1.Introduction
In this era of Big Data where the old adage of ‘bigger is better’ is championed, the small-scale sample sizes of the focus group method may be seen as a limitation to the generalisability of findings. This comparison of scale has become more and more evident as increasingly larger amounts of data are being generated every second. It has led some to speculate that qualitative research may be losing its value (Christensen, 2015) in a world where even such immeasurable concepts as emotions and stress are becoming quantified and translated into data to be analysed and understood (Cambria, 2016; Garcia-Ceja, Osmani, & Mayora-Ibarra, 2015). In market research, this has manifested in speculations and proclamations of ‘the death of focus groups’, which has grown in the last decade.
Qualitative research still has immense value, however, and no matter the size and scale of Big Data, numbers alone are still not enough to truly understand the whys of the world (Agius, 2013). In recent years, there have also been an increasing number of attempts to scale up qualitative research (Hunt, Moloney, & Fazio, 2011; Neale & Bishop, 2012; Fontaine, Baker, Zaghloul, & Carlson, 2020; Rolf, Campbell, Thompson, & Argus, 2021). However, these attempts, which may be collectively identified as ‘Big Qual’, appear to be labour intensive at either data collection or analysis, or even both. While the exchange of depth of insight for longer data collection periods is a common enough occurrence in qualitative research, it is likely to only become more burdensome as the scale of future studies increases.
This paper explores the use of a methodology to scale up focus group discussions (FGD) online, as an alternative to several regular-sized FGDs over a prolonged period. The objective is to determine if this methodology would be an adequate replacement for FGDs, as it aims to gather qualitative data at scale and in-the-moment, whilst still maintaining or coming close to the depth of insight of FGDs.
As the fascination with mass amounts of data shows no signs of abating (Marr, 2015; Ghia, Langstaff, Ware, & Wavra 2021), there is a need to continue to innovate qualitative research methods to evolve with the changing landscape of today. Adapting tried-and-true methods such as the focus group to meet the demands of the new age is one of the ways this can be done.
2.Literature Review
2.1 Big Data and the Death of the Focus Group
Big Data can be simply understood as the results of the datafication phenomenon (Mayer-Schönberger & Cukier, 2013). This “sense-making” (Lycett, 2013) phenomenon, where an increasing number of devices, sensors, applications, and more, are being used to track various data points, from which researchers and data analysts can interpret meaning, shows no signs of slowing down (Cambria, 2016; Garcia-Ceja, Osmani, & Mayora-Ibarra, 2015).
Rather, it would seem to be picking up speed, increasingly quantifying notions like social interactions and human behaviours (Narayanan & Georgiou, 2013; Mimura, Kishino, Karino, Nitta, Senoo, Ikegami, Kunikata, Yamanouchi, Nakamura, Sato, & Koshiba, 2015; Kolonin, 2018). Where before the understanding of the subtleties and nuances of such concepts were solidly within the domain of the qualitative researcher to provide, the advent of increased datafication begs the question - what value does qualitative research have in this age of Big Data? (Strong, 2013).
The attraction of Big Data seems especially evident in the field of market research. In an environment where practitioners strive to gather insight into consumers as quickly and accurately as possible before their competitors, it is easy to see a “widespread belief that large data sets offer a higher form of intelligence and knowledge that can generate insights that were previously impossible, with the aura of truth, objectivity, and accuracy” (Boyd & Crawford, 2012, p. 2).
Labourious methods such as focus groups may no longer be preferred when there is an increasing plethora of digital tools leveraging on the mass amounts of data being generated in real time (Guinee, 2016; Valentine 2021, Mghames, 2021; Wonderflow, 2021; Hoick, 2023). Using data mining and sentiment analysis to analyse consumers in the process of consumption (Gao, Wang, Luan, & Chua, 2014), rather than asking them to reflect and share their processes afterwards would be a more accurate picture of their thoughts, and it can be done quickly, accurately, and at scale. It has even led some to speculate on the death of the focus group (Christensen, 2015; Mghames, 2021).
However, other practitioners believe focus groups remain a valuable research methodology (Whitaker, 2021; Ramsdale, 2021). The chief value of focus groups over the newer methodologies leveraging on Big Data, is the ability to dive deeper into a topic and glean a fuller, more nuanced understanding. The interactive nature (Almutrafi, 2019; Gundumogula, 2020) of focus groups remains a key and unique characteristic which often leads to sparks of insights. Furthermore, the role of the moderator is not simply to ask questions and receive responses, but to go further and deviate from expected responses by reading between the lines of the responses, through observations of individual behaviours, group dynamics, and other non-verbal cues. It is clear then, that focus groups are not dead, per se, but are simply one of many tools available to researchers, albeit one that some perceive as having a somewhat limited efficacy, due to its comparatively much smaller scale.
2.2 Qual Goes Big
There have been attempts to scale up qualitative research. The resulting studies come under the umbrella of ‘Big Qual’. ‘Big Qual’ does not refer to a singular methodology, but rather describes methodologies which are either purely qualitative or mixed, with a sample size that is significantly larger than what is typical of qualitative research. Specifically, it has been defined as “qualitative research with data sets containing either primary or secondary qualitative data from at least 100 participants” (Brower, Jones, Osborne-Lampkin, Hu, & Park-Gaghan, 2019).
The larger sample size of Big Qual affords greater breadth in understanding the research topic, on top of the level of depth in insights which define qualitative research. To maintain the depth of qualitative inquiry whilst expanding its breadth to match that of quantitative research is an alluring concept, though not without its challenges.
Qualitative research is characterised by the depth of insight gained through data collection and analysis (Agius, 2013). It is typically gained through a laborious period of data collection, and an equally time-intensive period of analysis. Efforts to scale up appear to have a correspondingly scaled up amount of labour required to conduct such studies, taking place over months or even years (Hunt, Moloney, & Fazio, 2011; Rolf, Campbell, Thompson, & Argus, 2021; Scantlebury & Adamson, 2022). This holds true regardless of the methodology employed: interviews, focus groups, content analysis of secondary data, or some combination thereof.
Beyond the challenge of long durations for data collection and analysis, the methodologies themselves pose some limitations, from both a research and operational perspective. Interviews, “so embedded in the qualitative landscape that it is arguably naturalized” (Brinkmann, 2013), are individual, singular, time consuming and resource intensive. Focus groups share some similar limitations, particularly in that it may be even more difficult to coordinate several people to attend one focus group, let alone to arrange several. Additionally, though focus groups afford a relatively larger scale compared to interviews, researchers have the challenge of managing group dynamics, including the challenge of social desirability bias (Bergen & Labonté, 2019). In the case of content analysis of secondary data, it may be labour intensive to source and combine such data sets, and further, as the data was not collected for the express purpose of the study, the scope of research and analysis may be limited (Fontaine, Baker, Zaghloul, & Carlson, 2020; Davidson, Edwards, Jamieson, & Weller, 2018).
Still, there is potential to innovate qualitative data collection methods through scaling up. This is particularly so for focus groups, which have seen relatively fewer attempts to scale up compared to interviews and content analysis. This is especially so given new advancements in artificial intelligence and its capability to process large-scale amounts of data in an instant. To be able to apply such technology to focus groups, particularly in a manner which allows respondents to interact with each other, would indeed be a game-changer in conducting large-scale qualitative research.
3.Methodology
This assessment comprises two studies: a study using traditional FGDs and a pilot study which studied a similar topic using an online, at-scale focus group methodology. Though conducted independent of each other, having two studies with similar topics allowed better comparisons of the insights derived from both methodologies, as well as the advantages or limitations of each methodology in comparison with one another.
3.1 A Note on Saturation
Saturation is a commonly used criterion in determining both sample size and data analysis from a qualitative perspective. It can be defined as:
“Bringing [in] new participants continually into the study until the data set is complete, as indicated by data replication or redundancy. In other words, saturation is reached when the researcher gathers data to the point of diminishing returns, when nothing new is being added”. (Bowen, 2008, p.4)
As a market research agency, studies are typically conducted within a short timeline. Hence, it is particularly critical to have a decently robust and representative sample of respondents for each study before fieldwork, as there is little room to allow fieldwork to run-on “to the point of diminishing returns”. It is also imperative that studies are conducted with the optimum sample size to ensure that as much data as possible is gathered within the short time frame, to ultimately achieve saturation.
A typical study would consist of 6 focus groups, 8 to 10 participants each, for a total of 48 to 60 respondents per study. Research on saturation in the context of sample size supports these numbers as sufficient to achieve saturation (Krueger & Casey, 2015; Guest, Namey, & McKenna, 2017). Based on this number, it was determined that a conservative, manageable sample size of at least n=100 respondents would be sufficient for fieldwork for the pilot study with the new methodology.
3.2 Understanding the AI-Enhanced Platform: Digital Conversations @ Scale and How It Works
For ease of reference, the methodology used for the pilot study will be referred to as ‘Digital Conversations @ Scale’ or ‘DC@S’. The topic of the study was on the progress of women and challenges they face in Singapore.
For this study, a total of 103 self-selected respondents through purposive sampling via an external recruitment agency were recruited, aged between 21 and 49 years old, with a mix of education levels, from some secondary school education to postgraduate degrees. All respondents were also required to have internet access for the duration of the discussion.
On the day of the discussion, respondents logged on to the discussion platform and answered a series of demographic questions before the discussion began. The main online moderator, with the help of an assistant moderator, took all 103 respondents through the 1.5-hour discussion, using a pre-designed list of questions, reminiscent of the discussion guide of a focus group.
After this session, 16 respondents participated in a 1-hour online feedback session to understand their experience with the DC@S methodology. The participants were separated into two groups of 8, based on their gender.
3.2.1 Examining the Interaction Between Respondents
Each question was sent in individually as the discussion progressed, and respondents were given a fixed amount of time per question to type in their replies. If they submitted their answer before the timer ran out, they were then asked to vote on others’ submitted responses in the remaining time.
Respondents were to either a) agree or disagree on a single response from another participant, or b) choose which of two responses they preferred. The responses shown were selected entirely at random, with no influence from either moderator.
In Figure 1, the respondent has typed their reply to the question of “What areas are they [women] currently struggling in?” and is now being asked if they agree or disagree with another respondent’s reply.
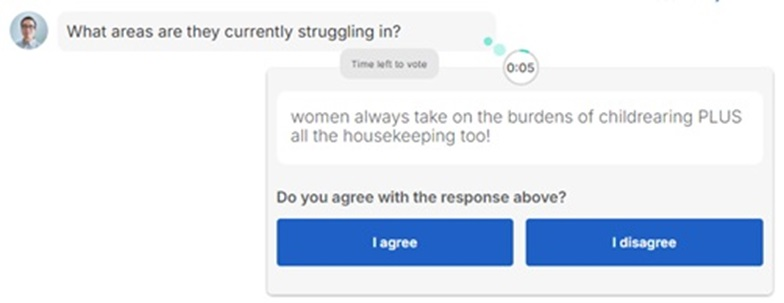
Figure 1 Question “What areas are they [women] currently struggling in?”, from the viewpoint of a respondent on a desktop.
In Figure 2, the respondent has submitted their reply to the question of “What about work-life balance are they [women] struggling in, and what support is lacking?” and is now being asked to choose which of two other respondents’ replies to this question they prefer. In Figure 3, the same respondent has clicked on “More options” and is given 4 more options on how they would prefer to reply to the question, allowing for a greater diversity of responses.
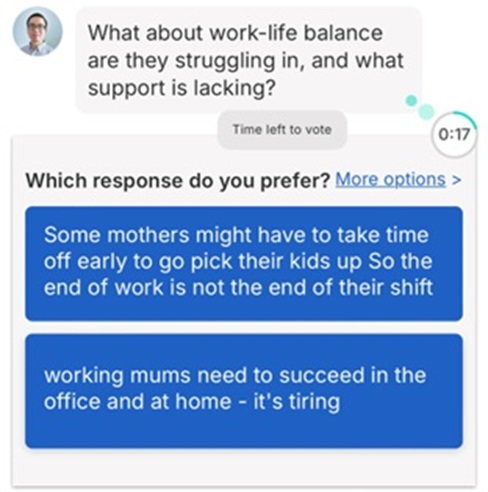
Figure 2 Responses to the question: “What about work-life balance are they struggling in, and what support is lacking?”, from the viewpoint of a respondent on a smartphone.
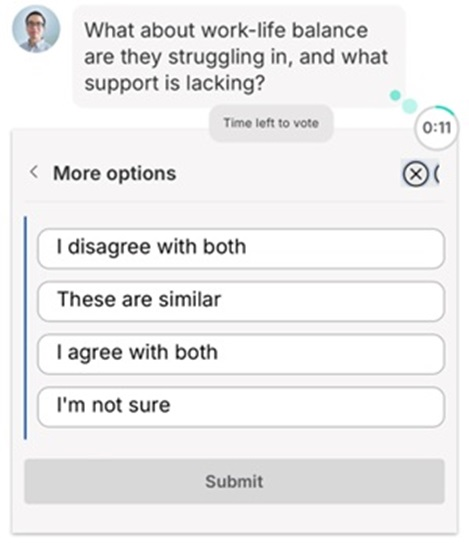
Figure 3 More options to respond to the question: “What about work-life balance are they struggling in, and what support is lacking?”, from the viewpoint of a respondent on a smartphone.
3.2.2 Real-Time Feedback, Ability to Pivot the Line of Questioning
During the discussion, the platform’s AI provided instant analyses of the respondents’ replies to the moderators, pulling out keywords, in Figure 4, and highlighting the most agreed upon responses, in Figure 5. This simulates the role played by a moderator in a more traditional focus group to allow for further probing of thematic responses.
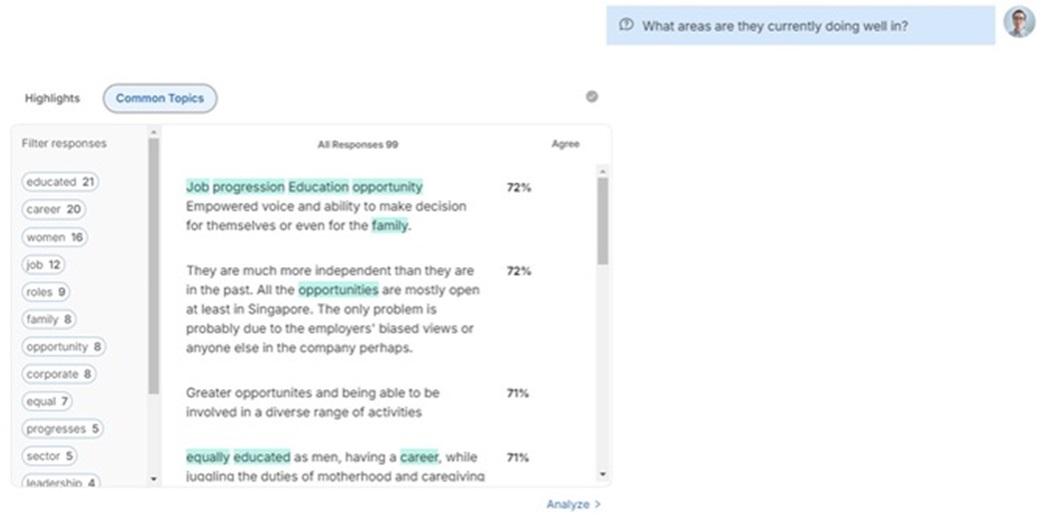
Figure 4 Keywords from responses to the question: “What areas are they [women] currently doing well in?”, from the viewpoint of a moderator on a desktop.
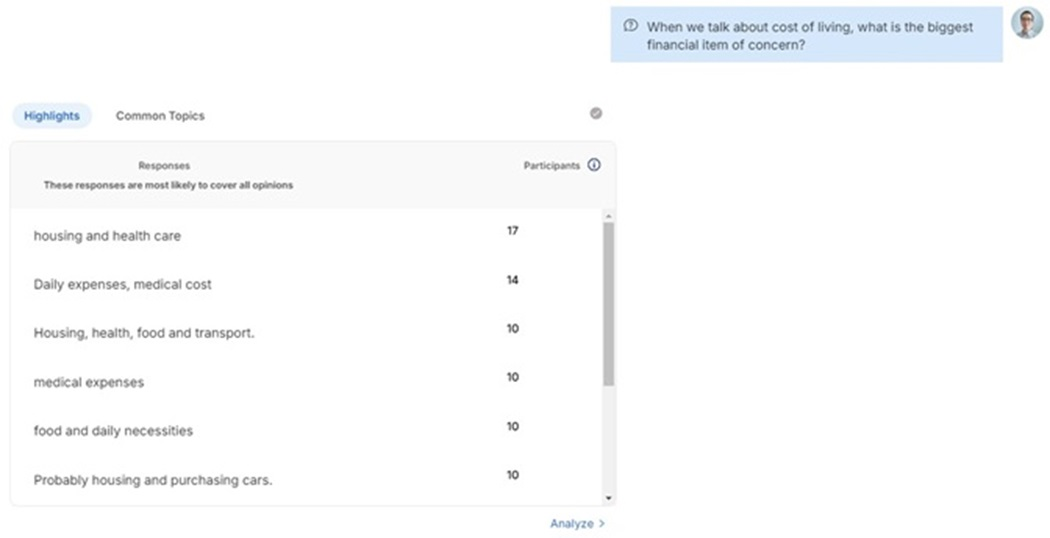
Figure 5 Response highlights to the question: “What areas are they currently struggling in?”, from the viewpoint of a moderator on a desktop.
Based on these analyses, the moderators could also send in additional questions at their own discretion to follow up or probe respondents further. All respondents were required to submit an answer to these additional questions.
This ability to adapt the discussion guide based on responses during the session, whether by adding new questions, changing the sequence in which questions would be sent out or even omitting questions entirely, mimics an essential characteristic of traditional qualitative methodologies such as focus groups or interviews.
Observers of the discussion were able to see the same analyses as the moderators, however they were not able to send in additional questions. The respondents were also able to see some quick analyses provided by the platform’s AI, though this analysis was largely at a superficial level.
3.3 In Comparison: Focus Group Discussions (FGDs)
To assess the effectiveness of the DC@S pilot study as a method to gather thick data and deep insights quickly, the findings were compared with a previous study with a similar topic, the development of women in Singapore. It is important to note that this study had been conducted completely independent of the DC@S pilot study.
For this study, five 2-hour FGDs over 3 days were conducted. Three of the groups had all-female respondents, and the remaining 2 had all-male respondents. All 5 groups had seven respondents for a total of 35 respondents, segregated by age and education. The respondents were aged 23 to 59 years old, and their education levels ranged from having some secondary school education to postgraduate degrees. Respondents were recruited through several independent external recruiters, using purposive sampling for each group. The moderator for each group was of the same gender as the respondents.
The moderator would lead the respondents through a 2-hour discussion with a pre-designed discussion guide, though he or she would also use ad lib probing questions to elicit deeper responses. Such ad lib questions were used at the moderator’s discretion and may not have been used in all five groups. The room where the discussions took place was adjoined to an observation room for observers to watch and listen to the discussion in real-time.
4.Findings
Overall, while the DC@S methodology achieved a level of saturation comparative to a series of FGDs, it does not appear to be able to replace focus groups as a methodology entirely. In addition to achieving saturation, it allows for some interactivity between respondents, and is useful in collecting large-scale qualitative data in a short period. It would likely be particularly useful in gathering rich insights from a large group of people who are geographically disparate, for example.
In comparison, in-person focus groups afford moderators the ability to ladder on individual’s responses further, something that is limited in DC@S, as well as allow researchers to observe participants’ non-verbal cues, adding layers of meaning to what is being said. These points will be illustrated further in the discussion below.
5.Discussion
5.1 Depth of Insight: Able to Achieve Similar Levels of Saturation to a Series of FGDs
While FGDs achieve a higher granularity of insights through layered and more targeted questioning, DC@S generates more rapid generalisability of findings with incidence rates and a more quantitative representation of respondents’ opinions.
Illustrated below are a comparison of similar questions from the DC@S study and from the FGD study. For the DC@S study, question 13 “What areas are women currently struggling in?” and the follow up probe, question 13a “What about work-life balance are they struggling in, and what support is lacking?”, is compared to a similar question asked in the focus groups, “What do you feel are the top 3 issues affecting the development of Singaporean women that needs to be addressed?”
Respondents’ written responses were analysed by the platform’s AI, produces instant visualisation of the responses for the moderator. These insights provided a summary of the large volume of responses and helped the moderator craft better, smarter probes in the moment.
Table 1 Top 10 Responses to Question 13 “What areas are women currently struggling in?” (Participants)1
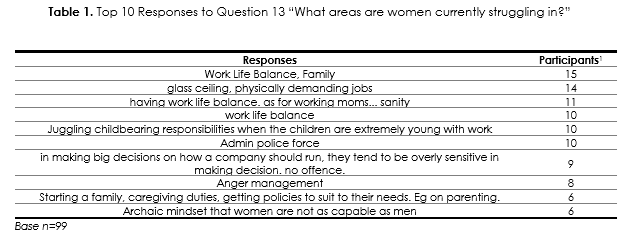
Table 2 Top 5 Responses to Question 13(a) “What about work-life balance are they struggling in, and what support is lacking?”
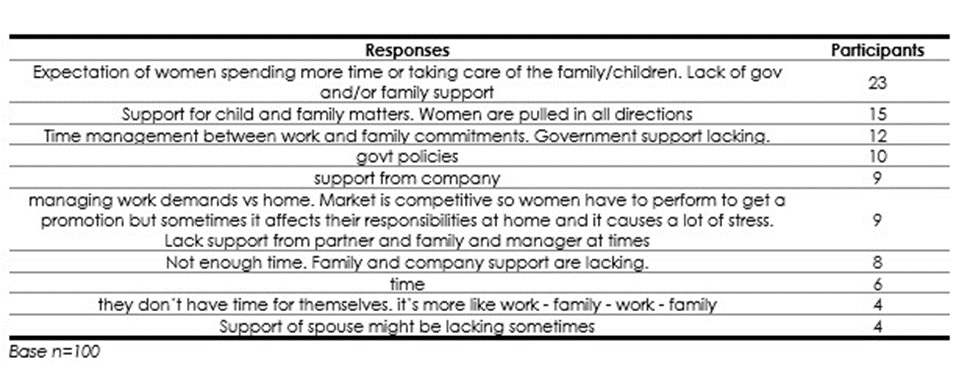
In Table 2, work-life balance emerged as the most common topic in response to Question 13, “What areas are women currently struggling in?”, based on the respondents’ agreement scores. With this instant visualisation, generated by the AI and based on the respondents’ real-time input, the moderator was then able to follow up with the probe, Question 13(a), “What about work-life balance are they struggling in, and what support is lacking?”, to elicit deeper insights. The instant analyses effectively bolster qualitative researchers’ organic moderation ability.
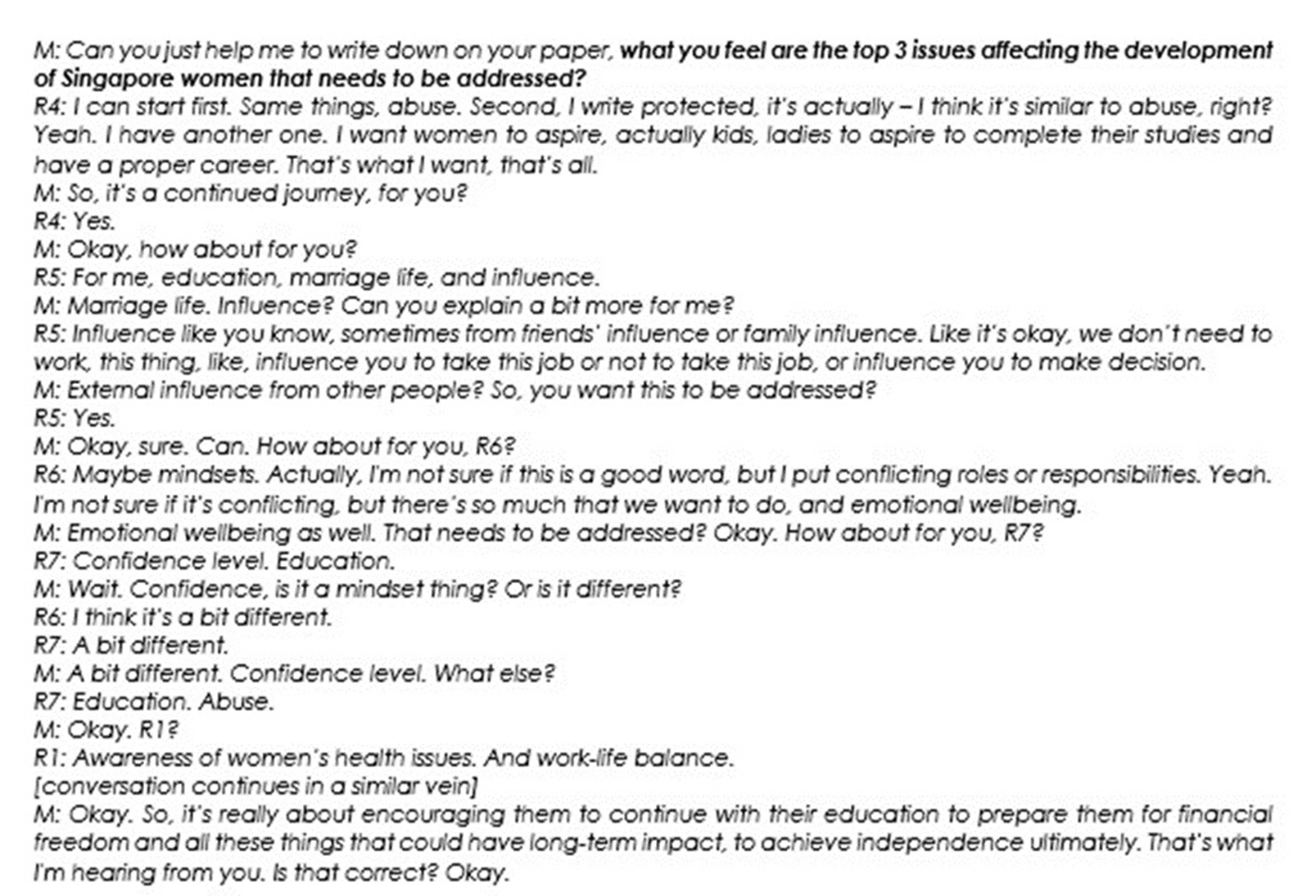
Figure 6 Excerpt from a focus group, on the topic of issues affecting women’s development in Singapore
In Figure 6, the respondents, R1, R4, R5, R6 & R7, were asked a similar question to Question 13 in the DC@S study. After some discussion, the moderator, M, provides a summary of issues that respondents raised.
While the moderator was able to accurately summarise the issues, this is dependent on their capability and expertise (Almutrafi, 2019), and it is possible that they may incorrectly highlight an issue as part of the respondents’ collective top 3. In comparison, the responses from the DC@S study shown in Tables 1 and 2 show more accurately the issues which were most salient and why.
5.2 Simulating Interactivity: Mimicking Real-World Interactions
A key, unique characteristic of FGDs are the interactions between respondents, which often lead to sparks of insights which may not have been achieved through a one-on-one interview between a single respondent and interviewer. It also tends to lead to achieving a consensus between respondents on an issue, thus strengthening that particular insight. Without such interactivity, it would be difficult to truly assess any method as an adequate replacement for FGDs. Hence it was necessary to replicate or at least simulate such interactivity between respondents during the discussion to allow a possibility of sparking ideas as well as consensus building.
The previously described feature of the platform, where respondents are asked to ‘react’ to others’ responses, was found to be a satisfactory simulation of an in-person FGD’s interactivity. It showed respondents the thoughts of others, thus possibly inspiring their subsequent thinking and responses, as well as allowing for consensus building through asking for their agreement to or preference between others’ responses.
Additionally, moderators often report a sense of social desirability from the respondents after a focus group, noting that some respondents may have been holding back in their responses, at specific moments or even throughout a group, to avoid potentially offending others or singling themselves out as an outlier. This is understandable given the intimacy of being in an enclosed room with strangers for a certain duration.
It appears that this issue of social desirability may be alleviated in the DC@S methodology, possibly due to the nature and limitation of interaction allowed between respondents during the discussion. While each respondent is able to see the whole group’s demographic and individual respondents’ answers to questions, as they are pushed to them, the responses are not tagged or identifiable to any other respondent. This effectively allows them to react only to what that other respondent has typed, and uncoloured by any biases they may have towards that respondents’ demographic profile, as seen in Figure 7.
5.3 Scaling Up and Optimising Efficiency
DC@S allows for a live conversation with a more robust sample size that engenders the ability to quickly capture a larger number of responses in real time and in respondents' own words.
It effectively engenders a similar breadth of insights akin to the saturation point achieved in 5 FGDs, within only 1.5 hours of fieldwork, compared to a total of 10 hours for FGDs. This indicates the efficiencies of time saved and manpower involved in gathering qualitative data at scale within the same duration of a single FGD. This allowed us to achieve rapid results that would aid in our reporting.
6.Limitations
6.1 Individual Limitations
6.1.1 Miscategorisation
The online platform’s AI-generated instant analyses utilise an algorithmic logic to probe responses in a manner that is more standardised and structured compared to the organic moderation capability of a qualitative researcher. As discussed above, this was a boon to the moderator. However, it is not perfect, and there are some significant incidences of miscategorisation of responses.
In Table 3, the AI has pulled the word “women” as a topic of interest from the responses. However, this categorisation of “women” as a topic of interest is flawed as the question was on women’s progress, and thus it is only logical to include mentions of women in responses. This is evident when compared to a manual coding of the responses, which found that “opportunities” was instead the most common topic for that question.
Table 3 Responses to Question 7 “What has impeded progress for women in Singapore in the past 10 years?”(Count) 2
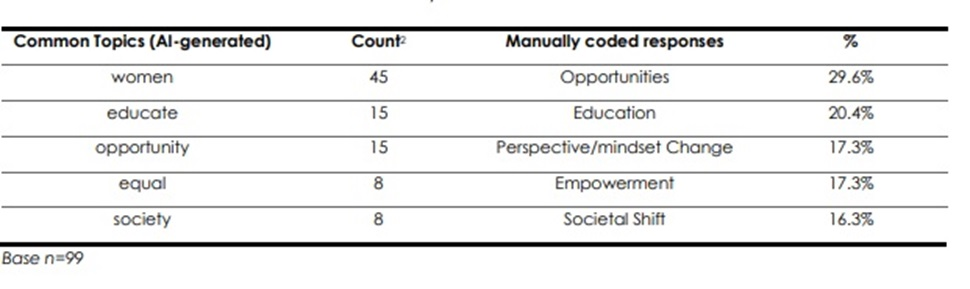
At present, the AI appears to lack the sophistication needed to recognise more complex topics of interest, for example, phrases instead of single words, as well as synonyms or abbreviations. This presents an issue as the auto-generated data, while useful at a glance during the discussion proper, would contain flawed data and hence still require cleaning post-data collection in order to be useful.
6.1.2 Labour Intensive Analysis
While it is possible to manually process and clean the raw data from the online discussion, this would be a massively time-consuming process due to the sheer volume of unstructured data produced. Hence, rather than aiding researchers, this labour-intensive process would be a hindrance, especially with regards to open-ended responses.
6.1.3 Reach
Online research allows for greater reach to respondents who are unable to attend live sessions. However, it is a double-edged sword, as it is not suitable for everyone.
Feedback from respondents of the DC@S study, in Figure 8, included complaints that they were not able to finish typing in their responses during the time limit given.
Some also misunderstood the questions or the voting exercise following the submission of their response. Thus, while this methodology may be accessible to those younger or more digitally savvy, it may be more challenging for other demographics such as senior citizens or those less digitally savvy, to be effectively reached or engaged on a digital platform, as would persons with limited to no access to the internet or an internet-enabled device.
6.2Limitations in Comparison to FGDs
6.2.1 Lacks Granular Responses
While the DC@S methodology is able to capture a wide breadth of responses, and the responses have more depth compared to a purely quantitative survey, in comparison to an FGD, the responses lack granularity and texture.
Though respondents can be asked questions based on their previous responses, this “branching” is only possible to one degree. This prevented the moderator from simulating techniques that would typically be used in a FGD to uncover deeper responses and insight, such as “laddering”, shown in Figure 9. This kept responses largely at surface level unless respondents themselves are effusive in their responses.
In DC@S, additional questions will also be seen by and require all respondents to reply to and participate in the subsequent voting exercise. While this captures a wider breadth of responses, it also restricts the moderator to broader questions, and does not allow for targeted questioning of certain responses.
6.2.2 Limited Interactions
While respondents have some interaction with each other through the voting exercises, it is limited in comparison to an in-person FGD, where interactions are freer and more natural.
The respondents are unable to spontaneously “speak” with the moderator. As the moderators send in questions that respondents must respond to, the discussion leans more to a one-way question-and-response rather than a true discussion. This could prove particularly troubling if respondents do not understand the question sent in, as they would not be able to clarify with the moderator or any other respondents.
Additionally, if they felt they were not able to contribute to the discussion and chose not to reply to the question, as in Figure 10, this may skew the data and affect the overall analysis.

Figure 10 Excerpt from the online feedback session, on respondents choosing not to answer a question
6.2.3 Does Not Capture Non-verbal Responses
Additionally, as it is an online platform, respondents’ non-verbal cues, including body language, facial expressions, tone, volume, and other observational data are not captured, which is another avenue of data collection that this methodology misses out on.
7.Final Considerations
The pilot study is a successful attempt at efficiently gathering large amounts of qualitative data within a short period of time. However, it is not currently able to achieve the same level of nuance and depth traditional FGDs provide, and hence cannot fully replace FGDs. Still, it may add value to specific use cases such as large-scale engagement studies which seeks to achieve wide breadth with some depth in the responses.
This particular method of DC@S can also be explored further:
•With a larger sample size
•In comparison to a purely quantitative online survey - to better understand the depth that this method can provide
•To conduct a DC@S study in conjunction with an FGD study with the same topic and discussion guide - given that the two studies compared in this paper were conducted independent of each other, it would be beneficial to a further understanding of this method’s capabilities to do a more direct comparison.