless bath for the deposition of Ni-P coating has been obtained by mixing the chemicals as given in Table 1, and in appropriate sequence.

The composition and deposition parameters of the electroless bath have been determined after literature review and several experiments. The chemicals are weighed on an electronic balance of high resolution, in order not to compromise the accuracy of the bath composition (Afcoset, India, Model No. ER182A, Maximum Range 180 gm, VACC 0.01 gm, Class-II). Nickel chloride and nickel sulphate are used as the source of nickel; sodium hypophosphite as the reducing agent; and sodium succinate as the stabilizer. Prior to immersion in the electroless bath, the substrates are activated by dipping in a palladium chloride (of about 0.01% strength) solution kept at 55 °C. Activation of the substrate is necessary to kick start the deposition process. Moreover, activation leads to a higher rate of deposition and good bonding of the coating with substrate, resulting in the deposition of a Ni-P coating considerable thick [38]. After activation, the samples are immersed into the electroless bath maintained at 80 2 °C. The pH of the solution is maintained at 4.5. The deposition is carried out for about 2 hours, and the thickness of the deposit was found to vary within 3035 μm. The deposition parameters are kept constant, so that all the specimens receive more or less the same amount of deposition. As heat treatment is found to have a positive influence on the hardness and wear resistance of electroless coatings [11], the coated samples are separately annealed in a box furnace (for 1 h), at a temperature of 400 °C, followed by slow cooling in the furnace.
Coating characterization
To ensure the proper deposition of electroless Ni-P coating onto the substrate, coating characterization has been done. The surface morphology, composition and the phases present in the electroless deposits influence the wear phenomenon to a large extent. Hence, a study of the morphology and composition of the deposits is very much needed. Coating characterization also reveals the changes that have taken place in the phases and composition of electroless Ni-P coating due to heat treatment. Surface morphology of the coating has been studied using scanning electron microscopy (SEM) (JEOL, JSM 6360 and FEI Quanta 200). Energy dispersive X-ray analysis (EDX) (EDAX Corporation) has been done in conjunction with SEM to determine the composition of coating in terms of weight percentages of nickel and phosphorus. The phase analysis of electroless Ni-P coating before and after heat treatment has been done with the help of X-ray diffraction (XRD) analysis (Rigaku Miniflex). Coating characterization has been done before subjecting the coated specimens to wear tests. SEM has been done also after the wear tests to determine the prevalent mechanism governing the wear phenomenon.
Wear tests
The wear tests are carried out in a multi-tribotester (TR-25, DUCOM) with a plate-on-roller arrangement. Since the present study, attempts to model the nonlinear wear behavior of electroless Ni-P coating with varying applied normal load (N), roller speed (rpm) and test duration (min) have been made. Those parameters have been varied at three different levels, as shown in Table 2.
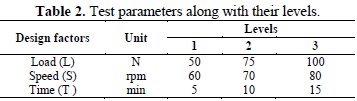
The lubricant used is Servo PRIDE-40, a commercially available product of Indian Oil used in automobile engines.
The tests have been carried out at an ambient temperature of 33 °C. The coated specimens are held stationary with the help of an attachment against a rotating hard chromium coated steel roller (dia 50 mm and thickness 20 mm), conforming to EN8 specification and with a hardness value of 55 HRc. The rotating roller acts as counterface material for the stationary Ni-P coated plate. The speed of the roller and duration of the tests can be controlled using a computer attached to the tribo-tester. Load is applied onto the specimen via a 1:5 ratio loading lever carrying a loading pan on one end, on which dead weights can be placed. The loading lever is pivoted near the normal load sensor. The wear depth is indicated and recorded on-line and is measured with the help of a linear voltage resistance transducer. The sensor allows measurement of the deflection of the loading lever, which is a direct indication of the wear of the coated specimen, as well as of the counterface material. Since the hardness of the specimen (∼45 HRc) is found to be lower than that of the counterface material, the indicated wear can be assumed to be of the coated specimen only. In this study, wear is indicated in terms of displacement (μm). For the data collection, Taguchi's L27 orthogonal array (OA) has been used. Since the degrees of freedom (DOF) of the individual design variables and their interactions taken together came out to be 18, an experimental design having a higher DOF needs to be selected. Hence, L27 OA having a DOF value 26 has been chosen.
Results and discussions
Surface morphology, composition and microstructure analysis
The surface morphology of as-deposited as well as heat treated (400OC for 1h) electroless Ni-P coating is investigated using SEM, to analyze the microstructural changes due to heat treatment, and has been illustrated in Fig. 2.
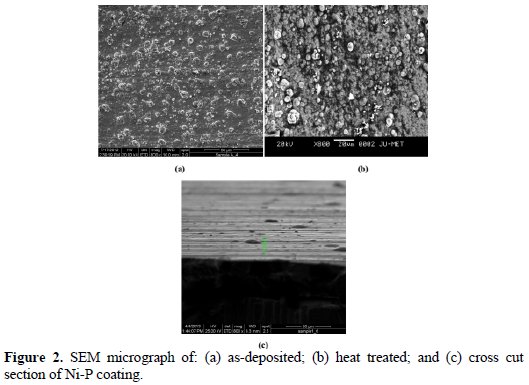
From the SEM micrograph of as-deposited coating (Fig. 2(a)), it can be observed that many globular particles exist, and that the surface has low porosity. On heat treatment, the size of the nodules increases, and the surface appears to be denser and coarser grained (Fig. 2(b)). The self-lubricating nature of electroless nickel coatings can be attributed to their surface morphology [4, 11], which results in lower wear of the coatings. SEM micrograph of cross cut Ni-P coating has been given in Fig. 2(c), which confirms the thickness and uniformity of the coating.
The content of phosphorus present in the deposits has a significant effect on the hardness and, consequently, on the wear resistance of EN coating. With increasing P content in the coating, the amorphous phase increases, which results in a decrease of EN coating hardness [4, 13]. To determine the composition of the deposited coating, an energy dispersive X-ray (EDX) analyzer has been used. The EDX spectrum of as-deposited and heat treated Ni-P coating has been given in Fig. 3(a) and Fig. 3(b), respectively.
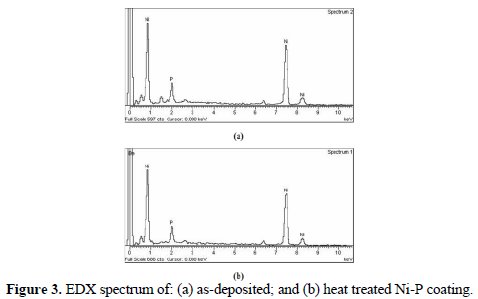
The peaks of Ni and P are quite specific, which confirms the presence of the elements in the coating. The composition in terms of weight percentage of Ni and P has been given in Table 3.

There has been no significant variation in the content of the deposits, due to heat treatment.
In as-deposited condition, electroless Ni-P coating is nanocrystalline in nature, having short range order. The phase structure depends on the phosphorus content. A higher percentage of phosphorus leads to the amorphous nature of deposits. The phase structure also depends on the heat treatment temperature. Phase transformation generally starts from 260 °C onwards [39]. The optimal hardness of Ni-P coating has been obtained at a heat treatment temperature of 400OC (for 1h), due to the crystallization of nickel and precipitation of crystalline nickel phosphides [4]. Similar results have been obtained in the present study from X- ray diffraction (XRD) analysis of the as-deposited and heat treated coatings (Fig. 4).
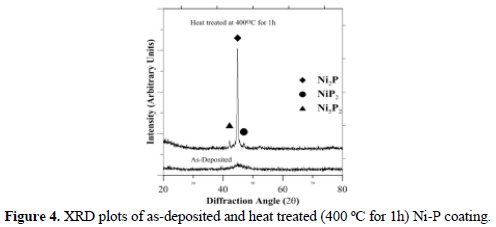
The as-deposited coating is seen to be microcrystalline in nature, while it changes to crystalline in nature due to the formation of Ni2P, NiP2 and Ni5P2. This leads to a higher hardness value of the heat treated coatings (∼45HRc).
Regression modeling
The wear tests to obtain the data for modeling of wear are done according to Taguchi's L27 OA. The combination of test parameters along with the obtained wear depth (μm) has been given in Table 4.
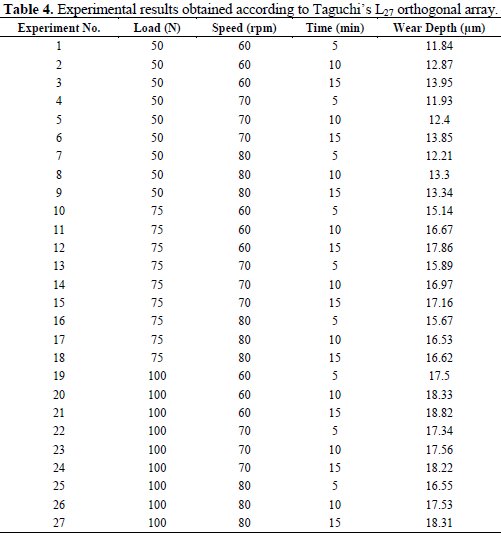
The second order regression model was fitted from the obtained data using Minitab software [40]. The obtained equation in an un-coded form of the design variables is:

A plot of the experimental results along with the regression predicted results has been given in Fig. 5.
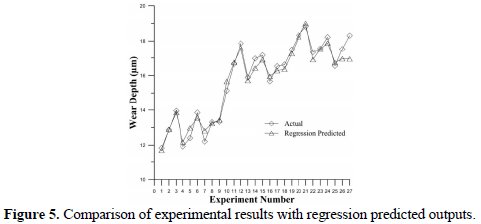
From the plot it can be deduced that the regression model is quite efficient in predicting the response with high accuracy. To evaluate the predicting capability several performance measures can be used. In this study, mean square error (MSE), mean percentage error (MPE) and coefficient of determination (R2) has been used and they can be expressed as follows:

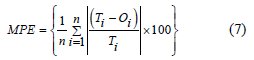
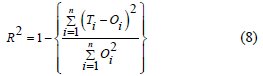
where n is the total number of results, Ti is the ith targeted data and Oi the observed value.
In the present work, the MSE, MPE and R2 value of the regression model comes out to be 0.1655, 1.95411 and 0.9935, respectively. From the performance measures and Fig. 5 it can be concluded that there is a close correlation between the actual and predicted results, and a second order model can predict the complex relationship between the process parameters and wear with minimal error.
Fuzzy modeling
The basis of a fuzzy model is linguistic variables, i.e. words instead of numbers and fuzzy sets. Hence, the preliminary step is to fuzzify the input parameters, i.e. load, speed and time. In the present study, the fuzzy modeling has been done using MATLAB 7 (Fuzzy Toolbox). For fuzzification of the inputs, triangular membership functions have been chosen because of its simplicity in modeling. The input space has been divided into three fuzzy subsets, i.e. low (L), medium (M) and high (H). To obtain a fuzzy value of the output (wear depth), it has been divided into nine fuzzy subsets, i.e. extremely low (EL), very low (VL), low (L), low medium (LM), medium (M), high medium (HM), high (H), very high (VH) and extremely/ high (EH/). Again, to map the output onto a fuzzy subset, triangular membership functions have been chosen. The membership functions for load, speed, time and wear depth have been depicted in Fig. 6.
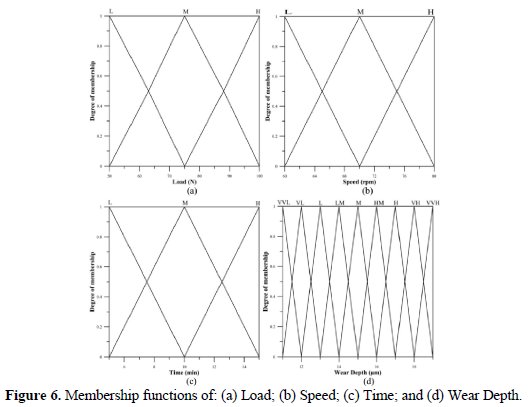
To relate the wear phenomenon with the process parameters, 27 rules are obtained directly from the combination of the parameters in L27 OA. The IFTHEN rule base along with the output has been enlisted in Table 5.
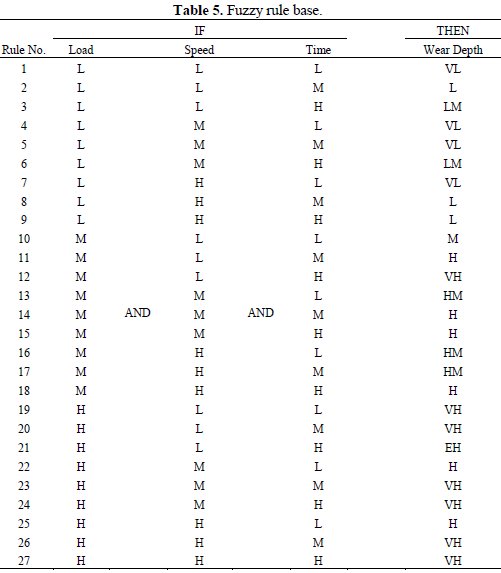
When a set of input is received by the fuzzy inference engine, it fires a certain number of rules, and a fuzzy output is obtained by using Mamdani's max-min implication. The fuzzy output is defuzzified using the centroid defuzzification method (Eqn. 4). A three input single output inference engine has been depicted in Fig. 7.
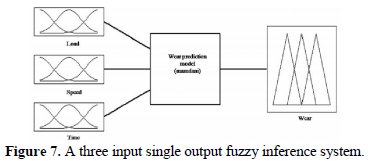
The experimental results have been compared with the ones that have been obtained from the fuzzy prediction model in Fig. 8.
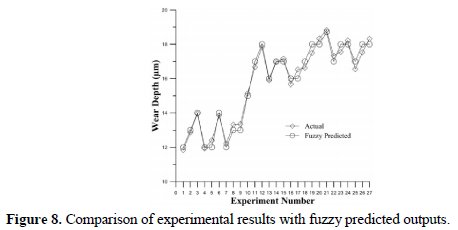
A close correlation between the experimental and predicted results is indicated. The MSE, MPE and R2 values of the fuzzy wear prediction model comes out to be 0.090867, 1.67849 and 0.99964 respectively, which indicate the high accuracy of the developed model. The performance measures of the regression and the fuzzy model have been compared in Table 6.

Results corroborate that both are capable of predicting the wear depth accurately, but the precision obtained from the fuzzy model is higher. Hence, fuzzy logic is seen to be more efficient than regression modelling to relate the highly non-linear behaviour of wear with the varying tribo-testing parameters.
Validation test
To check the adequacy of the developed second order regression and fuzzy rule based models, validation tests have been carried out. They are carried out with three different input values of load, speed and time, and the experimental, fuzzy predicted and regression predicted results are compared. The values of load, speed and time considered for the validation tests are selected from within the range of the parameters considered (L = 50N, S = 60 rpm, T = 9 min; L=75 N, S = 80 rpm, T = 12 min; L = 100 N, S = 70 rpm, T = 7 min). The fuzzy reasoning procedure for the parametric combinations of the second validation test has been shown in Fig. 9.
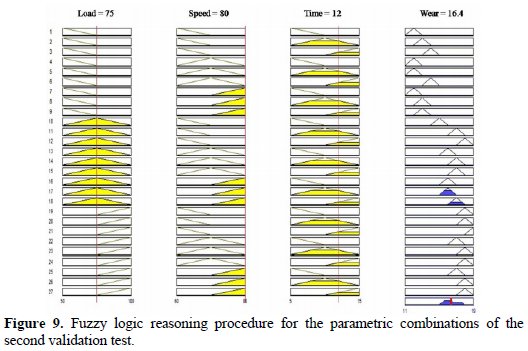
When the input values for load, speed and time are received by the inference engine, rules 17 and 18 are invoked (Table 5). The height of the darkened area represented in each triangle corresponds to the fuzzy membership value for that fuzzy set. Finally, a crisp output is obtained from the centroid of the combined darkened areas, which is shown at the bottom of Fig. 9, in the column for wear.
Fig. 10 shows the verification test results, and it can be inferred that both the models are capable of predicting the values of wear depth with high accuracy.
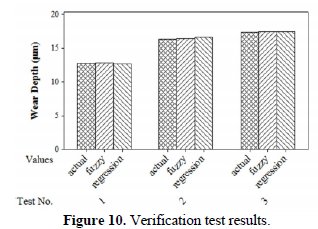
However, the precision obtained from the fuzzy logic model is higher, and hence it can be used effectively for the wear prediction of electroless Ni-P coating under lubricated condition.
Effect of process parameters on response variable
Surface plots revealing the trends in variation of wear depth of electroless Ni-P coating considering the interaction effect of the parameters are generated from the fuzzy logic model. The variation of wear depth with load, speed and time can be seen in Fig. 11.
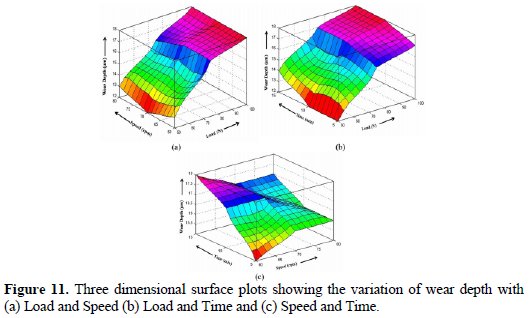
At higher values of load and speed, a higher wear of the electroless deposits is encountered (Fig. 11(a)). Similar trends are observed for the variation of wear depth with load and time, as well speed and time in Fig. 11(b) and Fig. 11(c), respectively, under lubricated condition. When the applied normal load is increased, the interacting surfaces advance more towards each other. This leads to an increase in the contact area of the asperities and consequently, a greater volume of the coating is sheared. With an increase in sliding speed or sliding time, sliding distance increases and, again, wear depth increases. Thus, a combination of the design parameters also leads to an increased value of the deposits wear.
Analysis of variance (ANOVA)
To ascertain the significance of the tribo-testing parameters and their interactions in controlling the wear of electroless Ni-P coating under lubricated condition, analysis of variance (ANOVA) has been carried out on the experimental results and given in Table 7.
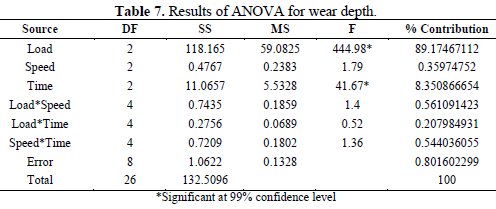
The last column of the table indicates the percentage contribution of each of the parameters and their interactions. The F-ratio obtained from Fisher's F-test [41] also gives a measure of the significance of any parameter on the response variable. At any confidence level α, if the calculated F-ratio for any factor is higher than the tabulated value, then it can be considered to be a significant one. In this study, it has been performed using MINITAB software [40]. Results reveal that the applied normal load has the highest significance followed by test duration. The interaction terms have negligible effect in controlling the wear of the coating.
Analysis of wear mechanism
SEM micrograph of a worn surface has been shown in Fig. 12.
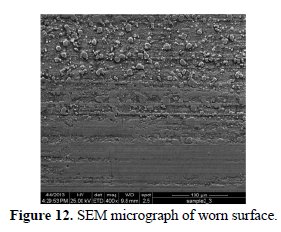
Longitudinal grooves with high degree of plasticity along the sliding direction can be observed. The surface is also characterized by micro-cutting and micro- ploughing effect. No pits or prows are observed. When a hard counterface material such as the one considered in the present study (hard chrome coated steel roller) moves through a softer surface and deforms it, mainly shear stresses are formed [42]. When this shear strength exceeds the shear strength of the material, a crack is formed which propagates, resulting in the fracture and detachment of the material, and formation of wear debris. Thus, material detachment takes place by abrasion, as well as fracture. Due to the heat generated during the sliding action, it is possible that the wear debris might get attached to the coated specimen. The flowing lubricant carries along with it the heat generated, as well as the wear debris formed, preventing adhesion or stick slip condition. Tribochemical wear may take place, if the used lubricant is corrosive in nature. However, the lubricant considered in the present study is engine oil, having a non-corrosive nature, and does not react chemically with the interacting surfaces. Hence, the possibility of tribochemical wear is eliminated. Thus, it can be noted that abrasive wear is the predominant wear mechanism.
The EDX spectrum of the worn surface has been illustrated in Fig. 13.
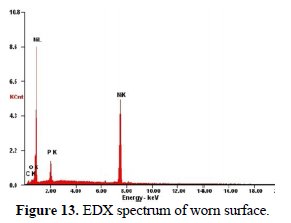
The presence of oxygen may be due to the formation of a thin microfilm (oxide layer) on the hard coating or only at the asperity tips of the coating [43]. Such type of tribo-layers formed on wear surfaces prove to be beneficial and result in a low coefficient of coatings friction. The effect of the oxide film on the deposits friction coefficient can be investigated further. The formation of a low shear micro-film onto the coating surface also leads to lower wear values. The presence of carbon is mainly due to the adsorbed lubricant during the tribological tests.
Conclusions
In the present study, electroless Ni-P coating is deposited on mild steel substrates and coating characterization is done using SEM, EDX and XRD. From the morphology and microstructure study, it can be seen that as-plated electroless Ni- P coating has nodulated structures, the size of which increases and becomes dense on heat treatment. The surface morphology is responsible for the self lubricating morphology of EN coatings. EDX spectrums confirm the presence of Ni and P in the coatings. XRD plots reveal that as-deposited Ni-P coating is microcrystalline in nature and changes to crystalline structure on heat treatment, due to the precipitation of phosphides. This results in enhanced deposits hardness and wear resistance.
Wear tests are carried out on a block-on-roller configuration multi-tribotester under lubricated condition. A second order regression and a fuzzy rule based expert system has been proposed to analyze and model the complex interrelationship between wear depth and the tribo-testing parameters (load, speed and time) considered in this article. The regression predicted and fuzzy predicted values are fairly close to the experimental values. However, various performance measures and validation tests indicate that the fuzzy-rule based model is better than the second order regression model for the prediction of wear depth.
Three dimensional surface plots reveal the variation of wear depth considering the interaction effects of the process parameters. It is seen that with the increase in load and speed, load and time as well as speed and time, the wear depth increases. The results of ANOVA performed on the experimental values obtained for wear depth of electroless Ni-P coating indicate that load and time are the most significant parameters in controlling the wear of the deposits at 99% confidence level. The wear mechanism as indicated by the SEM micrograph of a worn out specimen was seen to be abrasive in nature. EDX spectrum indicates the formation of a tribo-film on the coating surface.
Hence, fuzzy logic reasoning is successfully applied to predict the wear behavior of electroless Ni-P coating. The proposed fuzzy model can prove to be beneficial for extracting the optimum tribological performance out of the deposits under lubricated condition, and can also be utilized for online condition monitoring. The present method can further be extended to predict friction behavior of the deposits under lubricated condition and the behavior of electroless nickel composite and ternary coatings.
References
1. Brenner A, Riddell G E. J Res NBS. 1946;37:31. [ Links ]
2. Das S K, Sahoo P. Mater Design. 2011;32:2228. [ Links ]
3. Agarwala R C, Agarwala V. Sadhana, 2003;28:475. [ Links ]
4. Sahoo P, Das S K. Mater Design. 2011;32:1760. [ Links ]
5. Sudagar J, Lian J, Sha W. J Alloys Comp. 2013;571:183. [ Links ]
6. Apachitei I, Duszczyk J. Surf Coat Tech. 2000;132:89. [ Links ]
7. Apachitei I, Tichelaar F D, Duszczyk J, et al. Surf Coat Tech. 2002;149:263. [ Links ]
8. Keong K G, Sha W, Malinov S. Surface and Coatings Technology, 2003;168:263. [ Links ]
9. Guo Z, Keong K G, Sha W. J Alloys Comp. 2003;358:1129. [ Links ]
10. Yan M, Ying H G, Ma TY. Surf Coat Tech. 2008;202:5909. [ Links ]
11. Sahoo P. Mater Design. 2009;30:1341. [ Links ]
12. Sahoo P, Pal S K. Tribology Lett. 2007;28:191. [ Links ]
13. Taheri R, Oguocha I N A, Wear. 2001;249:389. [ Links ]
14. Palaniappa M, Seshadri S K. Wear. 2008;265:735. [ Links ]
15. Chen X M, Li G Y, Lian J S. Trans Nonferrous Metals Soc China. 2008;18:s323. [ Links ]
16. Roy S, Sahoo P. ISRN Tribology. 2012;Article ID 136740. [ Links ]
17. Roy S, Sahoo P. Procedia Tech. 2014;14:320. [ Links ]
18. Xu Y, Zheng X, Hu X, et al. Surf Coat Tech. 2014;258:790. [ Links ]
19. Wu Y, Liu H, Shen B, et al. Tribology Int. 2006;39:553. [ Links ]
20. Zhao Q, Liu Y, Müller-Steinhagen H, et al. Surf Coat Tech. 2002;155:279.
21. Moonir-Vaghefi SM, Saatchi A, Hedjazi J. Zeitschrift Metallkunde. 1997;88:498. [ Links ]
22. Li Z, Wang J, Lu J, et al. Appl Surf Sci. 2013;264:516. [ Links ]
23. Alirezaei S, Monirvaghefi S M, Salehi M, et al. Wear. 2007;262:978. [ Links ]
24. Sharma A, Singh A K. J Mater Eng Performance. 2013;22:176. [ Links ]
25. Gadhari P, Sahoo P. Procedia Mater Sci. 2014;6:623. [ Links ]
26. Jappes J W, Ramamoorthy B, Nair P K. J Mater Process Tech. 2009;209:1004. [ Links ]
27. Ramalho A, Miranda J C. Wear. 2007;263:592. [ Links ]
28. Duari S, Barman T K, Sahoo P. Procedia Mater Sci. 2014;5:978. [ Links ]
29. Zadeh LA. Information Control. 1965;8:338. [ Links ]
30. Ozek C, Caydas U, Unal E. Mater Manufacturing Proc. 2012;27:95. [ Links ]
31. Rajasekaran T, Palanikumar K, Vinayagam B K. J Comp Mater. 2012;46:809. [ Links ]
32. Chen J C, Savage M. Int J Adv Manuf Tech. 2001;17:670. [ Links ]
33. Ramesh S, Karunamoorthy L, Palanikumar K. Mater Manuf Proc. 2008;23:439. [ Links ]
34. Latha B, Senthilkumar V S. Mater Manuf Proc. 2009;24:509. [ Links ]
35. Chatterjee D, Sutradhar G, Oraon B. J Mater Proc Tech. 2008;200:212. [ Links ]
36. Montgomery D C. Design and analysis of experiments. John Wiley Sons; 2008. [ Links ]
37. Krishnamoorthy A, Boopathy S R, Palanikumar K, et al. Measurement. 2012;45:1286. [ Links ]
38. Ajibola O O, Oloruntoba D T, Adewuyi B O. J Coat. 2014;2014: Article ID 841619.
39. Krishnan K H, John S, Srinivasan K N, et al. Metallurgical Mater Trans A. 2006;37:1917. [ Links ]
40. Minitab User Manual (Release 13.2). MINITAB. PA, USA: Inc. State College; 2001. [ Links ]
41. Fisher R A. Statistical methods for research workers. Genesis Publishing Pvt Ltd; 1925. [ Links ]
42. Holmberg K, Ronkainen H, Laukkanen A, et al. Surf Coat Tech. 2007;202:1034. [ Links ]
43. Holmberg K, Ronkainen H, Matthews A. Ceramics Int. 2000;26:787. [ Links ]
*Corresponding author. E-mail address: psahoo@mech.jdvu.ac.in
Received 13 January 2016; accepted 19 February 2016