Introduction
Capsule endoscopy (CE) has revolutionized the approach to patients with suspected small bowel disease, providing noninvasive inspection of the entire small bowel, thus overcoming shortcomings of conventional endoscopy and the invasiveness of deep enteroscopy techniques. The clinical value of CE has been demonstrated for a wide array of diseases, including the evaluation of patients with suspected small bowel hemorrhage, diagnosis and evaluation of Crohn’s disease activity, and detection of protruding small intestine lesions [1-4].
Obscure gastrointestinal bleeding (OGIB), either overt or occult, accounts for 5% of all cases of gastrointestinal bleeding. The source of bleeding is located in the small intestine in most cases, and OGIB is currently the most frequent indication for CE [5]. Nevertheless, the diagnostic yield of CE for detection of the bleeding source remains suboptimal [2].
Evaluation of CE exams can be a burdensome task. Each CE video comprises a mean number of 20,000 still frames, requiring an average of 30-120 min [6]. Moreover, significant lesions may be restricted to a very small number of frames, which increases the risk of overlooking clinically important lesions.
The large pool of images produced by medical imaging exams and the continuous growth of computational power have boosted the development and application of artificial intelligence (AI) tools for automatic image analysis. Convolutional neural networks (CNNs) are a subtype of AI based on deep learning algorithms. This neural architecture is inspired by the human visual cortex, and it is designed for enhanced image analysis and pattern detection [7]. This type of deep learning algorithm has delivered promising results in diverse fields of medicine [8-10]. Endoscopic imaging, and particularly CE, is one of the branches where the development of CNN-based tools for automatic detection of lesions is expected to have a significant impact [11]. Future clinical application of these technological advances may translate into an increase in diagnostic accuracy of CE for the detection of several lesions. Moreover, these tools may contribute to optimizing the reading process, including its time cost, thus lessening the burden on gastroenterologists and overcoming one of its main drawbacks.
With this project, we aimed to create a CNN-based system for automatic detection of blood or hematic traces in the small bowel lumen. This project was divided into 3 distinct phases: first, acquisition of CE frames containing normal mucosa or other findings and others with luminal blood or hematic traces; second, development of a CNN and optimization of its neural architecture; third, validation of the model for automatic identification of blood or hematic traces in CE images.
Materials and Methods
Patients and Preparation of Image Sets
All subjects submitted to CE between 2015 and 2020 at a single tertiary center (São João University Hospital, Porto, Portugal) were approached for enrolment in this retrospective study (n = 1,229). A total of 1,483 CE exams were performed. Data retrieved from these examinations was used for development, training, and validation of a CNN-based model for automated detection of blood or hematic residues within the small bowel in CE images. The full-length CE video of all participants was reviewed (total number of frames: 23,317,209). Subsequently, 22,095 frames were ultimately extracted. These frames were labeled by 2 gastroenterologists (M.M.S. and H.C.) with experience in CE (>1,000 CE exams). The inclusion and final labelling of the frames was dependent on a double-validation method, requiring consensus between both researchers for the final decision.
Capsule Endoscopy Protocol
All procedures were conducted using the PillCamTM SB3 system (Medtronic, Minneapolis, MN, USA). The images were reviewed using PillCamTM Software version 9 (Medtronic). Image processing was performed in order to remove possible patient-identifying information (name, operating number, date of procedure). Each extracted frame was stored and assigned a consecutive number.
Each patient received bowel preparation following previously published guidelines by the European Society of Gastrointestinal Endoscopy [12]. In summary, patients were recommended to have a clear liquid diet on the day preceding capsule ingestion, with fasting in the night before examination. A bowel preparation consisting of 2 L of polyethylene glycol solution was used prior to the capsule ingestion. Simethicone was used as an anti-foaming agent. Prokinetic therapy (10 mg domperidone) was used if the capsule remained in the stomach 1 h after ingestion, upon image review on the data recorder worn by the patient. No eating was allowed for 4 h after the ingestion of the capsule.
Convolutional Neural Network Development
From the collected pool of images (n = 22,095), 13,510 images contained hematic content (active bleeding or free clots) and 8,585 had normal mucosa or nonhemorrhagic findings (protruding lesions, vascular lesions, lymphangiectasia, and ulcers and erosions). A training dataset was constructed by selecting 80% of all extracted frames (n = 17,676). The remaining 20% were used for the construction of a validation dataset (n = 4,419). The validation dataset was used for assessing the performance of the CNN. A flowchart summarizing the study design is presented in Figure 1.
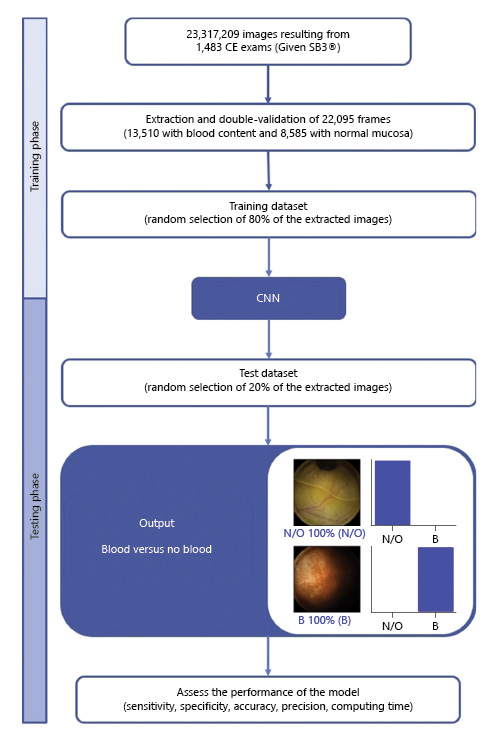
Fig. 1 Study flow chart for the training and validation phases. N/O, normal/other findings; B, blood or hematic residues.
To create the CNN, we used the Xception model with its weights trained on ImageNet. To transfer this learning to our data, we kept the convolutional layers of the model. We removed the last fully connected layers and attached fully connected layers based on the number of classes we used to classify our endoscopic images. We used 2 blocks, each having a fully connected layer followed by a Dropout layer of 0.3 drop rate. Following these 2 blocks, we added a Dense layer with a size defined as the number of categories to classify. The learning rate of 0.0001, batch size of 32, and the number of epochs of 100 were set by trial and error. We used Tensorflow 2.3 and Keras libraries to prepare the data and run the model. The analyses were performed with a computer equipped with an Intel® Xeon® Gold 6130 processor (Intel, Santa Clara, CA, USA) and a NVIDIA Quadro® RTXTM 4000 graphic processing unit (NVIDIA Corporate, Santa Clara, CA, USA).
Performance Measures and Statistical Analysis
The primary performance measures included sensitivity, specificity, precision, and accuracy in differentiating between images containing blood and those with normal findings or other pathologic findings. Moreover, we used receiver operating characteristic (ROC) curves analysis and area under the ROC curves to measure the performance of our model. The network’s classification was compared to the diagnosis provided by specialists’ analysis, the latter being considered the gold standard. In addition to the evaluation of diagnostic performance, the computational speed of the network was determined by calculating the time required by the CNN to provide output for all images.
For each image, the model calculated the probability of hematic content within the enteric lumen. A higher probability score translated into a greater confidence in the CNN prediction. The category with the highest probability score was outputted as the CNN’s predicted classification (Fig. 2). Sensitivities, specificities, and precisions are presented as means ± SD. ROC curves were graphically represented and AUROC calculated as means and 95% CIs, assuming normal distribution of these variables. Statistical analysis was performed using Sci-Kit learn version 0.22.2 [13].
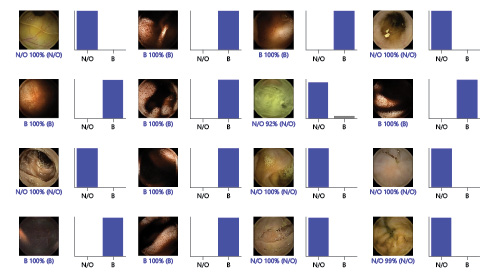
Fig. 2 Output obtained from the application of the convolutional neural network. The bars represent the probability estimated by the network. The finding with the highest probability was outputted as the predicted classification. A blue bar represents a correct prediction. Red bars represent an incorrect prediction. N/O, normal/other findings; B, blood or hematic residues.
Results
Construction of the Network
The performance of the CNN was evaluated using a validation dataset containing a total of 4,419 frames (20% of the total of extracted images), 2,702 labeled as containing blood in the enteric lumen, and 1,717 with normal mucosa or other distinct pathological findings. The CNN evaluated each image and predicted a classification (normal mucosa/other pathologic findings or luminal blood/hematic residues) which was compared with the labelling provided by the pair of specialists. The network demonstrated its learning ability, with increasing accuracy as data was being repeatedly inputted into the multi-layer CNN (Fig. 3).
Global Performance of the Network
The distribution of results is displayed in Table 1. The calculated sensitivity, specificity, precision, and accuracy were 98.6% (95% CI 97.6-99.7), 98.9% (95% CI 97.6-99.7), 98.7% (95% CI 97.7-99.6), and 98.5% (95% CI 98.6-98.6). The AUROC for detection of hematic content in the small bowel was 1.0 (Fig. 4).
Discussion/Conclusion
CE is a minimally invasive diagnostic method for the study of suspected small bowel disease. It assumes a pivotal role in the investigation of patients presenting with OGIB, either overt or occult [2]. In this setting, CE has shown to have a comparable diagnostic yield to the more invasive double-balloon enteroscopy, and it has demonstrated a favorable cost-effectiveness profile for the initial management of patients with OGIB [2, 14, 15]. However, reading CE exams is a monotonous and time-consuming task for gastroenterologists, which increases the risk of failing to diagnose significant lesions. The exponential growth of AI technologies allows to aim at the development of efficient tools that can assist the gastroenterologist in visualizing these exams, thus overcoming these drawbacks.
In this study, we have developed a deep learning tool capable of automatically detecting luminal blood or hematic residues in the small bowel using a large pool of CE images. Our CNN demonstrated high sensitivity, specificity, and accuracy for the detection of these findings. Also, our algorithm demonstrated a high image processing performance, with approximate image reading rates of 184 images per second. This performance is superior to most studies exploring the application of CNN algorithms for automatic detection of lesions in CE images [6, 16-19]. Although this is a proof-of-concept study, we believe that these results are promising and lay foundations for the development and introduction of these tools for AI-assisted reading of CE exams. Subsequent studies in real-life clinical settings, preferably of prospective and multicentric design, are required to assess if AI-assisted CE image reading translates into enhanced time and diagnostic efficiency compared to conventional reading.
OGIB is the most common indication for CE [20]. Several studies have assessed the performance of different AI architectures for the automatic detection of blood in CE images. Pan et al. [21] developed the first CNN for detection of GI bleeding, based on a large number of images (3,172 hemorrhage images and 11,458 images of normal mucosa). Their model had a sensitivity and specificity of 93 and 96%, respectively. Moreover, deep learning CNN models have shown to be able to identify lesions with substantial hemorrhagic risk, such as angiectasia, with high sensitivity and specificity [6, 18].
Recently, Aoki et al. [16] shared their experience with a model designed for the identification of enteric blood content. Our network presented performance metrics comparable to those reported in their work. Nevertheless, our system had a higher sensitivity (98.6 vs. 96.6%). Further studies replicating our results are needed to assess if this gain in sensitivity translates into fewer missed bleeding lesions. Moreover, our algorithm demonstrated a higher image processing performance (184 vs. 41 images per second). The clinical relevance of this increased image processing capacity is yet to be determined. Subsequent cost-effectiveness studies should address this question.
We developed a highly sensitive, specific, and accurate model for the detection of luminal blood in CE images. Our results are superior to most other studies regarding the application of AI techniques in the detection of GI hemorrhage (Table 2). Although promising, the interpretation of these results must acknowledge the proof-of-concept design of this study and that the application of this tool to a real clinical scenario may lead to different results.
There are several limitations to our study. First, our study focused on patients evaluated at a single center and was conducted in a retrospective manner. Thus, these promising results must be confirmed by robust prospective multicenter studies before application to clinical practice. Second, analyses in this proof-of-concept study were image-based instead of patient-based. Second, we applied this model to selected still frames and, therefore, these results may not be valid for full-length CE videos. Third, all CE exams during this period were performed using the Pillcam SB3® system. Therefore, results may not be completely generalizable for other CE systems. Fourth, although our network demonstrated high image processing speed, we did not assess if CNN-assisted image review reduces the reading time compared to conventional reading.
In summary, it is expected that the implementation of AI algorithms in daily clinical practice will introduce a landmark in the evolution of modern medicine. The development of these tools has the potential to revolutionize medical imaging. Endoscopy, and particularly CE, is a fertile ground for such innovations. The development and validation of highly accurate AI tools for CE, such as that described in this study, constitute the basic pillar for the creation of software capable of assisting the clinician in reading these exams.