Introduction
With a growing workload for Reporting Radiologists, artificial intelligence (AI) may reduce the burden of repetitive tasks and standardize the reporting workflow. It may also increase diagnostic precision when interpreting medical images.
AI-Rad Companion Chest CT (AIRC-cCT), ) by Siemens Healthineers®, Germany, is an AI-based automated post-processing solution that detects, highlights and quantifies relevant anatomy and abnormalities. Results are displayed as tables and images and a traffic-light color scheme is used to highlight/classify measurements outside the defined thresholds. By integrating this solution into clinical workflows, both images and supporting information can be accessed automatically on any PACS for reporting. Siemens Healthineers obtained CE-Mark approval to market AIRC-cCT software in European markets; it has also been cleared by the FDA for clinical use.
Our Hospital’s Imaging Department conducts over 14.250 chest CTs annually.
Emphysema is defined as a permanent and abnormally dilated airspace distal to the bronchial terminal. On CT, it is defined by a density below -950 HU,1 which strongly correlates with microscopic and macroscopic emphysema.1
In clinical practice, radiologists commonly do a visual assessment of emphysema using the criteria of Fleishner’s Statement, dividing it into: centrilobular (CLE), panlobular and paraseptal(PSE).2
CLE can be further graded: trace (<0.5% of lung volume), mild (0.5-5%), moderate (>5%), confluent and advanced destructive (ADE-panlobular lucencies). Panlobular emphysema is associated with A1AT deficiency. PSE includes mild type (≤1cm) and substantial.2
Lung cancer is the most common cancer in men, the second most common cancer in women and is the leading cause of cancer death, with an estimated 1.8 million deaths in 2020.3
Recent clinical trials have demonstrated the efficacy of low-dose CT imaging as a lung screening tool,4,5,6,7with a reduction in mortality rates.8 The majority are detected in CT screening participants at stage I,9,8,10,11,12showing their role in primary prevention, with a potential increase in survival rates. NLST showed that, in high-risk former and current smokers, volume CT lung-cancer screening resulted in lower referral rates for further tests and inferior lung cancer mortality.9
Enlargement of the thoracic aorta is common, with an estimated incidence of 5-10/100,000 person/year.13 It’s mostly an asymptomatic incidental finding. The European Society of Cardiology guidelines clarify that, in healthy adults, aortic diameters should be <40mm and taper gradually downstream, with a diameter on the descending aorta <30mm.14,15,16 There is no consensus between leading societies regarding these measurements, neither related to definition nor to cut-off, surveillance or treatment.
Guidelines recommend measurement of diameters perpendicular to the direction of blood flow, which requires additional post-processing, adding time to the report. Predefined locations for measurements are (S1) sinuses of Valsalva, (S2) sino-tubular junction, (S3) mid ascending, (S4) proximal and (S5) mid arch, (S6) proximal and (S7) mid descending, (S8) diaphragm and (S9) abdominal.17,18
Bone density is defined as the quantity of mineral bone contained within total bone tissue and is usually quantified using dual-energy x-ray absorptiometry(DEXA).19 This health marker is often ignored in asymptomatic/low-risk patients, which in conjunction with the low availability of DEXA scanners significantly limits our ability to diagnose and follow-up vertebral collapse and associated co-morbidities. The latter can be highly impactful by reducing quality of life and increasing healthcare spending. Comparatively, CT scanners are ubiquitous and can be used as an opportunistic cheap and convenient screening tool.20
Materials and Methods
The main objective of this work was to compare the performance of AIRC-cCT(version VA22B, Siemens Healthineers®, Germany) by analyzing chest CT scans and comparing the results against those obtained from Radiologists. We aim to understand if this tool has a positive clinical impact in imaging departments.
We retrospectively and randomly selected a sample size of 350 chest CT scans, performed with or without intravenous iodine contrast agent, from the pool of exams performed in January of 2021 at our institution. A sample size of 350 subjects is sufficient to detect a clinically important difference of 0.5 between groups, assuming a standard deviation of 1.195 for a 2-tailed McNemar’s test with 80% power and a 5% level. We included outpatient adults (≥22 years old - to meet software image requirements). Younger patients and exams performed in the emergency room were excluded since acute conditions could be a confusing factor. All relevant ethical approvals from our institution’s Ethics Committee were obtained.
Medium (soft tissue) and low kernel (lung parenchyma) axial image series from selected CT studies were originally stored on our institution’s PACS(SECTRA IDS7®). These series were uploaded to AIRC-cCT via the teamplay digital health platform (Teamplay, Siemens®, Germany), which automatically anonymizes, encrypts and transfers the data.
Patient-related variables considered were age, sex and clinical context. Pathology-related variables were emphysema, lung nodules, thoracic aorta and thoracic spine vertebrae.
For emphysema assessment, AIRC-cCT identifies and quantifies hypodense areas, displaying the affected lung tissue, as a percentage, per lobe and lung volume. We assumed the cut-off of ≥0.5% as it balances the sensitivity and specificity of AIRC-cCT, maximizing the results.
For lung nodules, AIRC-cCT automatically detects, highlights and measures lung nodules. Only the 6 largest of each study were considered and the software analyzes their number, volume, diameter (2D and 3D), type, location plus overall tumor burden.
AIRC-cCT identifies, segments and measures 9 diameters(S1-S9) of thoracic aorta from routine, but we excluded 2 segments: S9 and S1(abdominal aorta and Valsalva sinus, beyond the scope of the present work.14 We decided to follow our local institution’s clinical practice guidelines, due to lack of international consensus. The following thresholds were used: ascending thoracic aorta and arch (S2-S5) ≥40mm and descending thoracic aorta (S6-S8) ≥30mm.
AIRC-cCT measures dorsal vertebrae bodies heights (anterior, medial and posterior) and average HU. It uses a classifying score that summarizes bone alterations (MSK RANGE 1-4), where 1 equals no changes/normal and 4 means most severe changes. We consider abnormal case values of MSK RANGE 2-4. Although this score is user-configurable, we used the default configuration (Figure1).
Expert Panel included two thoracic radiologists from our hospital with over 10 years of experience who evaluated selected exams blinded to the report and software analysis.
For emphysema and thoracic vertebrae assessment, the Expert Panel’s opinion was considered the ground truth. When evaluating lung nodules and thoracic aortic diameter, the Expert Panel evaluated if they were real nodules and if segmentation was correct, respectively.
Statistical analysis of the final data was done with IBM® SPSS® Statistics 28. Sensitivity, specificity, positive and negative predictive values (PPV and NPV respectively) and negative and positive likelihood ratios were calculated to evaluate the effectiveness in detecting emphysema, aortic dilation and thoracic spine. Cohen’s Kappa coefficient was used to assess the findings agreement between the relevant groups. P-value was calculated with the Fisher method and p<0,0001 was used for significance.
Results
Population
The study encompassed 350 patients, with a median age of 65 years, ranging from 24 to 96 years. Of these patients, 199 (56.9%) were male, while 151 were female. Clinical contexts are given in Figure 2, with oncology leading the speciality for Chest CT performance.
Out of the 350 patients, 2 were excluded from the evaluation of emphysema, aortic enlargement and thoracic spine because the slice thickness was outside AIRC-cCT’s image requirements (the software requires a slice thickness ≤ 3mm).
Emphysema
When trying to assess AIRC-cCT’s performance against the Expert Panel, sensitivity was 68.5, specificity was 64.7%, PPV was 52.7%, NPV was 78.1%, Cohen’s kappa was 66.1% (p<0,0001)(Table 1).
Table 1: Emphysema - comparison between AIRC-cCT and Expert Panels. Emphysema considered when ≥0,5% lung volume affected. P<0,0001
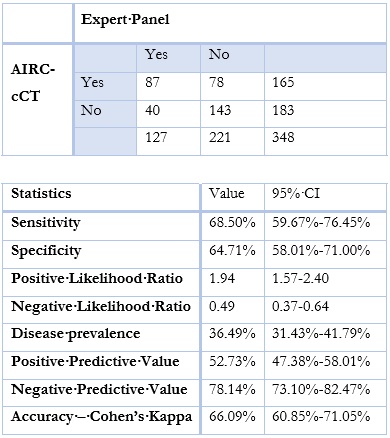
Most patients evaluated (221) did not have emphysema. The most common subtypes were centrilobular trace and paraseptal mild (Figure 3).
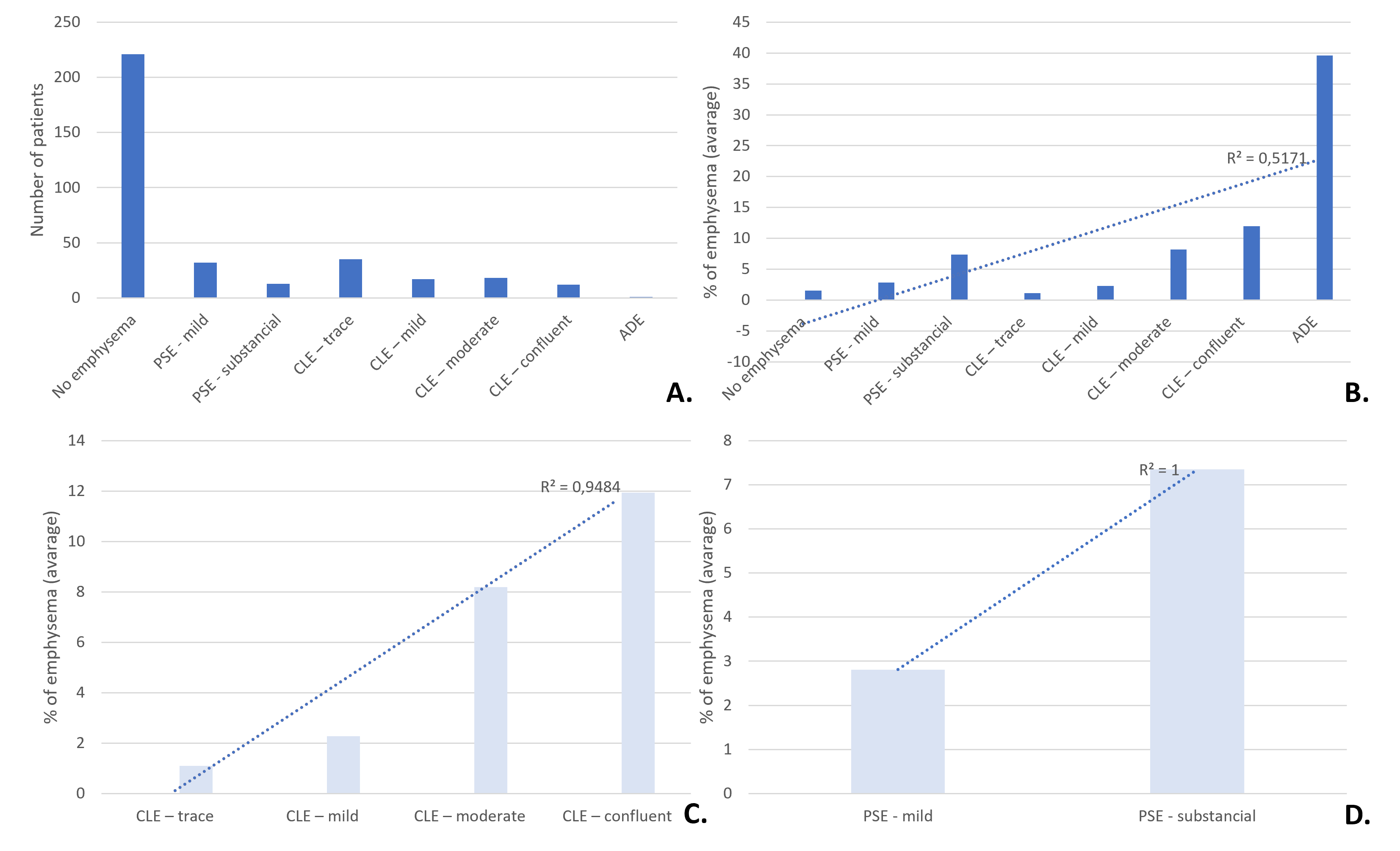
Figure 3: Emphysema type. A. Number of patients classified by the Expert Panel (qualitative classification) with each type of emphysema. B, C and D. Expert Panel’s classification with the corresponding percentage of emphysema on AIRC-cCT. PSE - paraseptal emphysema, CLE - centrilobular emphysema, ADE - advanced destructive emphysema.
There was a positive linear correlation between the degree of emphysema (Expert Panel) and the percentage of emphysema quantified by AIRC-cCT (Figure 3). R2 was 0.52 for overall analysis and close to 1 for PSE and CLE subtypes (Figure 3).
Pulmonary Nodules
Out of 1426 nodules identified by the software, 1003 were included in this work based on the criteria set above (Figure 4).
The Expert Panel regarded 677 (67.5%) real nodules, with a PPV of 46.7%. Out of those, 468 were clinically relevant: 452 solid nodules, 9 subsolid nodules, 4 ground-glass opacities and 3 hamartomas. 209 nodules (20.8%) were considered non-relevant (Table 2).
Aortic Enlargement
AIRC-cCT detected 111 patients with pathological dilation of the thoracic Aorta while Expert Panel considered 109 dilations. Results for sensitivity, specificity, PPV and NPV were 99.1%, 98.7%, 97.3% and 996%, respectively. Cohen’s kappa was 98.9% (p>0,0001) (Table 3).
Thoracic Spine
The program automatically classified 130 (37.4%) patients as abnormal, while only 18 (13.8%) of them were considered clinically relevant changes by the Expert Panel.
Sensitivity was 47.4% and specificity 63.9%. The PPV was 13.9%, the NPV was 90.8% and Cohen’s kappa was 62.1% (p<0,0001) (Table 4).
Discussion
We aim to understand if this tool has a positive clinical impact on imaging departments.
Processing Rate
From an operational perspective, AIRC-cCT has shown a successful processing rate. Lack of compliance with image requirements occurred in only 0.6% of scans (2 out of 350).
It is easily applicable and has wide potential adoption, bearing in mind that the CT scans used were randomly selected and acquired with a non-advanced imaging protocol.
Emphysema
AIRC-cCT was adequately sensitive (68.5%) and specific (64.7%) in detecting emphysema. Although the results were not perfect for a screening test, the possibility of quantifying emphysema represents an advantage.
The correlation was good for centrilobular and paraseptal emphysema. However, results for overall data were poor, since the accuracy in cases without emphysema was suboptimal and this population was included, spoiling the correlation value. AIRC-cCT misinterpreted low-density areas as emphysema, such as bronchial lumen, creating room for improvement of the algorithm.
Emphysema quantification has gained relevance over binary or descriptive categories, as recent evidence suggests a strong correlation between GOLD severity stages of COPD patients.21
Pulmonary Nodules
AIRC-cCT identified 1003 nodules, revealing a moderate capacity to detect real nodules, according to Expert Panel (67.5% were real nodules) and a moderate PPV (46.7%). Since we do not have a low prevalence of nodules in our population, low PPV is not dependent on the sample size. Chances that a positively screened nodule by AIRC-cCT is a relevant nodule are below <50%.
False positives were identified mostly as bronchial impaction, normal vascular structures and atelectasis, likely due to mass-like shape. Protruding osteophytes from thoracic vertebrae in direct contact with the lung parenchyma likely led to their misidentification as nodules.
21% of nodules identified by the software are non-relevant real nodules, therefore, the introduction of anatomic and density criteria may help reduce the number of granulomas and perifissural nodules, which represent the majority of those nodules.
Volumetric analysis is especially useful when measuring nodule growth over baseline, with volume-based measurements leading to 10 times fewer false-positive measurements.8,22 Nonetheless, it is time-consuming and therefore not applied to every chest CT in clinical routine. Having volumetric information available instead of 2D measurements of pulmonary nodules could be beneficial in follow-up examinations, to more accurately measure changes. The high incidence of pulmonary nodules in chest CT studies, encourages AI-based analysis to support high-volume reading workflows, decreasing reading time and would help in the implementation of large-scale lung cancer screening projects.
Aortic Enlargement
Expert Panel and AIRC-cCT strongly agreed about aortic dilation status (Cohen’s kappa = 98.9%, 95% CI 98.1-99.7%) with excellent screening parameters (sensitivity = 99.1%, specificity = 98.7%) representing a highly accurate and reliable test, feasible with or without endovenous contrast agent. The rate of false positives and false negatives was low (1.2% combined; 0.3% FN, 0.9% FP), resulting mostly from wrong measurement and/or segmentation, with the inclusion of nearby structures. These results fit within the available data in the literature for aorta aneurysms.1
Thoracic Spine
For thoracic spine assessment, AIRC-cCT was deemed reasonable, however, the number of false positives suggests that it is not yet fit as a screening tool. The concordance was similarly only mild in strength due to the rate of false positives (Cohen’s kappa = 62.1%). Mis-segmentation or measurement errors by the software contributed to this observation, along with a systematic mis-segmentation of S1. While our findings suggest potential for AI-driven bone density assessment via CT, we acknowledge the need for further refinement.
Other groups have reported success in the implementation of AI prototypes aimed at determining bone density via CT. Nam et al’s model showed 88% accuracy in classifying vertebrae as osteoporotic or non-osteoporotic.1
Limitations
Results cannot be extrapolated for other AI software.
The continuous lack of consensus in defining limits for what is considered pathological or not (e.g., what is the threshold for clinically significant solitary nodules, aortic dilation or spinal changes) is a hindrance for AIRC-cCT, because it relies upon clearly defined values to separate what is a relevant finding from what is not. In contrast, Radiologists rely on their own and their peers’ perceptions/experiences to make judgments and consider clinical context, giving them an edge in clinical decision-making.
As an advantage, AIRC-cCT settings can be adjusted to fit within the Radiologists’ defined limits to separate relevant from non-relevant findings, which would translate to lower rates of false negatives and higher Radiologist-Software decision agreement. We deliberately chose to keep AIRC-cCT settings as factory default to prevent a positive bias and to reproduce a first-time user experience. Nonetheless, we recommend and stress the utility of adjusting AIRC-cCT settings to the user’s institutional practice/guidelines.
In lung nodules assessment, one limitation of our study was the focus on detected nodules, without considering nodules AIRC-cCT missed.
Lastly, we must bear in mind that, like any other currently available method, AI-powered medical imaging analysis software is only meant to be used as an assistant/second opinion support tool for an experienced Radiologist, to enhance the reporting performance.
Conclusion
Our findings suggest that AIRC-cCT offers an innovative approach to developing diagnostic algorithms that have the potential to aid the diagnosis and differentiation of diseases affecting the chest.
The AIRC-cCT algorithm demonstrated exceptional accuracy in detecting aortic dilation, with a strong agreement and outstanding screening parameters. Its low rates of false positives and false negatives highlight its reliability. This outstanding performance, particularly in aortic enlargement evaluation, positions AIRC-cCT as a highly effective and clinically valuable tool for aortic health assessments.
To our knowledge, this is the first study to evaluate the performance and predictive power of AI software compared with an Expert Panel, for multiple variables simultaneously. Further studies are needed to minimize false results and optimize accuracy.