Introduction
The use and importance of mobile devices, especially smartphones, have increased in consumer life. Recent statistics show that the number of global smartphone users was approximately 6.7 billion in 2023 and is predicted to reach 7.7 billion by 2028 (Statista, 2023a). Smartphones are multipurpose devices that perform many functions (Kim, Wang, & Roh, 2021). Smartphones and mobile applications (apps) running on smart devices have become indispensable components of daily life (Moon et al., 2022). Accordingly, there is a remarkable increase in the number and variety of mobile apps. As of 2022, there are approximately 4.8 million apps on the Apple App Store (Statista, 2023b) and 2.6 million apps on Google Play Store (Statista, 2023c). The development of mobile services continues in every aspect of daily life, with an approach of anytime, anywhere, for everyone and everything (Huang, Gartner, Krisp, Raubal, & Van de Weghe, 2018). Many users can use various mobile services (e.g., communication, travel, business, information gathering, entertainment, gaming, shopping, banking, payment, social networking, and utilities) offered by mobile service providers via mobile apps on their smartphones (Moon et al., 2022; Zhou, 2017).
With the development of mobile communication technologies, GPS and other network-based location services have become natural features of smartphones. These features have enabled individual users to easily use location systems and opportunities via smart devices (Tan, Tao, Si, & Zhang, 2020). Currently, almost every smartphone has geolocation features (Sobolewski, 2021). These opportunities and developments have made it possible for companies to provide services through mobile apps to customize the information and services offered based on user location information (Zhou, 2013a). Such services are called location-based services (LBS). LBS companies obtain users' location information using GPS and other network-location services, customize their service offerings according to user location, and create value by providing more customized information and services (Ayuning Budi et al., 2021; Schwipper, Peche, & Schmitz, 2020; Yoon, Kim, & Connolly, 2018). The LBS provides its users with a wide range of information, such as user location, the location they want to reach, acquaintances and companies nearby, and weather forecasts at the exact location (Vaughan-Nichols, 2009).
LBS-based mobile apps (LBS apps) have become widespread in mobile smart devices, particularly with the increasing use of mobile technology (Heo & Kim, 2017). The potential for location information to enhance the benefits of services and user satisfaction has also increased the number of mobile LBS applications of various types (Yoon et al., 2018).
Mobile navigation apps are a type of LBS increasingly used by consumers daily. Advances in GPS-based mapping apps (e.g., Google Maps and Apple Maps) have increased everyone's wayfinding and navigation abilities, including people with disabilities (Joseph & Namboodiri, 2019). More than 55% of smartphone users use navigation apps (WeAreSocial, 2023). Google Maps alone has over one billion active users (Sobolewski, 2021). The increase in the use of mobile navigation apps has intensified competition among service companies.
Developments in and opportunities for mobile navigation technologies have also led to diversified service offerings. However, in information systems and marketing, the long-term success of services depends on the continued use of the services (and/or system) and low customer retention costs (Bhattacherjee, 2001). The reuse of services is crucial for companies to gain a competitive advantage based on user loyalty. Therefore, it is necessary to understand why consumers continue to use specific apps among a wide variety of apps and how the reuse intention of the apps is formed (Yang & Lin, 2017). From this perspective, this study evaluates the importance of determining the factors that affect consumers' continuance intention to use mobile navigation apps.
Various studies in the literature explaining user behavior toward LBS apps have focused mainly on users' intentions to adopt and use such services and apps (e.g., Ayuning Budi et al., 2021; Gupta & Dogra, 2017; Zhou, 2012). A few studies also show users' intentions to reuse LBS apps. In these studies, it has been found that variables, such as benefit expectation, commitment, compatibility, effort expectancy, enjoyment, information quality, habit, justice, perceived usefulness, privacy concern, performance expectancy, privacy risk, satisfaction, sense of belonging, social interaction, service quality, system quality, trust, value (Akel & Armağan, 2021; Chen, Hsiao, & Li, 2020; Koohikamali, Mousavizadeh, & Peak, 2019; Wang, Teo, & Liu, 2020; Wang & Lin, 2017; Yang & Lin, 2017; Yu, Zo, Choi, & Ciganek, 2013; Yun, Han, & Lee, 2013; Zhou, 2013b) have effects on intention to reuse LBS. While some of these studies focus on LBS in general, others are specific to different types of LBS apps. However, to our knowledge, no study has examined the adoption of and intention to reuse mobile navigation apps as a type of LBS. Despite LBS's growing popularity among scholars and professionals, research on mobile navigation apps is also scarce, and existing studies mainly focus on user evaluations and perceptions (e.g., Girginkaya Akdağ & Ergen, 2020; Park & Ohm, 2014; Sobolewski, 2021). In addition, mobile navigation apps and services continue to evolve rapidly, and there is a need to develop more insights and research to understand user behavior in these apps (Huang et al., 2018). Moreover, as emphasized above, understanding why users continue to use such apps is essential for companies that develop these apps and provide services to increase consumer satisfaction and loyalty and gain a competitive advantage (Wirtz & Lovelock, 2018). This study addresses this research gap.
Some marketing and information technologies literature have revealed that service quality (e.g., Hung, Cheng, & Chiu, 2019; Wang, Ou, & Chen, 2019; Wangpipatwong, Chutimaskul, & Papasratorn, 2009) and flow experience (e.g., Cheng, 2014; Lee, 2010; Park, 2020) affect service reuse. On the one hand, service quality is an indicator of how well a service consistently meets consumer expectations (Parasuraman, Zeithaml, & Berry, 1988). Flow experience, on the other hand, refers to an experiential state in which consumers act with deep concentration, enjoyment, and involvement (Csikszentmihalyi, 1975; Kim, 2021).
Mobile navigation apps must provide services in accordance with consumer performance expectations and meet their needs in terms of content, information, and interfaces. In this respect, the quality of app service offerings is expected to affect consumer evaluation (Wang et al., 2019). Furthermore, mobile navigation app users are expected to interact continuously with the app, particularly when obtaining directions and wayfinding. This process involves goal setting, sense of control, focus, and involvement in an activity that requires full attention. Based on this, it can be argued that the flow experience plays a role in using mobile navigation apps (Zhou, 2013a). However, in terms of mobile LBS-more specifically, mobile navigation apps-no study in the literature explains users' continuance intention to use apps and services within the framework of service quality and flow experience. The present study aims to fill this gap in the literature.
In this study, we evaluate the service quality perceptions of consumers who use mobile navigation services, and find that their flow experiences while using the app and service are significant factors in reusing the app. Accordingly, this study attempts to answer the following questions: (1) How is the interaction between consumers' flow experience in mobile navigation apps and their intention to reuse this service? (2) Does location-based service quality play a role in this interaction? If yes, how?
With these research questions in mind, this study aims to determine the effect of consumers' flow experience on the reuse intention of mobile navigation apps and reveal the mediating role of location-based mobile service quality in this effect. This study focuses on reusing mobile navigation apps, which are among the most important location-based services. This study contributes to the emerging literature on LBS by explaining consumer reuse intention of mobile navigation apps in terms of experience and service quality. In addition, this study provides valuable insights for location-based service providers regarding the design of service processes.
2. Literature review
The following section discusses LBS and mobile navigation apps, flow experience, and location-based mobile service quality concepts in light of the current literature.
2.1 Location-based services and mobile navigation apps
LBS is defined as services that provide customized and enriched benefits based on the user's or object's physical (Dhar & Varshney, 2011). The first LBS emerged in the early 1990s (Huang et al., 2018). These services have become widespread, particularly with the development of mobile technology and positioning services (Yoon et al., 2018). LBS continue to evolve by focusing on providing useful information and services to consumers through mobile devices (especially smartphones) (Heo & Kim, 2017). LBS is provided via mobile apps on smartphones (Moon et al., 2022). With the help of satellite-based GPS and other mobile network services, the current location of smartphones can be determined, and LBS use this location to offer information and functions/services to consumers in a specific, personalized, and dynamic manner (Dhar & Varshney, 2011; Schwipper et al., 2020; Zhou, 2017).
Typical LBS includes services such as navigation, mapping, transportation, smart tourism, trip planning, location-based social networking and entertainment, location-based advertisements, local information searches, emergencies, family and contact discovery, and mobile check-in (Ayuning Budi et al., 2021; Gupta & Dogra, 2017; Zhou, 2013a). These services make life easier for consumers and provide various benefits (Schwipper et al., 2020). LBS can also be associated with consumers' lifestyle habits, behaviors, and personal characteristics (Wang & Lin, 2017). The location-based advantages provided by LBS apps can also enhance consumer experience (Zhou, 2013b).
Mobile navigation apps are among the most frequently used LBS apps. These apps mainly provide services to users for wayfinding and navigation through digital maps (Joseph & Namboodiri, 2019). These apps comprise three complementary elements (Sobolewski, 2021): (1) a signal from a satellite navigation system (global positioning system (GPS) technologies); (2) a receiver of this signal inside the user's device; and (3) a digital map of the terrain using signal processing software. Mobile navigation apps allow users to find any geographic point and plan travel, journey, or route based on these features provided (Gupta & Dogra, 2017). Modern navigation apps continue to improve with additional layers of dynamic information, such as real-time traffic and transportation information (Nagaraj & Stern, 2020).
In general, LBS apps, particularly mobile navigation apps, have been widely adopted by users (Zhou, 2017). Various academic studies have been conducted on the reasons for adopting LBS apps by employing TAM (Davis, 1989), UTAUT (Venkatesh, Morris, Davis, & Davis, 2003), and UTAUT2 (Venkatesh, Thong, & Xu, 2012) theories. These studies have revealed that ease of use, effort expectancy, familiarity, facilitating conditions, fear of crime, flow, habit, hedonic motivation, innovativeness, perceived risk, perceived severity, performance expectancy, privacy concern, self-efficiency, social influence, social motivation, subjective norms, trust, usefulness, and utilitarian motivation variables have direct or indirect effects on users' intentions to adopt LBS apps (Ayuning Budi et al., 2021; Gupta & Dogra, 2017; Kim, Yoon, & Zemke, 2017; Park & Ohm, 2014; Xu & Gupta, 2009; Zhou, 2012, 2013a).
In addition to studies investigating the adoption intention of LBS apps, other studies have focused on user perceptions and evaluations of the apps. For example, Constantiou, Lehrer and Hess (2014) found that the high value offered by an LBS app compared to its alternatives and the user experience affect the reuse of the app. Choi (2018) revealed that location-based service offerings increase the usefulness of mobile shopping apps and indirectly affect their adoption. Schwipper et al. (2020) revealed that monetary support, convenience, fun, social, and epistemic benefits and irritation affect the perceived value of mobile LBS. Kim (2021) determined that the perceived value of location-based retail apps affects the intention to use and purchase them.
Another significant issue is why users continue to use LBS even after adopting this technology. This issue, which is the main focus of the present study, is addressed in the literature as continuance usage intention. Continuance usage intention (or reuse intention as discussed in this study) refers to users' post-adoption behavior. This represents users' willingness and motivation to continue using a service in the future, especially in terms of products involving technology and information systems (Bhattacherjee, 2001).
Previous studies have revealed the effects of various variables on consumers' intentions to continue using LBS apps. The details of these studies are summarized in Table 1.
In the literature, no study has directly focused on the reuse intention of mobile navigation apps. Various findings include consumer evaluations and perceptions in the context of mobile navigation apps. For example, Park and Ohm (2014) revealed that location accuracy, service and display quality, and mobility are the main psychological factors affecting users' perceptions of and use of mobile map services. Dalton and Thatcher (2019) found that if mobile navigation users have brand knowledge of a company pinned on a map or if there is a social context, users remember or learn the company's location on the map. Girginkaya Akdağ and Ergen (2020) concluded that connection, content, interaction, and contextual qualities are significant factors in location-based apps' usage behavior, and more specifically, location accuracy and ease of use are also important factors in the usage of mobile navigation apps. On the other hand, Sobolewski (2021) revealed that mobile navigation users perceive these apps as more valuable when they obtain location-based benefits and control over their personal information.
2.2 Flow experience
Flow experience, a psychological concept, is defined as the holistic feeling people experience when they act with full attention (Csikszentmihalyi, 1975). Flow is also summarized as the immersed feeling that a person experiences when interacting with/using a particular service or technology (Park, 2020). In a flow state, people are involved in an activity and enjoy the experience of the process with such full concentration that nothing else seems to matter and is not interrupted by external factors (Csikszentmihalyi, 2013; Wu, Chiu, & Chen, 2020).
Flow is a complex concept, and the related literature emphasizes that it is often treated using a multidimensional approach (Hoffman & Novak, 2009; Lee, 2010; Ozkara, Ozmen, & Kim, 2017). Previous studies have stated that the flow experience consists of many components, including the enjoyment of performing the activity, perceived control, union of action and awareness, immersion, attention focus or concentration, forgetting of time, temporal dissociation, curiosity, and intrinsic interest (Cheng, 2014; Koufaris, 2002; Ozkara et al., 2017). Among these, perceived enjoyment, perceived control, and attention focus are the most frequently used dimensions (Koufaris, 2002; Zhou, 2013a).
Perceived enjoyment represents the perception of pleasure and fun resulting from the use of IT (Zhou, 2013a). Perceived control is defined as the emotion that arises when a user perceives that he/she is in control of his/her activity and interactions with the surrounding environment while using technology (Ozkara et al., 2017). Attention focus refers to the user's concentration, immersion, and involvement in using technology (Koufaris, 2002).
Flow has been studied in various contexts based on human-information system interactions, such as online shopping, e-learning, computer games, online games, social networking sites, e-mail, mobile TV, mobile gaming, and mobile messaging (Chang, Liu, & Chen, 2014; Lee, 2010; Zhou, 2013a). Various studies have theorized that a positive flow experience can attract consumers and positively affect their subsequent attitudes and behaviors (Kim, 2021).
2.3 Service quality and location-based mobile service quality
Service quality represents consumer perceptions and evaluations based on a comparison of their expectations of the service and the performance they perceive from their service experience (Parasuraman, Zeithaml, & Berry, 1985; Parasuraman et al., 1988).
Service quality significantly affects consumer evaluations, attitudes, and behaviors in the post-encounter stage of service consumption, such as satisfaction, loyalty, and word-of-mouth (Wirtz & Lovelock, 2018).
Various researchers have investigated service quality dimensions from different theoretical perspectives and developed models and scales (Shao, Li, Guo, & Zhang, 2020). Service quality was conceptually first discussed by Grönroos (1984). Grönroos (1984) emphasized that service quality has two dimensions: technical and functional. One of the most popular approaches developed for conceptualizing and measuring service quality is SERVQUAL, which has five basic dimensions: reliability, assurance, tangibles, empathy, and responsiveness (Parasuraman et al., 1985, 1988). The SERVQUAL scale has continued to be widely used in different contexts (including e-services) due to its relative stability and robustness in measuring service quality (Hung et al., 2019; Shao et al., 2020).
With technological developments and opportunities provided by the Internet, service delivery channels have transitioned from offline to online. The widespread use of online services (e-services), primarily e-commerce, and their acceptance by consumers has led to the development of an e-service quality approach (Huang, Lin, & Fan, 2015). E-services differ from services in traditional environments. The virtual nature of e-services, the absence or limitation of face-to-face interactions between staff and customers, the prominence of human-computer interaction, and the technical framework differentiate e-services from traditional services (Wang et al., 2019). This difference makes it necessary to differentiate between e-SQ processes (e-SQ). Consequently, various e-SQ scales, such as eTailQ (Wolfinbarger & Gilly, 2003), E-S-QUAL (Parasuraman, Zeithaml & Malhotra, 2005), have emerged to measure different e-service contexts. Blut, Chowdhry, Mittal and Brock (2015) conducted a meta-analytic review of e-SQ and evaluated the e-SQ scales frequently used in the literature. They revealed that the e-SQ has four primary dimensions and later confirmed these findings in Blut's (2016) study. Accordingly, these dimensions are (1) website design, (2) fulfillment, (3) customer service, and (4) security and privacy.
Mobile technology developments have diversified mobile services and increased their use, triggering an approach to measure mobile service quality (Huang et al., 2015). However, mobile environments and services have different characteristics, which may affect service quality evaluations. For example, mobile services have unique features such as mobility, being anywhere, location- centeredness, information processing anytime and anywhere, identifiability, and personalization (Lu, Zhang, & Wang, 2009; Özer, Argan, & Argan, 2013; Wang & Li, 2012). Thus, it is necessary to consider mobile service quality (m-SQ) separately from e-SQ. There is no generally accepted structure in the literature for m-SQ measurements. Various studies have investigated m-SQ as a multidimensional concept adapted from e-SQ (e.g., Trabelsi-Zoghlami, Berraies, & Ben Yahia, 2020). Researchers have mostly adapted existing e-SQ scales by adapting them to measure m-SQ (Özer et al., 2013). In addition, although limited, various scales have been developed specifically for measuring m-SQ in the literature (e.g., Huang et al., 2015; Lu et al., 2009).
Current studies on m-SQ focus on various types of mobile services rather than mobile LBS. Although current m-SQ measurement and evaluation approaches explain some characteristics of mobile LBS quality, other characteristics of mobile LBS (especially locationability and related customization aspects) have not been clearly explained (Heo & Kim, 2017). Based on this argument, Heo and Kim (2017) developed a multidimensional scale to measure the quality of mobile LBS. The mobile LBS quality scale dimensions include information, localization, function, personalization, design, reliability, connection, interaction, and security (Heo & Kim, 2017).
2.4 Hypothesis Development
The following sections explain the findings of previous studies as the basis for the hypothesis formulation in the present study.
Flow and Reuse intention
Consumers must interact with the app when using mobile LBS and mobile navigation apps. During this process, the consumers are in a state of flow. Positive or negative experiences during the flow state will likely affect the intention to reuse an app. Various studies have revealed the effects of the flow experience on the reuse intentions of mobile services and apps. For example, Zhou (2013b) emphasized the importance of experience in LBS and demonstrated that flow experience affects usage intention. Other studies conducted by the same author on mobile Internet and mobile games demonstrated that flow affects reuse intention (Zhou, 2011, 2013c, 2013d). Park and Ohm (2014) revealed that the flow state while using mobile map services positively affects the intention to use these services. Gao and Bai (2014) found that the flow experience in mobile apps that provide social networking services positively affects the intention to continue using the apps. Zhou (2016) determined that flow strongly affects the intention to reuse LBS, including mobile navigation apps. Rauschnabel, Rossmann and Tom Dieck (2017) also found that flow significantly affects the reuse intention of mobile augmented reality game apps and the intention to purchase in-apps. Jamshidi, Keshavarz, Kazemi and Mohammadian (2018) revealed that the flow experience positively affects the intention to continue using apps that provide mobile banking services. Sarkar and Khare (2019) showed that the flow experience positively affects the intention to continue using mobile shopping apps. Park (2020) demonstrated that the flow state of users interacting with smart wearable devices positively affects their intention to continue using the device.
Based on the results of previous studies, the following hypotheses were developed:
H1: Experiencing flow state when using the mobile navigation app (flow experience) affects the reuse intention of the app.
Location-based mobile service quality and Reuse intention
Consumers of LBS and mobile navigation apps evaluate the extent to which performance expectations from the app are met. These evaluations shape their subsequent attitudes and behaviors (Wirtz & Lovelock, 2018). Therefore, it can be argued that consumers' service quality evaluations toward mobile apps affect their intention to reuse them. Various studies in the literature support this situation and establish the relationship between service quality and reuse intention. Santos (2003) stated that e-service quality is critical to consumers' purchase and reuse intentions. Li, Duan, Fu and Alford (2012) reported that service quality affects reuse intention in the context of e-learning. Consistent with these findings, Malhotra and Kubowicz Malhotra (2013) found that mobile service quality affects the intention to purchase and continue using mobile service providers. Akter, Ray and D'Ambra (2013) revealed that service quality affects the intention to reuse mobile apps that provide health services. Noh and Lee (2016) found that the perceived service quality of mobile banking apps positively affects consumers' reuse intentions. Almaiah and Al Mulhem (2019) revealed that the perceived service quality of mobile learning apps affects consumers' reuse intentions. Khalil and Suharjito (2019) found that service quality affects the intention to reuse mobile apps that provide ridesharing services. Moreover, Liébana- Cabanillas, Molinillo and Ruiz-Montañez (2019) investigated the use of NFC mobile payment systems for public transportation services and determined that service quality affects the reuse intention of the app. Chiu, Ho, Yu, Liu, and Mo (2021) also showed that consumers' perceived service quality from mobile retail service apps supported with augmented reality affects the reuse intention of the app.
Based on the findings of relevant literature, this study hypothesized that:
H2: Perceived service quality of the mobile navigation app (location-based mobile service quality) affects the reuse intention of the app.
Flow and Location-based mobile service quality
Consumers experience flow while using LBS and mobile navigation apps, which shapes their service evaluations. Considering that service quality is a critical consumer evaluation in the post-encounter stage of service consumption (Wirtz & Lovelock, 2018), it can be claimed that flow experience affects service quality perceptions. Various studies have shown that the flow experience affects service quality in contexts other than mobile services. For example, Shamdasani, Mukherjee and Malhotra (2008) investigated consumer evaluations in the context of self-service Internet technologies and revealed that enjoyment and control, which are dimensions of flow, are among the factors that affect service quality. Nambisan and Watt (2011) examined user experience in an online product community offered by companies as a product-related service. They also found that user experience affects service quality, especially hedonic and pragmatic experiences. Obadă (2014) determined that flow affects the service quality of branded websites. In another study, Rareş (2014) demonstrated a conceptual model explaining e-service quality and showed that flow significantly affects service quality. Wu, Gursoy, and Zhang (2021) separately evaluated the effects of self-service technology and human contact-based experiences on user behavior in restaurants. They found that the flow experience, especially technology- based self-service, affects service quality.
Based on the findings, this study hypothesized that:
H3: Flow experience affects location-based mobile service quality.
Considering the related literature explained above in detail, it has been hypothesized that location-based service quality plays a mediating role in the relationship between flow experience and reuse intention.
H4: Location-based mobile service quality mediates the relationship between flow experience and reuse intention.
Figure 1 illustrates the research model constructed based on the hypotheses.
3.Methods
This study employed a quantitative research design to test its research model and hypotheses (Malhotra, 2020). As shown in Figure 1, the flow experience is the independent variable, location-based mobile service quality is the mediator variable, and reuse intention is the dependent variable.
Existing well-established 5-point Likert-type scales, ranging from strongly disagree (1) to strongly agree (5), were adapted to measure the variables in this study. Flow experience was measured using ten items adapted from Koufaris (2002) and Zhou, Li and Liu (2010). Location-based mobile service quality was measured using a scale developed by Heo and Kim (2017). Reuse intention was measured using a scale originally developed by Venkatesh et al. (2003) and adapted from Schaupp (2010). All scales were adapted to the context of mobile navigation applications and translated into Turkish. Expert opinions regarding language and context were consulted during the adaptation process of the scales. A pre-test was also conducted.
The study population consisted of smartphone owners who actively used a navigation app in general and mobile navigation app to help reach an unknown address in the last two weeks. Continuous assistance from the mobile navigation app while reaching an unknown address was determined to be the essential criterion for respondents to be included in the sample. These types of direction and wayfinding are the conditions under which a user's interaction with the mobile navigation app is greater. It is believed that under these conditions, the flow state can be better measured, and its effects can be better evaluated. As stated in the literature, the flow experience requires an uninterrupted process and concentration.
There were no limitations regarding the brand of mobile navigation app used by the participants. This was sufficient for the participants to use any brand. During the data collection process, the participants were asked about the brand name of the mobile app they had used in their most recent experience. They were asked to answer other scales based on their experiences with the app. To help users specify the brand name of the app they use, the three most popular apps (Apple Maps, Google Maps, and Yandex Maps) in terms of download numbers during the research period were included as options under the relevant questions. In addition, the "other" option has been added so that other brand users could specify their app's brand name.
Data were collected via an online survey, and respondents were selected using the convenience sampling method (Malhotra, 2020). The survey link was shared on various relevant community pages on social networking sites (e.g., Facebook, LinkedIn) and various online forums (e.g., technology-focused) during the data collection process. In addition, to reach a broad audience, various users with several followers on social media shared the survey link. Consequently, 513 respondents answered the survey completely and adequately.
The data were analyzed using covariance-based structural equation modeling (CB-SEM) using AMOS (Hair, Black, Babin, & Anderson, 2019). A two-step approach was used to test the hypothesized effects (Anderson & Gerbing, 1988). In the first step, the measurement model was tested using confirmatory factor analysis (CFA) to assess the discriminant and convergent validity. Subsequently, a structural model was developed based on the measurement model and tested using path analysis (PA). In order to test the mediating role of location-based mobile service quality, Baron and Kenny's (1986) procedure was followed.
4. Results
Table 2 shows the respondents' sociodemographic characteristics.
Since the data were collected from the same participant group during the same period, there may be a common method bias (CMB) (Podsakoff, MacKenzie & Podsakoff, 2012). Three different statistical approaches were used to check for the presence of CMB. First, Harman's one-factor test was conducted (Podsakoff, MacKenzie, Lee, & Podsakoff, 2003). In this method, the items of all variables were evaluated in an unrotated one-factor solution with an eigenvalue greater than 1 in the EFA. The first factor accounted for 39.17% of the total variance. As this is lower than the critical limit of 50%, it indicates no CMB. Second, we used the CFA-based Harman test (Malhotra, Kim & Patil, 2006). In the CFA, the items of all variables were modeled as a single unmeasured latent variable, and model fit was evaluated. As a result of the analysis, the model did not fit the data (χ²(495) = 7691.893, p < .001; χ²/df = 15.539, CFI = 0.485; RMSEA = 0.169; SRMR = 0.120). This is evidence for the absence of CMB. Third, the correlation matrix between the variables was examined according to Pavlou, Liang and Xue (2007). The highest correlation in the correlation matrix (Table 5) was 0.70. Since this value is lower than the critical value of 0.90, it indicates no concern regarding CMB. As a result of these approaches, the hypothesis test results in the study were not biased because of CMB.
Before testing the hypotheses, exploratory factor analysis (EFA) was conducted for flow experience (FE), location-based mobile service quality (LB-MSQ), and reuse intention (RUI) variables to evaluate the construct validity of the scales and decide appropriate factor structures for the scales, since these scales were adapted. In the EFAs, principal component analysis with the varimax rotation method was applied to determine the factor structures. Items with factor loadings less than 0.60, items that loaded more than one factor, and items that deteriorated the original factor structure were removed, and the analysis was repeated until appropriate factor structures were constructed (Hair et al., 2019).
Table 3 presents the results for the final factor structures obtained based on the EFAs. As shown in Table 3, the FE scale has three dimensions: (1) perceived enjoyment (FE-PE), (2) attention focus (FE-AF), and (3) perceived control (FE-PC). The LB-MSQ has five dimensions: (1) information quality and reliability, (2) personalization, (3) design quality, (4) security and privacy, and (5) interaction quality. These names were assigned according to the interactions between the items and names used in the original scale. Additionally, the RUI scale was found to be unidimensional.
The reliability of the scales was evaluated using Cronbach's alpha coefficient (Malhotra, 2020). As seen in Table 3, the Cronbach's alpha coefficients were 0.75 for the FE scale, 0.94 for the LB-MSQ scale, and 0.83 for the RUI, respectively. As Cronbach's alpha coefficients above 0.70 are considered acceptable (Malhotra, 2020), all the scales were found to be reliable.
Table 3 Results of exploratory factor analysis
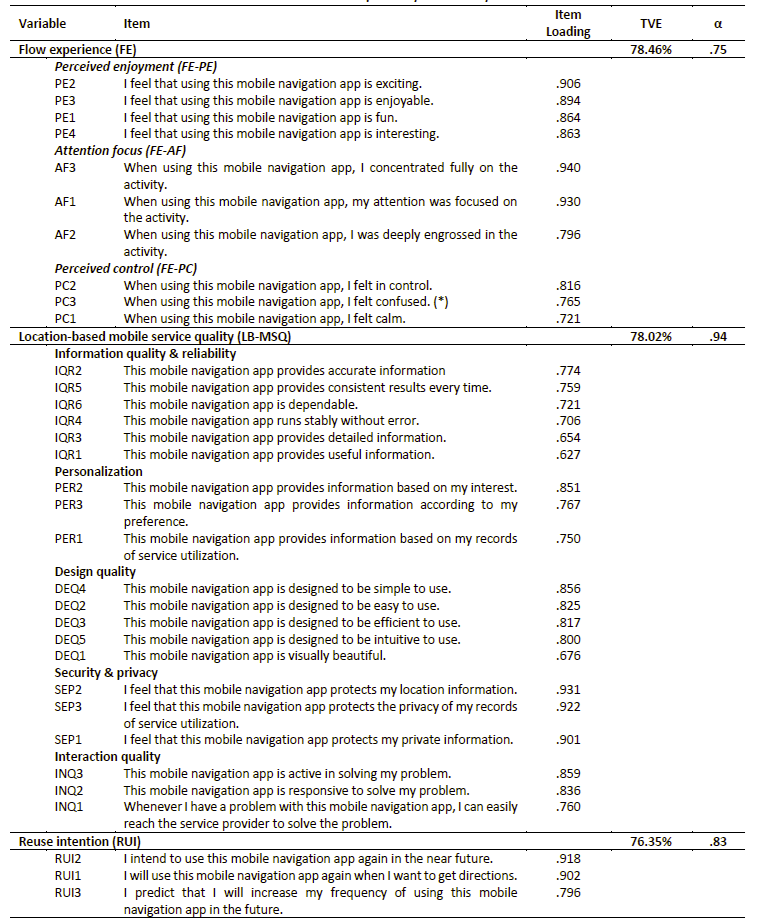
(*) marked expressions are reverse-coded. TVE = Total variance explained, α = Cronbach's alpha coefficient
4.1 Measurement model
The measurement model was constructed based on the factor structures obtained from the EFAs for the research variables. In this model, LB-MSQ was defined as a second-order structure, and FE was a first-order structure. In the model, the sub-factors of FE were defined as separate latent variables so that the effects of the sub-factors of FE on RUI were more specifically analyzed. CFA was used to assess the convergent and discriminant validity of the constructs in the measurement model. The criterion of Fornell and Larcker (1981) was used to assess the convergent validity of the model, and Average Variance Extracted (AVE) and Composite Reliability (CR) values were calculated (AVE≥.50; CR≥.70). To assess the discriminant validity of the model, the second criterion was that the correlation between the two latent variables should not be greater than the square root of the AVE values for each construct (Fornell & Larcker, 1981). Besides, it was evaluated whether the model fit values calculated for the model are within limits commonly accepted in the literature [χ2/df (Good Fit ≤ 3), RMSEA (Acceptable Fit ≤ .08), CFI (Acceptable Fit ≥ .90), SRMR (≤.08); (Dash & Paul, 2021; Hooper, Coughlan, & Mullen, 2008; Hu & Bentler, 1999)].
Table 4 shows the factor loadings, CR and AVE values, and model fit values calculated for the measurement model. As shown in Table 4, the measurement model meets the minimum criteria for convergent validity and fit.
Table 5 shows the mean and standard deviation values of the latent variables and correlation matrix representing the correlations among the latent variables in the measurement model. The diagonal of the matrix represents the square root of the AVE values.
As seen in Table 5, there is no multicollinearity problem (|r|<.90; Hair et al., 2019), and the correlation between each pair of variables is lower than the square root of the AVE values of these variables (shown as bold and italic at diagonal). Therefore, the measurement model meets the criteria for discriminant validity.
4.2 Structural model and mediation test
A structural model was constructed based on the final measurement model, and the relationships in the structural model were analyzed using path analysis. Additionally, the mediation effects in the model were analyzed following Baron and Kenny's (1986) 4- step approach. This approach was applied according to the testing procedures proposed by James, Mulaik, and Brett (2006) for SEM and the practical applications of Baldauf, Cravens, Diamantopoulos and Zeugner-Roth (2009), Grace and Weaven (2011), and Luo and Bhattacharya (2006). Accordingly, direct (Model 1) and mediation models (Model 2) were formed and analyzed. Model 1 was constructed by defining the direct effects of the FE subfactors (perceived enjoyment, perceived control, and attention focus) on the RUI (see Figure 2).
The effects arising from the LB-MSQ (second-order level) were defined in Model 2 (see Figure 3), in addition the casual relationships in Model 1.
Using path analysis, path coefficients (β), t, model fit, and R2 values were calculated for each model. In addition, the change statistics used to compare Models 1 and 2 were calculated. Besides, the chi-square difference test was applied to evaluate whether the change in χ2 values of both models is significant. Table 6 shows the results of the path analyses and chi-square difference test.
According to results obtained from the direct model (Model 1), perceived enjoyment (FE-PE) and perceived control (FE-PC) have positive effects (β1=.256; β2=.374; p<.05), whereas attention focus (FE-AF) has a negative effect (β3a=-.094; p<.05) on reuse intention (RUI). Thus, H1 is supported. Accordingly, in Model 1, the subfactors of flow experience explain 35.1% of the variance in reuse intention.
According to Baron and Kenny's (1986) procedure, four conditions must be met for a mediation effect to exist: (1) the independent variable should affect the mediator variable, (2) the mediator variable should affect the dependent variable, (3) the independent variable should affect the dependent variable, and (4) after the mediator variable is added to the model, the previously significant effect of the independent variable on the dependent variable should become statistically insignificant or decrease. In the fourth condition, insignificance of the effect is interpreted as the presence of full mediation, whereas a decrease in the effect is interpreted as the presence of partial mediation.
A mediation model (Model 2) was formed by adding the LB-MSQ to Model 1. In Model 2, FE-PE and FE-PC have positive effects on LB-MSQ (β4=.309; β5=.581; p<.05); however, FE-AF does not have a significant effect on LB-MSQ (p>.05). Accordingly, H2 is partially supported, and Baron and Kenny's (1986) first condition is met for FE-PE and FE-PC. In addition, LB-MSQ positively affects RUI (β7=.656; p<.05). Accordingly, H3 is supported, and Baron and Kenny's (1986) second condition is met. After that, in Model 1, FE- PE, FE-PC, and FE-AF as sub-factors of flow experience affect the RUI (β1a=.256, β2a=.374, β3a=-.094; p<.05). When the LB-MSQ is included in the model, the effects of FE-PE and FE-PC on the RUI also become insignificant (p>.05). Thus, the third and fourth conditions of Baron and Kenny (1986) are met. However, FE-AF has continued to affect the RUI negatively, and the effect size has also been increased (β3b=-.134). As a result, LB-MSQ has a full mediation effect on the relationships between FE-PE and RUI and between FE-PC and RUI. In contrast, there is no mediating effect on the relationship between FE-AF and RUI.
When the LB-MSQ is included in the model, the proportion of the variance in the RUI explained by other variables in Model 2 increased to 52.9%. As seen in the model fit values in Table 6, both models meet the minimum model fit requirements; however, Model 2 has better model fit values than Model 1. According to the chi-square difference test results, there is a statistically significant difference between the chi-square values of the models (χ2=1125.208; df=421; p=.000). The chi-square difference test shows that if the difference is statistically significant according to the χ2 and df values of the two models, the model with more parameters fits the data better (Werner & Schermelleh-Engel, 2010). In this study, since Model 2 has more parameters than Model 1, and the chi-square difference is significant, the mediation model is better than the direct model. According to these findings, H4 is partially supported for FE-PE and FE-PC.
5. Discussion
The findings demonstrated that service quality mediates the relationship between perceived enjoyment and perceived control dimensions of flow experience and reuse intention in the context of LBS and mobile navigation apps. Accordingly, a significant determinant of using mobile navigation apps repeatedly is the flow experience that users perceive when using them to obtain direction while driving. These results are consistent with those of previous studies on the relationship between flow experience and reuse intention (Gao & Bai, 2014; Jamshidi et al., 2018; Koufaris, 2002; Park, 2020; Park & Ohm, 2014; Rauschnabel et al., 2017; Sarkar & Khare, 2019; Zhou, 2013c, 2013a, 2016, 2011, 2013d). However, a significant portion of this effect was realized through the perceived service quality of mobile navigation apps. As a result, it has been concluded that perceived service quality increases as the perceived enjoyment and perceived control level of mobile navigation apps increase. Moreover, when perceived service quality increases, consumers' reuse intentions for mobile navigation apps also increase.
According to the study results, the mediating role of service quality increased the explained variance in reuse intention. Consequently, the higher the perceived service quality of mobile navigation apps, the more likely consumers will use them again.
Information quality, reliability, and design quality are the most significant factors affecting consumers' perceptions of mobile service quality. These are followed by interaction quality and personalization, whereas security and privacy are the least important factors. Accordingly, in addition to meeting consumer quality and reliable information needs, design quality is essential for forming service quality for mobile navigation apps. Furthermore, the ability of a mobile navigation app to provide personalization options and interaction opportunities with other users is a significant determinant of service quality.
The study results regarding the effect of perceived service quality on reuse intention are compatible with the findings of previous studies (Akter et al., 2013; Almaiah & Al Mulhem, 2019; Chiu et al., 2021; Khalil & Suharjito, 2019; Li et al., 2012; Liébana-Cabanillas et al., 2019; Malhotra & Kubowicz Malhotra, 2013; Noh & Lee, 2016). Furthermore, the study results regarding mobile navigation apps are considered important because the number of such studies is limited. Moreover, this study also supported the mediating role of service quality in the relationship between perceived enjoyment and perceived control dimensions of flow experience and reuse intention.
An exciting finding is that service quality does not mediate the relationship between the attention-focus dimension of the flow experience and reuse intention. However, the attention-focus dimension of the flow experience has a direct but negative effect on reuse intention. This finding is incompatible with those of Zhou (2011), Zhou (2013b), and Zhou (2013c), who reveal that attention focus positively affects mobile service quality. However, since the context of the present study is mobile navigation apps, this finding is not contradictory. Drivers should focus on secure driving and traffic rules rather than on other stimuli, including mobile navigation apps. In addition, Zhou's (2011, 2013c, 2013d) studies address mobile service quality for mobile websites and mobile game contexts; therefore, non-compatibility in the results arises from the attention-focus difference in mobile navigation apps.
5.1 Theoretical Implications
The findings of this study are important for the relevant literature. Notably, the role of mobile service quality in the relationship between the flow experience and consumers' future usage intentions was examined and defined for location and mobility-based apps. Additionally, this study measured mobile service quality using the location-based approach proposed by Heo and Kim (2017) and demonstrated that it is a valid and reliable scale for measuring service quality in the context of mobile navigation apps. As there is a limited number of empirical studies on location-based navigation services, this study is expected to significantly contribute to the services marketing literature.
5.2Managerial Implications
The study findings can benefit marketing practitioners by providing insights into designing service proposals and realization processes for mobile navigation apps. Additionally, the findings can help identify the critical elements of consumer-oriented app design, such as the importance of flow experience, service quality, and information reliability in LBS app design. App designers should focus on creating user-friendly apps with colors, fonts, and in-app designs suitable for target consumers' tastes and expectations. Mobile navigation service providers can also enhance users' flow experiences and encourage app usage by adding multimedia features such as augmented reality and gamification. Furthermore, mobile navigation apps should prioritize providing information and services that do not violate users' privacy concerns or negatively impact the app experience. The findings also contribute to the fields of leisure and tourism. Wayfinding and navigation are important for tourists, especially in unfamiliar environments (Chang, 2015). Therefore, understanding user behavior in mobile navigation apps will help design more tourist- oriented LBS apps.
6. Conclusions
This study investigated the effect of consumers' flow experience on the reuse intention of mobile navigation apps and the mediating role of location-based mobile service quality. The study concluded that users' experiences while using a mobile navigation app and their service quality perceptions are significant determinants of their intention to reuse the app.
More specifically, the flow experience while using the app is a significant determinant of users' reuse intentions for mobile navigation apps. However, only the attention-focus dimension of the flow experience directly and negatively affects the reuse intention of the app. The perceived enjoyment and control dimensions also indirectly and positively affect users' intention to reuse the app. This effect is mediated by the quality of location-based mobile services. In this case, the critical point is the perception of service quality. According to these results, attentional focus is an essential determinant of reuse intention. In other words, the more the user concentrates on the app and is immersed in the process, the lower the reuse intention, regardless of the quality assessment of the service obtained from the app.
However, users' quality perceptions of the services provided by the app are powerful determinants of their reuse intentions. When service quality perception increases, the reuse intention also increases. Information and design quality are the most significant factors affecting perceptions of service quality. Interaction quality, personalization, security, and privacy perceptions are other factors that affect user perceptions of service quality.
Limitations and Further Research
Although this study has some limitations, it is useful to approach the findings within this framework. The scope of this study is limited to mobile navigation apps running on smartphones and users receiving continuous/uninterrupted assistance from mobile navigation apps in the process of reaching an unknown address. Therefore, the research model should be tested using different samples and contexts. This study explains consumer reuse intentions for mobile navigation apps through location-based mobile service quality and flow experience variables. However, other variables may also affect consumers' intentions to reuse mobile navigation apps. Designing and analyzing different research models by employing other possible variables affecting reuse intention, such as customer satisfaction and perceived risk, is recommended for further studies to better understand this concept.