Serviços Personalizados
Journal
Artigo
Indicadores
-
Citado por SciELO
-
Acessos
Links relacionados
-
Similares em SciELO
Compartilhar
Portugaliae Electrochimica Acta
versão impressa ISSN 0872-1904
Port. Electrochim. Acta vol.34 no.4 Coimbra jul. 2016
https://doi.org/10.4152/pea.201604231
Investigation of Wear Behavior of Electroless Ni-P-W Coating under Dry and Lubricated Conditions Using RSM and Fuzzy Logic
Arkadeb Mukhopadhyay , Santanu Duari , Tapan Kr. Barman and Prasanta Sahoo*
Department of Mechanical Engineering, Jadavpur University, Kolkata 700032, India1
Abstract
The present work aims to investigate and correlate the wear behavior of electroless Ni- P-W coating under dry and lubricated conditions with the tribological testing parameters. A pin -on -disc configuration test setup is used for the same. Taguchi's orthogonal design of experiments technique is used to carry out the experiments. Both response surface and fuzzy rule based models are seen to be effective in determining the complex interrelationship between the wear depth of the coatings and the test parameters, namely applied normal load, sliding speed and sliding duration. The coefficient of determination for fuzzy logic based predictions is seen to be higher than the regression predicted ones, indicating better modeling capabilities of the artificial intelligence technique. ANOVA results reveal that the wear depth is mostly influenced by sliding speed followed by applied normal load and sliding duration for both dry and lubricated conditions. Coating composition, phase transformation and microstructure studies are undertaken to analyze their effects on the wear behavior. Abrasive wear mechanism is seen to be the predominating under both dry and lubricated condition. The coatings are seen to suffer more wear under dry sliding condition compared to lubricated environment.
Keywords: Electroless Ni-P-W coating; wear; response surface methodology; fuzzy logic; lubricated.
Introduction
Prevention of wear and corrosion of tribological contact by the application of surface coatings has received significant attention and has experienced a rapid evolution in the last few decades. Electroless nickel (EN) coating has proven to be a potential candidate for the same [1-4]. EN coatings possess excellent wear and corrosion resistance, high hardness and low coefficient of friction (COF), which make them suitable for use in several industries such as marine, aerospace, automobile, chemical, electrical, food processing, etc [2]. EN coatings can be categorized as pure nickel, nickel alloy and composite coatings. The deposition of pure nickel (99%) from hydrazine bath has found little industrial usage due to the hazards and cost associated with it [1]. At higher temperatures, hydrazine baths tend to become unstable and difficult to control. Sodium hypophosphite reduced electroless Ni-P alloy coating finds 90% share in industrial applications [1]. On using sodium borohydride as the reducing agent, a Ni-B alloy coating is obtained, which possesses even higher hardness and wear resistance comparable to that of chromium [2].
Incorporation of hard particles and solid lubricants in the Ni-P matrix such as diamond, Al2O3, TiO2, PTFE, SiC, graphite, etc. has been reported in several research works to improve the tribological performance of the coatings under dry condition [5-9]. The Ni-P binary alloy coating has been explored by several researchers, and it is seen that friction, wear and corrosion resistance properties can be easily tailored by controlling the coating bath parameters and proper heat treatment [10-12]. The deposit uniformity and the wide variety of substrates that can be plated by this method are the added advantages [1-4]. The friction and wear behavior of EN coatings under lubricated condition has been reported in several research works [13-18]. The tribological behavior of borohydride reduced Ni-B alloy coatings under oil lubrication has also been addressed [19-21]. The results obtained from the studies of tribological behavior of Ni-P, Ni-P-PTFE, Ni-P-Cu, Ni-P-CNT and Ni-B coatings indicated that the deposits have tremendous friction and wear reduction capabilities under lubricated condition. In fact Ni-P-Cu coatings can effectively prevent engine cylinders from corrosive wear of bio-lubricants [16]. Electroless Ni-P-PTFE coating has proven to be suitable, especially under boundary lubrication condition [15]. A hybrid grey- fuzzy logic along with Taguchi's design of experiments has been employed by Mukhopadhyay et al. [19], to achieve optimal tribological behavior of Ni-B coating under oil lubricated condition.
The use of mathematical and artificial intelligence techniques for the analysis of a complex system leads to an improvement in product quality and a reduction in time, keeping in mind cost effectivity. The conventional regression analysis using orthogonal arrays and response surface methodology has proved to be quite simple and fruitful for effective modeling and prediction of EN plating [22-24]. Some instances of the use of artificial neural network (ANN) for the modeling of crystallization temperature, plating rate and phosphorus content have been reported [25-29]. Hardness prediction model for Ni-P coatings considering heat treatment temperature and its duration, as well as the phosphorus content has been elaborated by Vaghefi and Vaghefi [30]. Fuzzy logic is seen to be quite efficient in relating the complex wear phenomenon with the tribological test parameters for Ni-P coatings [13].
The introduction of a transition element such as W to the binary Ni-P alloy coating has received considerable attention due to the unique properties achieved, such as thermal stability, high hardness, wear resistance and corrosion resistance. Co-deposition of tungsten controls the crystallinity of Ni-P coatings by reducing the phosphorus content [31, 32]. However, the crystallization temperature is raised on account of tungsten addition. The formation of a Ni-W solid solution explains the high hardness and wear resistance achieved in addition to thermal stability [33, 34]. The thermal stability under long term aging treatment for electroless Ni-P-W coating has been investigated by Tien et al [35]. It is reported that, at 375°C, the coating is strengthened up to 1440 HK, due to the crystallization of nickel, precipitation of Ni3P and solid solution strengthening. The hardness remains unchanged even after 20h heat treatment. The hardness further increases to 1460 HK with heat treatment of the deposits at 450°C for 4h. The as-deposited coatings are seen to be amorphous/ nanocrystalline in nature, which depends primarily on the content of phosphorus of the deposits. The phase transformation behavior and thermal stability of Ni-P-W coating for various phosphorus and tungsten content has been investigated by Balaraju et al [36]. It is reported that the addition of W leads to a more nodular surface morphology, and metastable phases such as Ni5P12 and NiP are identified along with stable Ni3P and Ni for heat treated coatings at 400°C for 1h. Better thermal stability is obtained for baths containing 20g/L sodium tungstate. A correlation between the structure of electroless deposited Ni-P-W coating with the hardness and corrosion behavior has been established by Liu et al [37]. It is revealed that with heat treatment of the coatings at a temperature of 300°C or below, the increase in hardness is mainly due to the crystallization of nickel from the amorphous phase and not to Ni3P. Peak hardness is obtained for a heat treatment temperature of 400 or 500°C, which can be mainly attributed to the precipitation of Ni3P phase. The poorest corrosion resistance is obtained for the coatings heat treated at 400°C for 1h. Laser nano-crystallization affects the wear and corrosion resistance significantly compared to the furnace annealed ones [38]. The best corrosion resistance is achieved at lower scanning velocities [39, 40].
It has been used the design of experiments and Taguchi based Grey relational analysis for the optimization of tribological testing parameters for minimum friction and wear, while optimization of coating deposition parameters for improving corrosion resistance of electroless Ni-P-W coating has been carried out extensively by Roy and Sahoo [41, 42]. A parametric combination of 50N applied normal load, 70 rpm roller speed and 5min test duration has been reported to yield minimum friction and wear of the coatings on a block-on-roller multi-tribotester [41]. From electrochemical impedance spectroscopy study in 3.5% NaCl solution, the best corrosion resistance is seen for a coating bath composition having 30 g/L nickel sulphate, 17 g/L sodium hypophosphite, 25 g/L sodium tungstate and an annealing temperature of 600 °C [42]. Optimum deposition of tungsten and phosphorus content of the coatings using orthogonal arrays by considering plating bath parameters such as concentration of sodium hypophosphite, sodium citrate, bath temperature, pH and stabilizer has been reported by Shu et al. [43].
From an extensive review of literature, it seems that the research concerning electroless Ni-P-W coating is mainly directed towards the investigation of surface morphology, microstructure, corrosion resistance, microhardness, wear resistance and friction behavior. The effect of different heat and surface treatments on the same has also been studied, while the tribological behavior has been investigated mostly under dry sliding condition [31-43]. Investigation of tribological behavior of EN coatings under lubricated condition is seen to be mostly concentrated around Ni-P binary alloy coatings and its composite variants [13, 14-18]. EN coatings find extensive usage in the automotive industry [1-4]. The exploration of friction and wear behavior of Ni-P-W coating under oil lubricated condition can prove to be fruitful to extend the usage of the coatings to newer applications involving tribological contact under lubricated environments. Moreover, a scarcity of literature on the investigation and modeling of tribological behavior of Ni-P-W coatings using AI techniques is also seen. The use of fuzzy logics is extensively seen for the prediction and optimization of machining operations and manufacturing processes, due to its simplicity and effectivity in handling vague and imprecise data [44-47].The present study is thus intended towards the same in a systematic manner, using Taguchi's design of experiments, regression analysis and artificial intelligence (AI) technique. Hence, a fuzzy rule based expert system has been proposed in the present study along with the conventional regression method for the prediction of wear depth of electroless Ni-P-W coating under dry and lubricated condition. An expert fuzzy model can be effectively used for quicker decision making, in cases where a practical approach is not feasible.
In the present work, Ni-P-W coating is deposited on AISI 1040 steel specimens. The coating characteristics are studied using energy dispersive X-ray analysis, X- ray diffraction and scanning electron microscope. The wear tests are carried out under dry and lubricated sliding condition on a pin-on-disc tribological test setup. An effort has been made to analyze the relationship between the tribological test parameters and wear behavior of the coatings, using response surface methodology and fuzzy logics. A comparative analysis between the two modeling procedures has been undertaken. The mechanism governing the wear of Ni-P-W coating has been investigated both under dry and oil lubricated condition. The feasibility of the coatings for applications involving tribological contact under lubricated condition is ascertained.
Response surface methodology
Response surface methodology (RSM) is a simple yet powerful tool for the modeling and analysis of a response of interest that is influenced by several process variables. It combines both mathematical and statistical techniques to find out the interrelationships between the responses and process parameters, as well as to evaluate the competence of the model for future predictions [48-52]. Generally, a response surface is graphically represented. If the response of interest can be defined efficiently by a first order model, then the approximating function can be written as [52]:

where k is the number of independent variables, x1, x2, x3, ..., xk are the independent variables, β0, β1, β2, β3, ..., βk are constants, while η is the error term.
In cases where curvature exists in the system, a higher order polynomial is required to approximate the surface. Generally, a second order model is seen to be adequate in representing the same, and is given as [52]:

where β0 is a constant, βi, βii and βij are the coefficients of linear, quadratic and interaction terms, respectively. The fitted equation is obtained as:

Fuzzy reasoning method
Development of a mathematical model of a system response with the corresponding design variables has always interested researches. However, it is not always feasible to obtain an accurate mathematical model in several practical situations, due to the highly complex and vague interrelationships that exist between the system and the process variables. In such cases, fuzzy logic offers an efficient means of system design based on non-formally expressed laws that are derived from a database or expert knowledge. Fuzzy logic was firstly proposed by Zadeh [53], and the true essence of it lies in the use of linguistic variables. The relationship between a system response and the process variables can be captured in the form of rules which can be expressed as:
IF (antecedent) THEN (consequent)
Vague, uncertain and imprecise information can be well handled by the fuzzy logic method. The basis of fuzzy logic is the fuzzy set theory. In any ordinary set, an object is either included or excluded, meaning its membership value is either 1 or 0. But, in a fuzzy set, there is a gradual transition from membership to nonmembership [54]. A membership value between 0 and 1 is assigned to an object. A fuzzy logic system consists of a fuzzifier, fuzzy inference engine and a defuzzifier. A typical fuzzy process is shown in Fig. 1.
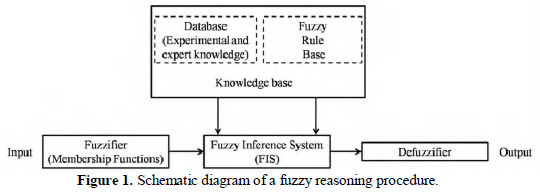
The crisp inputs are fuzzified, i.e., converted to linguistic expressions. The fuzzification is carried out using membership function (MF) that assigns membership values. The range of the input parameters is found out, and the whole space is divided into fuzzy subsets using MF. Values of MF range between 0 and 1, which determine the degree of membership of a variable in a subset. A MF can be triangular, trapezoidal, gaussian, bell shaped or sigmoidal. The fuzzified members then invoke the fuzzy inference engine (FIS), which comprises a knowledge base based on expert intuition and rule base. A certain number of rules are satisfied, which in turn give the fuzzified output by performing Mamdani's MAX-MIN implication or Takagi and Sugeno's approach [54]. Finally, a crisp output is obtained from the defuzzifier.
Experimental details
Coating deposition
Coating deposition is carried out on steel (AISI 1040) substrates of dimension Φ6 × 30 mm. The substrates are rinsed in deionized water and cleaned using acetone for degreasing and removal of corrosive products. A pickling treatment in 50% HCl is given to remove rust or oxide layers which may have formed. After thorough cleaning of the substrates, they are subjected to surface roughness measurements. EN coatings follow the substrate profile and, hence, they are selected with precision, to eliminate the effect of surface roughness on the tribological behavior of the coatings. The specimens corresponding to N6 grade (Ra = 0.8 μm) are selected for coating deposition. Prior to deposition in the electroless bath, the specimens are again rinsed in deionized water, and dipped in a warm (55°C) PdCl2 solution. This process, known as âactivation', is done to achieve a higher deposition rate, good adhesion of the coatings with the substrate, and obtain a significant amount of deposit thickness, so that tribological tests can be carried out [19, 55, 56]. It may be noted here that the substrates used in the present study are catalytically active. But it is observed that, without the activation in PdCl2, the deposition proceeds at a very slow rate, leading to a very low deposit thickness [56]. In the meantime, the electroless bath is prepared by mixing the chemicals in the selected order, as enlisted in Table 1.
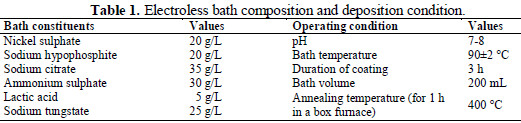
A bath volume of 200 ml is taken for coating deposition. The parameters of the electroless bath are kept constant to achieve equal coating thickness and properties for all the specimens. After a deposit period of 3 hrs, the coated specimens are heat treated in a muffle furnace at 400°C for 1 hr.
The coatings are allowed to cool to the ambient temperature inside the furnace itself. The deposition setup is illustrated in Fig. 2.
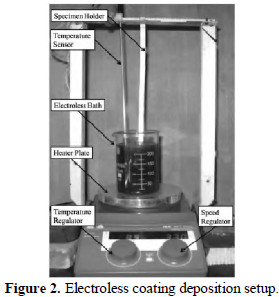
Coating characterization
The composition of EN coatings significantly determines the phase structure and tribological behavior. Heat treatment results in an improved hardness, wear resistance and low coefficient of friction. Therefore, it is necessary to study the coating characteristics in detail, to understand their effect on wear behavior. Energy dispersive X-ray (EDX) analysis (FEI, Quanta FEG 250) is used to determine the percentage by weight of nickel, phosphorus and tungsten in the coating. To analyze the phase transformation taking place due to heat treatment, X-ray diffraction (XRD) with Cu-Kα (Rigaku, Miniflex) technique is used. The surface morphology of the coating before and after annealing at 400°C for 1hr is observed under a scanning electron microscope (SEM) (JEOL, JSM 6360). The wear mechanism is also ascertained from SEM micrographs of worn out specimens after the tribological tests under dry and lubricated sliding condition.
Tribological tests
The dry and lubricated sliding wear tests are carried out on a pin-on-disc tribological test setup (Ducom, TR-20-LE-CHM-400, India). The coated pin specimens are held stationary against a rotating counterface disc (diameter 165mm, 8mm thick) of EN 31 material hardened to 60HRc and ground to 1.6Ra surface roughness. The wear track diameter is kept fixed at 80mm. The normal load is applied to the specimen by placing dead weights on a loading pan connected to a loading lever of ratio 1:1. The sliding speed and sliding duration can be controlled from the friction/wear monitor. Table 2 enlists the tribological test parameters along with their levels.
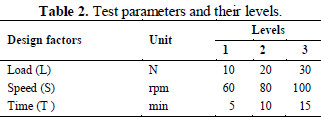
The parameters are selected after a meticulous study of the literature and carrying out several experiments. Wear depth is acquired by a LVDT (Syscon) with a least count of 1μm and 1±1% of measured wear. A commercially available engine oil (Servo PRIDE-4T, Indian Oil) is used for the wear tests under lubricated condition. Experiments are systematically carried out following the combinations available in Taguchi's L27 orthogonal array (OA). The L27 OA can accommodate the direct as well as the interaction effect of the test parameters on responses.
Wear depth is acquired by a LVDT (Syscon) with a least count of 1μm and 1±1% of measured wear. A commercially available engine oil (Servo PRIDE-4T, Indian Oil) is used for the wear tests under lubricated condition. Experiments are systematically carried out following the combinations available in Taguchi's L27 orthogonal array (OA). The L27 OA can accommodate the direct as well as the interaction effect of the test parameters on responses. The pin-on-disc tribotester is shown in Fig. 3.
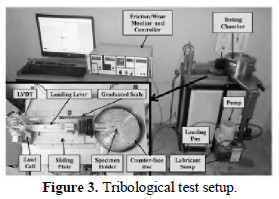
Results and discussion
Composition, phase transformation, microstructure and coating thickness
Composition of the coatings in terms of weight percentages of nickel, phosphorus and tungsten is obtained from EDX analysis which is 88%, 8% and 4%, respectively, in the as-deposited coatings. The EDX spectrum of the coatings in its as-deposited and heat treated condition is shown in Fig. 4.
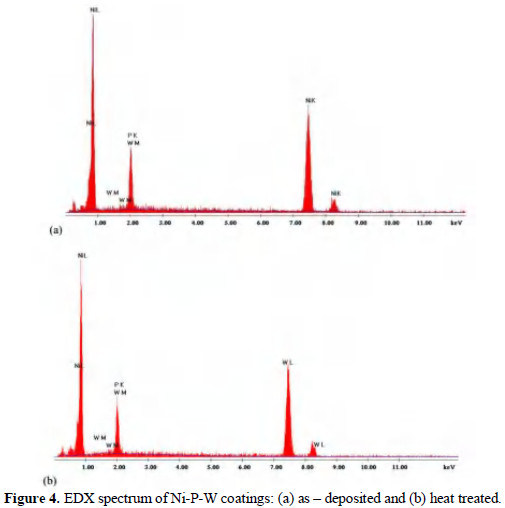
Phosphorus percentage of the deposits determines their crystallinity. In general, for the binary Ni-P alloy system, the coatings are seen to be amorphous in the high phosphorus (10-13 wt% P) range, while there is a mixture of nanocrystalline and amorphous phases in the medium phosphorus (6-9 wt% P) range. The incorporation of tungsten to Ni-P coating leads to a decrease in the phosphorus content. As the concentration of sodium tungstate is increased in the electroless bath, the phosphorus content decreases, while the tungsten content increases [32], and the coatings are transformed more to a mixture of amorphous and nanocrystalline phases. This also causes an improvement in the microhardness of the deposits, which in turn affects the tribological behavior. The phosphorus content obtained in the present case is around 8% by weight, which corresponds to the medium phosphorus range, and it is expected that the coatings would exhibit an X-ray amorphous/nanocrystalline nature in its as-deposited condition. The phase transformation caused on account of the heat treatment is analyzed using XRD, and shown in Fig. 5.
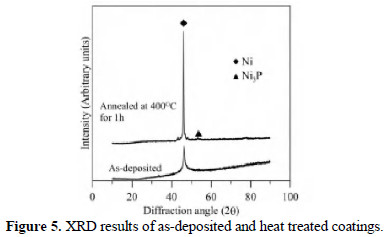
Results obtained in the EDX analysis are further supported by the XRD results where as-deposited Ni-P-W coating exhibits amorphous/ nanocrystalline behavior with a sharp peak pertaining to Ni (111) plane surrounded by short peaks. The intensity corresponding to Ni (111) increases on heat treatment at 400°C for 1 hour, and the coatings turn crystalline due to the precipitation of stable crystalline phases of nickel phosphides (Ni3P). Heat treatment improves the microhardness and wear resistance of Ni-P-W coatings. This is mainly attributed to precipitation hardening, as well as to a solid solution strengthening phenomenon [33].
SEM micrographs of an as-deposited and heat treated specimen are shown in Fig. 6(a) and Fig. 6(b), respectively.
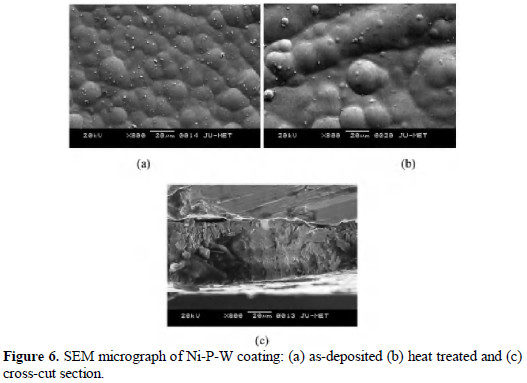
A nodular surface morphology similar to that of a cauliflower can be observed in Fig. 6(a) for the as-deposited coating. The coating deposit is quite homogenous without any porosity. Bright particles are visible to be scattered all over the coating surface. This can be attributed to the excess growth of nickel alloy over the nodules. Similar results were obtained by Selvi et al. [57], and it was explained that this excess growth could be due to the mixed complex in the electroless bath which acts as a precursor for the deposition of the alloy. On heat treatment the nodules are seen to become more compact, and some amount of volume contraction is observed in Fig. 6(b). Phase transformation of Ni-P-W coating results in a large number of Ni3P crystallites precipitating out of the supersaturated solid solution of nickel, thereby causing volume contraction [40]. A cross-cut section of the coated specimen is shown in Fig. 6(c). The coating thickness is seen to be around 30 μm and quite uniform, which is a characteristic feature of EN coatings.
Analysis of response surface model
The present investigation considers applied normal load, sliding speed and sliding duration as the independent variables, while the wear depth is the system response. The experimental combinations of the independent regressor variables (process parameters) in their un-coded and coded form, along with the system response, i.e., wear depth under dry and lubricated conditions, are given in Table 3.
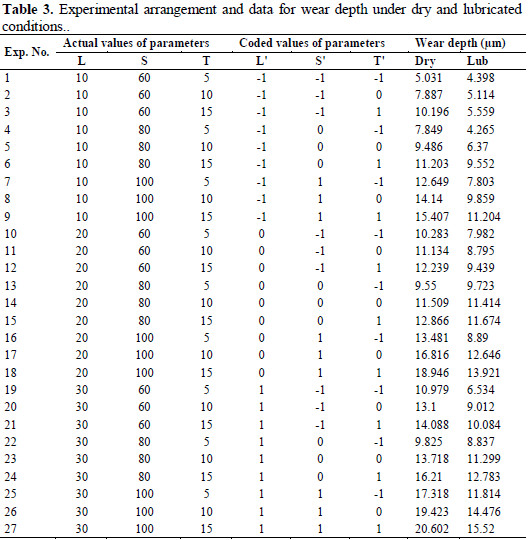
The regression constants are evaluated by using a method of least squares, and it is carried out using Minitab [58]. The fitted second order equation for wear depth under dry condition in un-coded form is:

Similarly, the wear depth under lubricated condition can be expressed in uncoded form by the following:

The analysis of the response surface model is carried out using Minitab software [58] for wear depth under dry and lubricated condition. The significance of the second order regression model is evaluated by calculating the coefficient of determination (R2). The coefficient of determination signifies the percentage of variability that can be accounted for by the model. The capability to predict the response with accuracy is denoted by the term R2 [48-51].
Moreover, the correlation between the predicted and experimental wear depth for both dry and lubricated conditions is shown in Fig. 7(a) and Fig. 7(b), respectively.
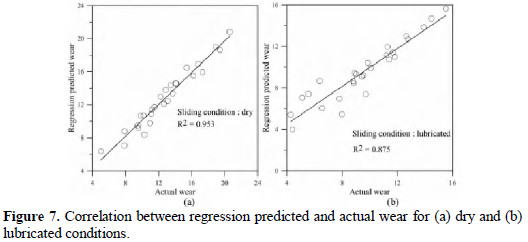
A high correlation between the two is observed in Fig. 7, which indicates that the model is quite significant, and can be used for further predictions. Furthermore, the R2 value is seen to be 95.3% and 87.5% for dry and lubricated condition, respectively, which is reasonably high. This indicates the sufficient closeness of the predicted response to the actual response. Hence, it can be assimilated that the quadratic models for wear depth under dry and lubricated condition can suitably conceive the complex relationship with the tribological test parameters.
Similarly, the ANOVA results for wear depth under lubricated condition are presented in Table 3. Again, the linear terms are seen to be important, along with the square term of load.
The percentage contributions of the process parameters, their square terms and interactions are also given in Tables 4 and 5, for dry and lubricated condition, respectively.
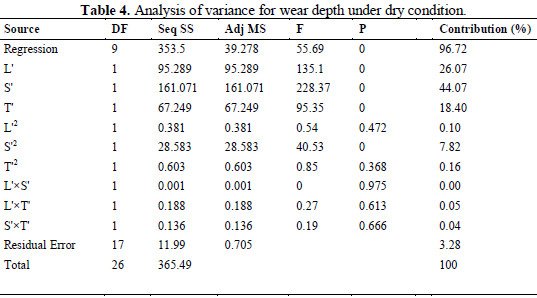
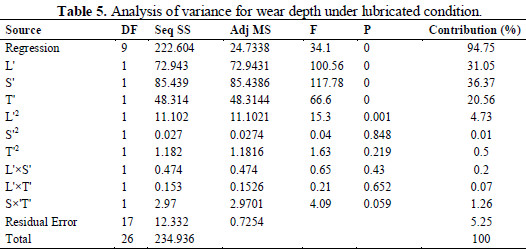
Accordingly, it can be noticed that sliding speed has the highest contribution followed by applied normal load and sliding duration for both dry and lubricated condition.
Statistical significance of the developed models is analyzed by carrying out analysis of variance (ANOVA) using Minitab [58], and in coded form of the parameters. The quadratic models expressed in Eqn. 4 and Eqn. 5 have been developed at 95% confidence interval. In that case, a P value lower than 0.05 indicates a significant contribution by a factor, square term or an interaction term. ANOVA results for wear depth under dry condition are given in Table 4.
From the results, it can be assessed that the regression model is highly significant. All the three linear terms, i.e., load, speed and time are significant along with the square term of speed.
Analysis of fuzzy rule based model
In the present work, the input (load, speed and time) as well as the output parameter (wear depth) are fuzzified using triangular membership function. To represent the input parameters, linguistic variables such as low (L), medium (M) and high (H) are used [13]. The fuzzy subsets for the same are shown in Fig. 8.
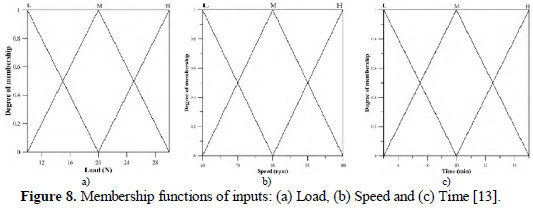
To obtain a fuzzy value of the output (wear depth), it has been divided into nine fuzzy subsets, i.e., very very low (VVL), very low (VL), low (L), low medium (LM), medium (M), high medium (HM), high (H), very high (VH) and very very high (VVH) [13]. Again, to map the output onto a fuzzy subset, triangular membership functions (MF) have been chosen. The MF for wear depth under dry and lubricated condition has been shown in Fig. 9(a) and Fig. 9(b), respectively. Triangular MF is chosen for its simplicity of use.
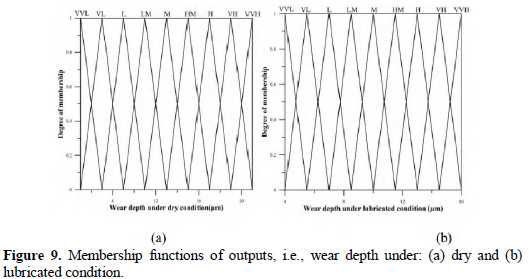
After fuzzification of the input and the output space using linguistic variables, they are correlated by a IF-THEN fuzzy rule based system, which forms the core of the FIS. Two separate FIS are created for modeling the wear depth under dry and lubricated condition. From the number of process parameters and their levels, 27 rules are directly obtained which again depend on the experimental values of the responses. Table 6 enlists the rule base derived from the experimental results for both dry and lubricated sliding wear.
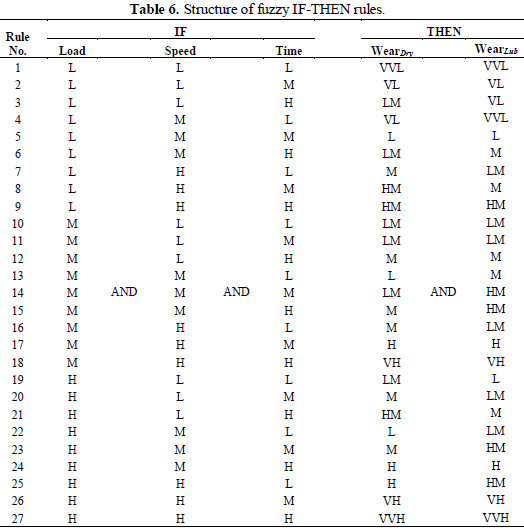
Using Mamdani's max-min implication, a fuzzy subset is assigned to each output variable for a particular rule. Finally, the centroid defuzzification scheme is used in the present investigation to obtain a crisp value of the output. The centroid method computes the centroid of the area under the membership function.
The correlation graph for fuzzy rule based predicted wear depth under dry and lubricated condition is illustrated in Fig. 10(a) and Fig. 10(b), respectively.
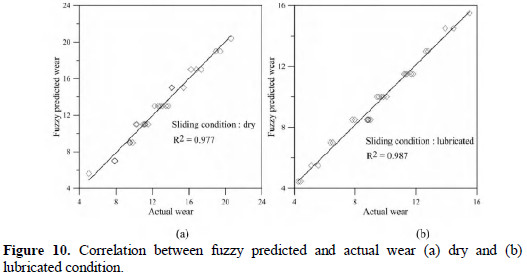
The R2 value for the fuzzy prediction model is seen to be 97.7% and 98.7% for dry and lubricated sliding condition, respectively. Therefore, within the experimental domain, fuzzy model is seen to be adequate in interpreting the relationship of the complex wear phenomenon and the process parameters viz. load, speed and time. The closeness of the points to the straight line in Fig. 10 clearly indicates the close correlation that exists between the predicted and experimentally obtained values. In fact, the fuzzy model is seen to be superior compared to the response surface method in precisely relating vague and uncertain of the data by the use of linguistic variables and an expert system. Thus, it can be concluded that both regression and fuzzy model are valid and acceptable, but fuzzy system has better modeling capabilities, and in a more human like manner.
Validation tests
To validate the observations made from RSM and fuzzy models, two test runs T1 and T2 are undertaken at dry and lubricated condition. T1 corresponds to a parametric combination, where the applied normal load is 15N, a sliding speed of 70 rpm and a sliding duration of 9 min. The combination of test parameters corresponding to T2 is applied normal load of 25N, sliding speed of 90 rpm and 12 min sliding duration. Intermediate values of tribo-testing parameters within the experimental domain are purposely selected to check the adequacy and correlation of the developed models with the actual (experimental) results obtained. The validation test run results for dry and lubricated sliding condition are presented in Fig. 11(a) and Fig. 11(b), respectively.
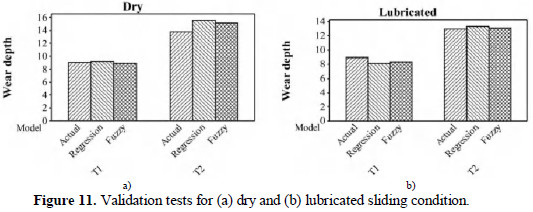
It can be clearly seen that both second order regression and fuzzy models have excellent prediction capabilities, and corroborate well with the actual results experimentally obtained.
However, the results obtained from fuzzy model exhibit less errors compared to the regression predicted results. Therefore, the validation tests also substantiate the observations made in the present experiment, where fuzzy rule based expert system is seen to have a higher R2 value compared to RSM for both dry and lubricated tribological test conditions. Similar results were obtained by Mukhopadhyay et al. [13], where fuzzy logic and conventional regression method were used to analyze the wear behavior of electroless Ni-P coating under lubricated condition.
Effect of process parameters on wear
The trends in variation of wear depth with the interaction effect of the process parameters are determined from surface plots predicted by the fuzzy model both under dry and lubricated conditions. The plots for dry sliding wear behavior are depicted in Fig. 12.
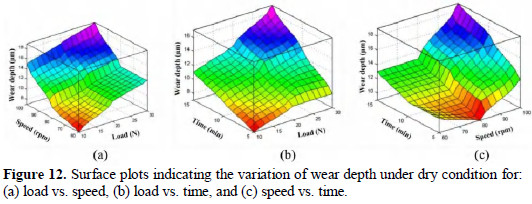
For load vs. speed interaction (Fig. 12(a)), the wear depth is seen to increase with an increase in load and speed. In general, with an increase in the applied normal load, the actual contact area of the asperities increases, because of the advancement of the mating surfaces towards each other. Due to this, the sheared volume of the coatings by the hard counterface material increases, indicating an increase in the wear depth in the present case. Similarly, an increasing trend in wear depth is observed for load vs. time interaction (Fig. 12(b)). It is also to be noted that, as the sliding speed or the sliding duration increases, a simultaneous increase in sliding distance is achieved. This again leads to more wear of the coatings, unless tribochemical reaction at the sliding interface occurs, and a protective oxide film formation takes place at the tip of the asperities. Due to the aforesaid reasons, the wear depth is again seen to increase with speed vs. time interaction (Fig. 12(c)).
However, in Fig. 12(a) and Fig. 12(b), the wear depth encountered is seen to be quite low up to 80 rpm. This could be due to the rolling effect of worn particles of the debris being entrapped between the counterface and coating. This effect again vanishes at a higher level of the test parameters, and the wear debris seems to contribute to the ploughing effect, causing higher wear.
The obtained trends are also seen to be in accordance with Archard's relation for wear according to which, the wear volume is directly proportional to the applied normal load and sliding distance, and inversely proportional to the hardness of the material.
To assess the wear behavior of electroless Ni-P-W coating with interaction effects of the test parameters under lubricated condition, 3D surface plots are again generated from the fuzzy prediction model and are displayed in Fig. 13.
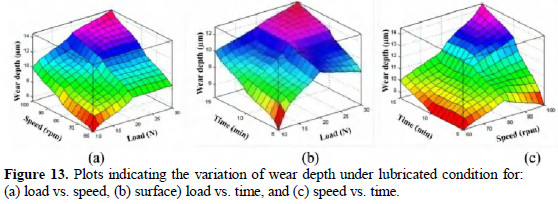
For load vs. speed interaction (Fig. 13(a)), the wear depth is seen to increase up to 20N, and then gradually stabilizes. No steep increase in wear depth is observed. Initially higher wear may be encountered due to the running in stage. The wear depth stabilizes at a later stage. Moreover, as the speed increases, the adsorbed film thickness increases leading to better lubrication. However, it can be observed in Fig. 13(a) that, at higher values of the parameters, a higher wear depth is encountered, which may be due to the breakage of the lubricant film at higher load. Similar result is obtained in Fig. 13(b) for the load vs. time interaction. The wear depth tends to stabilize after 20 N applied normal, and up to a sliding duration of 10 min, after which the response seems to increase. In Fig. 13(c), for speed vs. time interaction, the wear depth is again seen to be stable at higher speed, up to 10 min sliding duration, above which it increases. Thus, in Fig. 13, for all the interaction effects of the tribo-test parameters, the wear depth is seen to be higher at higher levels of the same. But, on comparing with the results obtained in dry condition (Fig. 12), a clear enhancement in wear behavior is seen under lubricated condition. Another reason for the low wear of the coatings under lubricated condition can be due to the fact that the lubricant carries away the generated heat, and lowers the flash temperature at the contact point of the asperities. Therefore, electroless Ni-P-W coatings are found to be suitable for reduction of wear of tribological contact applications working under lubrication.
The wear mechanism of electroless Ni-P-W coating after dry sliding against hardened EN 31 counterface disc is analyzed by observing the worn specimens under SEM. Under dry sliding condition, most of the specimens exhibit similar wear morphology. Hence, the wear track at the highest parametric combination, i.e., 30N applied normal load, 100 rpm sliding speed and 15 min sliding duration is shown in Fig. 14.
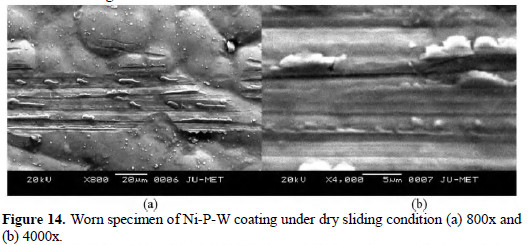
Micro cutting and micro ploughing in the direction of sliding arte observed (Fig. 14(a)). A three body abrasion is clearly indicated with the debris particles cutting through the coating and displacing the material to the side of the wear track (Fig. 14(b)). Therefore, abrasive wear mechanism of the coatings is established from the wear track images. Such abrasion leads to an increase in the wear depth, with an increase in the test parameters, and the same is reflected in Fig. 14.
Similarly, the wear mechanism is analyzed for lubricated sliding condition, and the SEM micrograph is shown for 10 N applied normal load, 100 rpm sliding speed and 15 min sliding duration in Fig. 15.
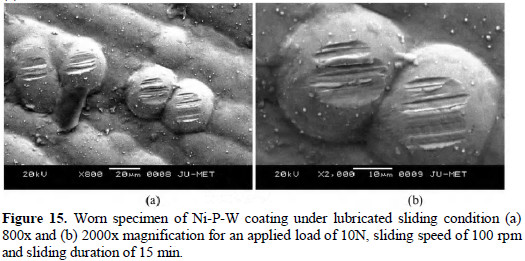
It can be noted here that worn specimen for 10 N and 20 N load and other combination of sliding speed and sliding duration exhibits similar wear morphology, and, hence, has been omitted for brevity reasons. It can be clearly seen in Fig. 15(a) that wear occurs by mild abrasion. The load is carried by some of the nodules, and minor scratch marks are visible in Fig. 15(a), and more evidently in the magnified view in Fig. 15(b).
SEM micrographs of wear tracks at 30 N load and a sliding duration of 15 min are shown in Fig. 16 for Ni-P-W coating under lubricated sliding condition.
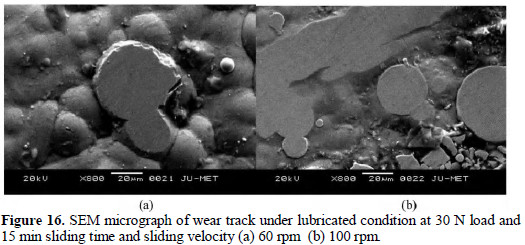
At 60 rpm sliding speed, mild abrasion and surface smoothening effect can be observed (Fig. 16(a)). The smoothening effect is more prominent at 100 rpm (Fig. 16(b)). Such effects tend to reduce the wear rate of the coatings. In Fig. 13(a) and Fig. 13(c), it can be observed that the wear depth becomes stable at higher values of sliding speed. This can be attributed to the smoothening effect observed in Fig. 16). Therefore, although the breakage of lubricant film might take place at higher applied normal loads, a decrease in wear depth is observed. In fact, the flowing lubricant carries away the wear debris, thereby preventing the ploughing effect caused by the particles. Hence, enhanced tribological behavior of electroless Ni-P-W coating is observed under lubrication compared to dry sliding condition. It also seems that boundary lubrication regime exists within the experimental domain considered. Thus, from the wear behavior and wear mechanism exhibited by the coatings, superior tribological behavior is established under boundary lubrication sliding condition.
At this point, it is interesting to note that the wear depth of the coatings obtained for both dry and lubricated sliding conditions is lower than the coating thickness. Moreover, the hardness of the coatings is lower than that of the hardened counterface disc. This indicates that only the wear of the coatings has taken place, and the measurement of wear in terms of displacement adequately represents the wear of the deposits.
Conclusions
In the present work, Ni-P-W coating is deposited by the electroless method onto AISI 1040 steel substrates. Tribological tests under dry and lubricated condition are carried out on a pin-on-disc test setup following Taguchi's L27 orthogonal array. Prediction models are developed using the experimental data through conventional regression method and fuzzy logic, which is an artificial intelligence technique capable of handling uncertain and vague data. The coefficient of determination of fuzzy rule based model is seen to be 97.7% and 98.7% under dry and lubricated conditions, respectively, indicating the high efficiency and prediction capabilities of the method. A lower coefficient of determination compared to the fuzzy logic method is observed for the response surface method. ANOVA results reveal that the highest contribution to wear under both the conditions is sliding speed followed by applied normal load and sliding duration. Three dimensional surface plots indicate an increase in wear with the interaction effect of the test parameters. But the coatings exhibit excellent wear resistance under lubricated condition, due to a surface smoothening effect at higher parametric combinations. Abrasive wear is the predominant mechanism under dry and lubricated condition. It is also seen that wear behavior of the coatings is mainly governed by the synergistic effects of the tribo-testing parameters, coating microstructure, surface morphology, strengthening of the deposits on heat treatment and surface smoothening effects. Finally, validation runs conducted at arbitrary parametric combination consolidated the outstanding prediction capabilities of the developed models. The present study thus widens the scope of Ni-P-W coatings for tribological contact applications working under lubricated condition. Moreover, the prediction models can reduce computational and experimental costs, as well as save time, leading to quicker decision making, and improve tribological behavior of electroless Ni-P-W coatings.
References
1. Sudagar J, Lian J, Sha W. J Alloys Comp. 2013;571:183. [ Links ]
2. Sahoo P, Das SK. Mater Design. 2011;32:1760. [ Links ]
3. Agarwala R C, Agarwala V. Sadhana. 2003;28:475. [ Links ]
4. Loto C A. Silicon. 2016;8:177. [ Links ]
5. Reddy V V N, Ramamoorthy B, Nair P K. Wear. 2000;239:111. [ Links ]
6. Gadhari P, Sahoo P. Procedia Mater Sci. 2014;6:623. [ Links ]
7. Gadhari P, Sahoo P. Surf Rev Lett. 2015;22:1550082. [ Links ]
8. Wu Y, Liu H, Shen B, et al. Tribol Int. 2006;39:553. [ Links ]
9. Wu Y, Shen B, Liu L, et al. Wear, 2006;261:2017. [ Links ]
10. Yoon JW, Park JH, Shur CC, et al. Microelectronic Eng. 2007;84:2552. [ Links ]
11. Guo Z, Keong KG, Sha W. J Alloys Comp. 2003;358:112. [ Links ]
12. Keong K G, Sha W, Malinov S. Surf Coat Tech. 2003;168:263. [ Links ]
13. Mukhopadhyay A, Duari S, Barman TK, et al. Port Electroch Acta. 2016;34:63. [ Links ]
14. Duari S, Barman TK, Sahoo P. Proc Mat Sci. 2014;5:978. [ Links ]
15. Ramalho A, Miranda JC. Wear. 2007;263:592. [ Links ]
16. Xu Y, Zheng X, Hu X, et al. Surf Coat Technol. 2014;258:790. [ Links ]
17. Chen WX, Tu JP, Gan HY, et al. Surf Coat Technol. 2002;160:68. [ Links ]
18. Der Ger M, Hou KH, Wang LM, et al. Mater Chem Phys. 2003;77:755. [ Links ]
19. Mukhopadhyay A, Duari S, Barman TK, et al. J Instit Eng (India): Series D. [ Links ]
20. Duari S, Mukhopadhyay A, Barman TK, et al. Int J Appl Eng Res. 2015;10:10090. [ Links ]
21. Duari S, Mukhopadhyay A, Barman TK, et al. Int J Eng Technol. 2016;7:94. [ Links ]
22. Oraon B, Majumdar G, Ghosh B. Mater Design. 2008;29:1412. [ Links ]
23. Oraon B, Majumdar G, Ghosh B. Mater Design. 2007;28:2138. [ Links ]
24. Choudhury BS, Sen RS, Oraon B, et al. Surf Eng. 2009;25:410. [ Links ]
25. Keong KG, Sha W, Malinov S. Mater Sci Eng: A. 2004;365:212. [ Links ]
26. Yating W, Bin S, Lei L, et al. J Mater Proc Technol. 2008;205:207. [ Links ]
27. Beygi H, Vafaeenezhad H, Sajjadi SA. Appl Surf Sci. 2012;258:7744. [ Links ]
28. Vaghefi SYM, Vaghefi SMM. Neural Comp Appl. 2011;20:1055. [ Links ]
29. Xu Y, Luan T, Zou Y. Artificial neural networks implementation in Ni-Cu- P ternary coating: Investigation of the effects of bath stabilizers. In: 8th Int Conf on Natural Computation (ICNC 2012). 2012;422. [ Links ]
30. Vaghefi SYM, Vaghefi SMM. A novel multilayer neural network model for heat treatment of electroless Ni-P coatings. Proceedings of International Joint Conference on Neural Networks. 2011; San Jose, California, USA. pp 3036. [ Links ]
31. Palaniappa M, Seshadri SK. Mater Sci Eng: A. 2007;460:638. [ Links ]
32. Balaraju, JN, Rajam KS. J Alloys Comp. 2009;486:468. [ Links ]
33. Wu FB, Tien SK, Chen WY, et al. Surf Coat Technol. 2004;177:312. [ Links ]
34. Tien SK, Duh JG, Chen YI. Thin Solid Films. 2004;469:333. [ Links ]
35. Tien SK, Duh JG. Thin Solid Films. 2004;469:268. [ Links ]
36. Balaraju JN, Manikandanath NT, Grips VW. Surf Coat Technol. 2012;206:2682. [ Links ]
37. Liu H, Yao HL, Thompson GE, et al. Surf Eng. 2015;31:412. [ Links ]
38. Liu H, Guo RX, Liu Z. Surf Coat Technol. 2013;219:31. [ Links ]
39. Liu H, Yao HL, Viejo F, et al. Transactions of the IMF. 2014;92:212. [ Links ]
40. Liu H, Guo RX, Zong Y, et al. Trans Nonferrous Metals Soc China. 2010;20:1024. [ Links ]
41. Roy S, Sahoo P. J Coat. 2013;Article ID 608140. [ Links ]
42. Roy S, Sahoo P. Port Electrochim Acta. 2012;30:203. [ Links ]
43. Shu X, Wang Y, Lu X, et al. Surf Coat Technol. 2015;276:195. [ Links ]
44. Latha B, Senthilkumar VS. Mater Manufacturing Proc. 2010;25:817. [ Links ]
45. Ramesh S, Karunamoorthy L, Palanikumar K. Mater Manufacturing Proc. 2008;23:439. [ Links ]
46. Kuram E, Ozcelik B. Indian J Eng Mater Sci. 2013;20:51. [ Links ]
47. Chatterjee D, Sutradhar G, Oraon B. J Mater Proc Technol. 2008;200:212. [ Links ]
48. Das MK, Kumar K, Barman TK, et al. Proc Eng. 2014;97:1587. [ Links ]
49. Das MK, Kumar K, Barman TK, et al. Proc Mater Sci. 2014;6:741. [ Links ]
50. Palanikumar K. Mater Manufacturing Processes. 2010;25:1059. [ Links ]
51. Asilturk I, Neseli S, Ince MA. Measurement. 2016;78:120. [ Links ]
52. Montgomery DC. Design and analysis of experiments. John Wiley & Sons; 2008. [ Links ]
53. Zadeh LA. Information Control. 1965;8:338. [ Links ]
54. Zak SH. Systems and control. New York: Oxford University Press; 2003. [ Links ]
55. Ajibola OO, Oloruntoba DT, Adewuyi BO. J Coatings. 2014;Article ID 841619. [ Links ]
56. Panja B, Das SK, Sahoo P. Surf Rev Lett. 2016;23:1650040. [ Links ]
57. Selvi VE, Chatterji P, Subramanian S, et al. Surf Coat Technol. 2014;240:103. [ Links ]
58. Minitab User Manual (Release 13.2). Making data analysis easier, MINITAB Inc. State College, PA, USA; 2001. [ Links ]
Acknowledgements
The authors gratefully acknowledge the financial support of University Grants Commission (UGC), Govt. of India, through Major Research Project vide Ref. No. F No. 41-984/2012 (SR) dated 25.07.2012.
*Corresponding author. E-mail address: psjume@gmail.com
Received July 14, 2016; accepted July 29, 2016X