Background
Video capsule endoscopy (VCE) appears as a reliable, safe, and non-invasive diagnostic method [1], which revolutionized the definition, diagnosis, and management of obscure gastrointestinal bleeding (OGIB) [2-4]. This method consists in swallowing a non-reusable wireless capsule with a miniaturized camera that travels through the gastrointestinal tract. The camera captures 50,000-60,000 images, uploaded in a video with about 8-12 h [5, 6]. All endoscopic capsules developed so far have similar components, but each individual VCE system has distinct specifications such as resolution and image capture, transmission, and video duration [7, 8]. There is no agreement in the literature about the best system, so the choice of VCE software depends on the operator/institution.
Despite the contribution to the diagnosis of small bowel (SB) diseases (OGIB, Crohn’s disease or familial polyposis syndromes) [2-4, 9], VCE has several limitations: absence of movement and camera orientation control, which allows blind SB areas, difficulty in locating lesions within SB, lack of specificity, inability to perform endoscopic therapy, and image interpretation is physician dependent [8, 10]. There is great heterogeneity concerning image reading, namely in the mode and speed of visualization [6, 11]. It takes 45-90 min to evaluate a VCE, and studies show that faster readings decrease the detection rate [6]. To date, there is no gold standard method, contributing to interobserver variability.
Digestive hemorrhage from the SB occurs with an estimated prevalence of 5-10% [3]. For OGIB, diagnostic yield for VCE ranges between 38 and 83% [12]. The most prevalent etiology in patients older than 40 years is angioectasias [3]. These degenerative lesions of previously healthy blood vessels account for up to 8% of all episodes of gastrointestinal tract bleeding [13]. Zheng et al. [6] revealed a disappointing detection rate of angioectasias by physicians. Though only 69% of angioectasias were detected, this value was significantly higher than for other SB lesions.
Computational methods have been implemented in the reviewing software of the VCE devices to improve diagnostic yield and time efficiency [14].
Most commercially available tools are integrated in the RAPID reader workstation (Given Imaging®). One of the first methods developed was suspected blood indicator, which has been criticized for its suboptimal performance, even for patients with active intestinal bleeding [15-16]. VCE technology has been further expanded with the incorporation of Flexible Spectral Imaging Color Enhancement (FICE) and the Blue Filter (BF) modes [17]. However, it is currently controversial whether virtual chromoendoscopy may increase the diagnostic accuracy of VCE [18]. TOP100, which automatically select 100 frames of interest, was incorporated in the late version of RAPID® software. For an overall detection of significant lesions, TOP 100 identified 76.3% lesions with a sensitivity of 51.28% [19]. Despite recent improvements, these methods still display limitations.
Regarding MiroCam® software (IntroMedic, Korea), studies are scarce, and the tools are limited. The Augmented Live-Body Image Color-Spectrum Enhancement (ALICE) chromoendoscopy system was able to enhance the visibility of angioectasias [20]. On contrary, another chromoendoscopy system that enhances the image in 3 color modes (CM1, CM2, and CM3) was not useful to improve evaluation and characterization of any of the elementary lesions [21].
Currently, several groups are looking to develop novel tools to automatically detect significant lesions in VCE, though only a few deal with the detection of angioectasias [14, 22]. Texture [23] and color [24, 25] extraction algorithms are usually used as feature sources of classifiers. Regarding color, angioectasias seem to have a better characterization in the CIELab than in the red, green, blue (RGB) color spaces [25]. In this line of thought, we worked with the Center for MicroElectroMechanics Systems (CMEMS-Uminho) to develop a novel algorithm to identify angioectasias in SB [26].
The CMEMS-Uminho algorithm is the first to approach the transformation of the image according to the CIELab color system and to add a segmentation process. When compared to the methods already published to detect angioectasias in VCE static frames, the proposed system shows a better performance: sensitivity of 95.32%, specificity of 94.75% and ROC area of 97.87% [26]. Even though these results are promising, as in other algorithms previously described, it demands realistic experimentation.
Therefore, we aimed to evaluate our algorithm overall performance using full-length VCE video, assess its diagnostic yield in clinical practice and impact on physician’s performance.
Methods
The CMEMS-Uminho Algorithm
The algorithm developed at the CMEMS-Uminho, in University of Minho Engineering School, summarily consists in the transformation of the image according to the CIELab color system which has three parameters: L represents the brightness variation between black (0) and white (100); the components a and b represent the color opponent dimensions, where a sets the magenta color to positive values and the green color to negative values, and b sets the yellow color to positive values and the blue color to negative values. This model is more uniform and its components have a less degree of correlation than the RGB System [27]. Angioectasias usually have a red color, so they will be highlighted in component a. As the length of the diameter increases, the possibility of bleeding and the rate of detection by CIELab color system also increases (Fig. 1).
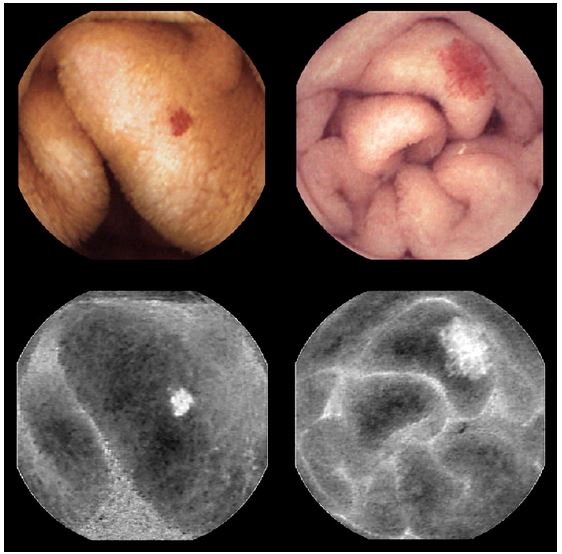
Fig. 1. Original frames of angioectasias with different diameters (above) and the component a of the same lesions (below).
Afterwards, the histogram of the component a is divided into two sections, where the one with the higher values is considered as the region with the lesion (angioectasia). This segmentation of the image is done using a method called expectation-maximization [24], where the parameters of both distributions are estimated in an iterative manner. The extraction of the characteristics is obtained based on histogram measures, namely the mean and variance of the two-color components (a and b) from both selected regions. The process of segmentation of the images allows a standardization of the color spaces, finding one that is complete enough to be able to define all the existing colors [28, 29].
The classification of the frame as normal or abnormal is done using supervised learning methods, in this case a multilayer perceptron [27], which is a specific type of a neural network.
Algorithm accuracy was previously attained with static images from PillCam® (Given, Israel) [26].
Overall Performance of the CMEMS-Uminho Algorithm
Algorithm overall performance was determined using 54 fulllength VCE videos (39 PillCam® [Given, Israel] and 15 MiroCam® [IntroMedic, Korea]) with SB angioectasias, previously identified by an expert capsule endoscopist at the Gastroenterology Department of Braga Hospital.
VCE videos were downloaded from the original software, so the CMEMS-Uminho algorithm could be applied separately from the vendors’ software. For each VCE, the algorithm provided a smaller video consisting of the grouping of frames selected. One frame automatically tagged by the algorithm and one selected by the expert had the same time code, thus the overall performance of the CMEMS-Uminho algorithm was defined by a positive concordance between a frame selected by the algorithm and the expert.
Clinical Performance of CMEMS-Uminho and the Impact on Physicians
To assess the usability of the CMEMS-Uminho algorithm in clinical practice, VCE recordings with SB angioectasias consecutively performed (2017-2018) in the Department of Gastroenterology of Hospital of Braga were evaluated, a total of 41 VCE videos. Videos with blood and poor preparation were excluded. VCE procedures were performed according to a standardized protocol: clear liquid diet the day prior to VCE plus a 12-h fasting protocol. The recording device was set in the morning and removed in the late afternoon. Patients were allowed to drink fluids after 2 h and to have a light meal after 4 h.
All VCE recordings were firstly interpreted by an expert capsule endoscopist (experience around 500 capsules procedures; physician 1), who proposed the clinical diagnosis of angioectasias and also registered the anatomic landmarks, the clinically significant lesions, timing and speed viewing, and quality of SB cleansing. Only lesions in the SB were considered (those found in between the pylorus and the ileocecal valve as defined by the first duodenal and first colonic video frames, respectively).
Posteriorly, two gastroenterology trainees with different VCE reading experience (50 and 10 capsules procedures; physician 2 and 3, respectively) were asked to review the VCE recordings and to register the data described above for physician 1. The SingleView mode of VCE was defined for all physicians. Each physician reviewed each VCE only once and without knowing the results from either one of the other physicians and before applying the algorithm.
VCE videos were downloaded from the original software. The CMEMS-Uminho algorithm was applied separately from the vendors’ software and provided a smaller video consisting of the grouping of frames selected. All images tagged by the algorithm and physicians were reviewed by an independent capsule endoscopist (experience around 150 CE) that confirmed if the lesion selected was an angioectasia and evaluated the concordance between them.
We compared physicians’ performance, regarding global detection rate (total number of angioectasias detected), detection rate per capsule (number of angioectasias tagged in each VCE), diagnostic yield (number of VCE with clinical diagnosis of angioectasias), true positive rate (TPR, number of lesions correctly identified as angioectasia in each VCE), viewing time, and speed.
At last, an analysis was also made to assess the performance of each physician together with the results of the algorithm. The purpose was to infer what would be the result of using the algorithm in a clinical practice as a complement to physicians’ readings of VCEs.
The experimental protocol was submitted and approved by the Sub-commission of Life and Health Sciences, University of Minho, and Ethics Committee for Health of Braga Hospital.
Statistical Analysis
Statistical analysis was performed using Statistical Package for the Social Sciences (SPSS Inc®, Chicago, IL, USA version 24.0 for Microsoft® Windows) and MATLAB® software (Mathworks®; version 9.4). For the descriptive data, absolute frequencies (n) and relative frequencies (%) were used for qualitative variables; and medians and interquartile range were used for quantitative variables. Mean and standard deviations were also presented for quantitative variables, when required to facilitate data interpretation. Normal distribution was assessed by the skewness and kurtosis tests. Since this assumption was not observed, non-parametric statistics were used throughout the analysis.
The Mann-Whitney (U), Wilcoxon signed-rank (Z), and Friedman tests were performed to compare the distribution of continuous variables. The χ2, Fisher, and Cochran’s Q tests were assessed to test the association between categorical dichotomic variables. Results were considered statistically significant for a p value below 0.05.
Results
Overall Performance of the CMEMS-Uminho Algorithm
In the evaluation of the full-length 54 VCE recordings, the CMEMS-Uminho algorithm revealed a detection rate of 77.70% (identified 115 from a total of 148 angioectasias) (Fig. 2, 3). The lower performance significantly resulted from the failure of the algorithm in identifying punctiform angioectasias (p < 0.001).
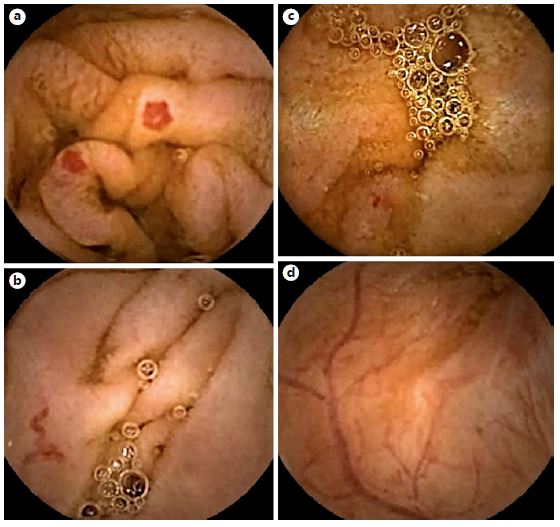
Fig. 2. Examples of frames from PillCam® software selected by the CMEMS-Uminho algorithm: true positive (a,b); false negative (c); false positive (d).
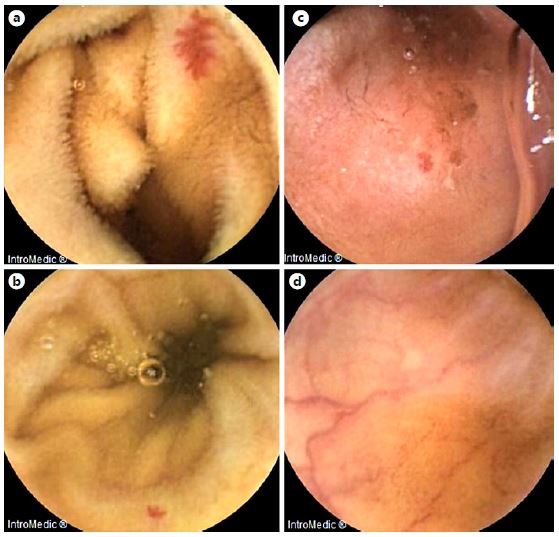
Fig. 3. Examples of frames from MiroCam® software selected by the CMEMS-Uminho algorithm: true positive (a,b); false negative (c); false positive (d).
No significant differences were observed in the algorithm performance between MiroCam® (74.2%) and Pill-Cam® (78.6%) (p = 0.598). Regarding the number of frames (and subsequently the size of the video performed by the software), the algorithm significantly selected more frames in VCEs from MiroCam® (mean = 886.06 vs. 372.45; p = 0.028), suggesting a significant lower specificity. To further explore this data, 100 normal SB images were randomly selected from each software, and histograms with the color intensity of channels a and b were plotted. Both channel a (p < 0.00001) and b (p < 0.00001) of Mirocam® frames significantly showed a higher intensity, with images displaying a more yellowish and reddish coloration, resulting in more false positives (Fig. 4).
Clinical Performance of CMEMS-Uminho and the Impact on Physicians
Regarding the 41 videos collected, 2 VCE examinations were excluded due to the presence of blood and 1 due to poor preparation. Thus, 38 VCE recordings were considered for the analysis, and the CMEMS-Uminho algorithm revealed a diagnostic yield of 94.70% (n = 36). The algorithm failed in 2 VCE examinations that displayed an isolated punctiform angioectasia. Nevertheless, it identified 3 angioectasias that were not identified by any of the physicians.
Concerning the global detection rate (Table 1), no significant differences were observed between physician 1 and 2 (p = 0.728), but both were significantly superior to physician 3 (p < 0.001). After addition of the algorithm analysis, each physician significantly improved the total number of angioectasias detected (p < 0.001) (Table 2), and differences between physicians’ performance were no longer noted (p = 0.108). Similar results were obtained in Detection Rate per capsule. Physician 1 and 2 did not significantly differ (p = 0.064), though both revealed greater detection rate than physician 3 (p < 0.001 and p = 0.029, respectively) (Table 1). All physicians revealed significantly better results following algorithm application (Table 2), and differences between them were diluted (p = 0.108).
With regard to diagnostic yield, only physician 1 detained significant superiority against physician 3 (p = 0.008) (Table 1). Physician 2 and 3 could improve their diagnostic yield, though without significance (p = 0.125 and p = 0.063, respectively) (Table 2). Nevertheless, differences between readers’ performance were no longer detected following algorithm inclusion (p = 0.097).
At last, physician 1 revealed significantly higher TPR than physicians 2 (p < 0.001) and 3 (p < 0.001). Physician 2 also displayed a better result when compared to physician 3 (p = 0.003) (Table 2). Similar results were observed regarding VCE viewing speed and time. Reader 1 was faster (in speed and time) than the other physicians (p < 0.001). Physician 3 was also significantly slower than physician 2 (p < 0.001), but no significant differences were revealed between the time spent in VCE viewing.
Discussion
SB capsule endoscopy is currently the first line of investigation of OGIB and an important diagnostic tool in several other diseases [2-4, 30]. Despite its high diagnostic yield, the true sensitivity of VCE is difficult to determine due to the lack of an adequate gold standard [6]. Furthermore, the reading time and interpretation of video capsule data is very time consuming, given that, in total more than 50,000 images have to be reviewed, which contributes to the high cost of a VCE examination [24]. Thus, a computer aided diagnosis (CAD) tool to help the physicians to evaluate VCE exams faster and more accurately is an important technical challenge and an excellent economic opportunity.
The algorithm developed at the CMEMS-Uminho showed greater performance to detect angioectasias than other methods previously published [26]. To overcome the limitation inherent to most studies that examine CAD tools, we evaluated CMEMS-Uminho algorithm performance in full-length VCE recordings. As expected, angioectasias detection rate diminished, but a satisfactory performance was maintained (77.70%). This result was still superior to suspected blood indicator, which detains a sensitivity of 55% to detect potentially bleeding lesions [31].
Interestingly, our software mostly failed to identify punctiform angioectasias (reduced area/volume). Considering the positive correlation between severity and lesion extension [25, 29], the algorithm sustained a great detection rate of lesions with higher probability of bleeding. Even so, in the future we can “teach” the algorithm in the training stage to enhance its ability to recognize punctiform angioectasias.
This is the first study to assess different VCE systems. Most available tools to enhance VCE diagnostic yield were developed using Given Imaging®, though other systems have been widely used in the clinical practice. CMEMS-Uminho algorithm performance did not differ between both endoscopic capsules evaluated. Even though specificity was not possible to calculate, data suggests that our algorithm displays a lower specificity in VCE recordings from Mirocam®, since the number of frames selected by the algorithm was significantly higher (at the expense of a heavier video). This occurs as a consequence of Mirocam® frames displaying a more yellowish and reddish coloration, resulting in more false positives. Since the algorithm was trained with PillCam® images [26], it possibly makes sense to change the segmentation method so that the CMEMS-Uminho algorithm can better separate the tissue from the angioectasias amongst the normal tissue. This can be done using a segmentation method that uses both channel a and channel b; or the inclusion of a normalization factor that takes into account the overall coloration of each image. Despite this, the algorithm proved to be able to detect angioectasias in both systems, which allows to infer that following a training with images of both capsules systems, the algorithm can increase its diagnostic performance.
The CMEMS-Uminho algorithm revealed an excellent diagnostic yield (94.70%), though interpretation should be carefully done due to our small sample. Notwithstanding, our data may be comparable or even better than previously discussed tools [14-18]. Arieira et al. [19] revealed an overall diagnostic yield of 42% for Top100, though angioectasia was diagnosed in 86.7%. Boal Carvalho et al. [32] also showed that FICE 1 displayed a significantly higher diagnostic yield than white light (55 vs. 42%), particularly to identify angioectasias. Moreover, the 2 VCE examinations that the algorithm failed to identify any lesion displayed an isolated punctiform angioectasia. Hence, training the algorithm not only can possibly improve algorithm overall performance, but also its diagnostic yield.
The main purpose of these computerized tools is to enhance physicians’ performance in the clinical practice. Zheng et al. [6]. revealed that the detection rate of angioectasias by physicians is disappointing, since only 69% of these lesions were detected. In our study, the most experienced physician was faster (in speed and time) to review CE recordings, while revealing greater diagnostic yield and TPR than both trainees, but his global detection rate and detection rate per capsule were not superior to the most experienced trainee. Even though our results should not be inferred to the population due to our sample, our data is comparable to the literature. Experienced capsule endoscopists are not necessarily better interpreters than their less-experienced counterparts [6, 33]. Therefore, experience should not serve as a pass to review studies more efficiently.
Remarkably, the CMEMS-Uminho algorithm might enhance physicians’ performance in the clinical practice. With the addition of the algorithm analysis, it was possible to infer what would be the result of using the algorithm in a clinical practice as a complement to physicians’ readings of VCEs. All readers significantly improved their global detection rate and detection rate per capsule. Moreover, significant differences between them were no longer observed. Consequently, physicians with different experiences in VCE examinations may achieve similar clinical performance, which may also suggest a role of the CMEMS-Uminho algorithm in the learning process of the Gastroenterology trainee.
The main limitation of this study was sample size and the number of physicians enrolled in the study. Also, more than one study-independent capsule endoscopist should have been used to achieve a more accurate algorithm and readers’ performance data. In the future, we intend to improve this tool in order to overcome the exposed failures and to develop an improved version of the software, where other lesions can be simultaneously detected.
In conclusion, this new computerized tool displayed an excellent diagnostic yield and improved physicians’ performance in clinical practice. Multicenter and randomized controlled trials are important to validate our findings.