BACKGROUND
Kidney transplant success as kidney replacement treatment for end-stage renal disease patients has evolved over several decades, with both scientific advances and some incidents, and it should be viewed as a work-in-progress.1 Renal transplantation, when possible, is the preferential treatment for patients with end-stage kidney disease. It is associated with a long-term reduction in risk of death when compared to patients on dialysis waiting for a transplant.2 As donorrecipient incompatibilities are a major cause for rejection, improving the match between donors and recipients could minimize the rejection rates and optimize the utilization of such valuable resource.3
In 2018, with 256 cases per million population (p.m.p.), Portugal was the second European country with the highest incidence of patients starting renal replacement therapy, barely surpassed by Greece.4 In Portugal, the prevalence of patients in renal replacement therapy was 2011 p.m.p., the highest number in all of Europe. A much higher value than those from countries like Spain, Belgium, or Greece with less than 1500 patients p.m.p.4
The implementation of new measures to improve activities related to renal transplantation in Portugal requires the definition of systematic metrics for these activities. We not only need to know how kidney transplantation has evolved in our country over the years, but we also need tools that allow us to assess established practices. Thus, we seek to make available several open access applications that can be an adjuvant to evaluate transplantation activities as well as some implemented policies, in Portugal. Some of these applications include: predicting transplant’s outcomes; count the percentage of potential histocompatible donors that may be available for a given patient; simulating kidney allocation rules; or simple visualizations of the evolution of kidney transplantation compared to other European Union countries.
Health data science is a multidisciplinary concept rendered into the extraction of knowledge from data using theories and techniques from fields as diverse as computation, statistics, epidemiology, and knowledge domains such as medical specialties.5 Although there is evidence that these computational and statistical techniques could be an asset to the field of transplantation, the best way to implement them in the clinical management of renal patients is still questionable.6
Data science solutions applied to patients’ managing and monitoring are increasingly frequent, either as an aid in determining diagnoses or for prognosis and prediction of disease evolution. Machine learning can be used to develop models that predict transplant outcomes, allowing efficiency gains in organ allocation by determining the ideal candidate to receive a specific organ, maximizing the survival of both patient and graft.6 Likewise, these models can be a clinical prognostic tool that maximizes the best matching between donor and recipient while ensuring equity in kidney allocation systems.
This article presents the Transplant Open Registry (TxOR) initiative.7 TxOR (Fig. 1) compiles health data science applications on kidney transplantation, available as free open source.
TXOR
TxOR is a private initiative based on open-source means to provide high-quality information on kidney transplantation in Portugal. The fame-motivated open-source community grants a savory opportunity to make TxOR evolve at a higher pace than it would as proprietary software.8
Health data science has the potential to generate knowledge by extracting information from data, and getting answers to clinical research questions otherwise unsolvable through more traditional research approaches. With this in mind, the TxOR initiative’s mission is to:
• Assemble open data from both kidney transplant donors and recipients and make it available, in a transparent and accessibl format, for all interested parties;
• Provide clear and transparent information on kidney transplantation activities in Portugal allowing informed data-driven decision-making;
• Perform data and epidemiological analysis with open data on transplantation;
• Look for the best kidney allocation algorithms for kidney transplantation, using simulations to find an equitable way to distribute this valuable and scarce resource.
We lived in a so-called big data era and collections of data are generated constantly. Big data is usually characterized by 3 dimensions: Volume, with more data-collecting devices, used every day, the volume of data increases dramatically; Variety, data can have different formats and different levels of completeness; and Velocity, as the volume of data increases, the rate of data generation (from the internet of things, social media, etc.) is increasing as well. Big data use has the potential to revolute medical practice as we know it.
In Portugal, the Portuguese Institute of Blood and Transplantation (IPST) is responsible for maintaining and running an information system to manage the candidates’ kidney transplant waiting list and the selection of donor-recipient pairs in renal transplantation. Moreover, according to the Council of Europe, member states’ entities responsible for transplant’s waiting list management must provide information, to the general public, at least on an annual basis. Comparing the information provided by Portugal with the information from other European Union countries, it can be quickly concluded that IPST has very limited performance in this concern. Unfortunately, neither a characterization of the transplant candidates’ waiting list over the years nor a clear description of transplanted patients could be found.9
APPLICATIONS
At TxOR we found two applications: Renal Transplant activity in Portugal and Portugal within the EU (Fig. 2); that use data from the Global Observatory on Donation and Transplantation.10 These applications analyze the evolution of renal transplantation activity in Portugal11 and compare some of the information with that from other European Union countries.12 Annual rates of kidney transplants by type of donor and the number of deceased donors for organ transplantation are presented since 2003.
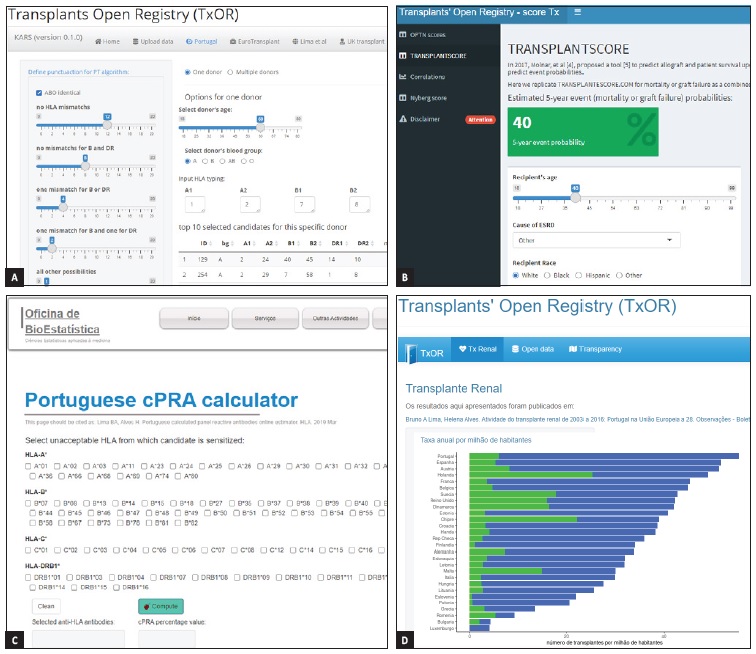
From left to right, top to bottom: A -The Kidney Allocation Rules Simulator (KARS); B -the Score Tx application; C - the cPRA calculator; D - Portugal within the EU.
In 2020, while Portugal was the fourth country with the higher rate p.m.p. of renal transplants performed from deceased donors in the European Union, it only occupies the 14th position for kidney transplants from living donors.10 In 2009, Portugal, reached the higher number of performed transplants from deceased donors’ kidneys, with 50 transplants p.m.p., whilst between 2015 and 2019 barely surpasses 40 transplants p.m.p, even with a similar number of registered deceased donors (between 31 and 34 p.m.p.). The exception was 2020 with a reduced number of deceased donors (24.8 p.m.p.) and also a reduced number of performed transplants both from deceased and living donors (34.6 and 4 p.m.p., respectively), explainable by the effects of the COVID pandemic. Moreover, we also can observe that the number of patients actively listed for transplantation has been constantly around two thousand, for the last decade. For both applications, we have a left panel from which the user can select the indicators and a specific year to be displayed, while on the right we can visualize the data. These applications versions are intended to be used in a personal computer rather than a smartphone.
Human leukocyte antigens (HLA) are very polymorphic proteins that play a pivotal role in transplantation. HLA-A, -B, and -C (class I) are present on virtually all our cells (except red blood cells) while HLA-DR,-DQ, and HLA-DP (class II) are mainly on immune cells. While HLA polymorphism may help diversify humanity, it is a huge barrier to successful transplantation, which requires matching, as closely as possible, the HLA types of the recipient and donor.13
Sensitized patients have alloantibodies reactive to HLA alleles. Renal transplant candidates with HLA sensitization tend to be disregarded in kidney transplant allocation and even when transplanted they have a higher risk of graft rejection and long-term complications.14
For each transplant candidate, the calculated Panel Reactive Antibody (cPRA) value estimates the percentage of potential deceased donors having HLA alleles for which the candidate is reactive and consequently not an option for transplantation. Patients on the waiting list for renal transplantation from a deceased donor with higher cPRA values are less likely to found a suitable donor and must be informed about other alternatives (as could be transplantation with a living donor). The reliability of cPRA values depends on the pool of potential donors from which it is estimated and as such those donors’ HLA allelic and haplotypic frequencies must be known and scrutinized. The application Portuguese cPRA calculator15 available from TxOR, computes cPRA values from identified HLA antibodies and is based on HLA frequencies from Portuguese voluntary bone marrow donors16 and therefore comparable to potential deceased kidney donors in Portugal. With transplant candidates’ HLA alloantibodies as input, the cPRA calculator returns a percentage estimate of potential donors not available for transplantation.
When allocating a scarce commodity such as deceased donor kidneys for transplantation, it is crucial maximizing transplant outcome in order to guarantee efficient use of that organ. The application ScoreTx17 compiles some risk scores to predict kidney transplants outcome using only data known at the time of transplantation for the donor-recipient pair. The Organ Procurement and Transplantation Network (OPTN) uses two different scores: an Estimated Post Transplant Survival (EPTS) score (based on patients characteristics) to summarize the fitness of the patient; and a Kidney Donor Percentual Index (KDPI), that combines factors from donors and from the transplant, to summarize the risk of graft failure.
Also, we make available the TRANSPLANTSCORE proposed by Molnar et al18 to predict posttransplant outcomes (for mortality or graft failure as a combined outcome) using pretransplant
information, computing the estimated 5-year event probability. Moreover, the Nyberg score19 can be computed from ScoreTx17 using only variables from the donor and the number of HLA mismatches with the recipient. In particular, this score is a quantitative approach to assessing kidneys from expanded criteria donors. The development of risk scores that can predict the outcome inherent to the data-recipient pair at transplantation has allowed for better decision-making either in allocating organs and patients’ clinical management.
According to the Council of Europe, organ transplantation allocation rules must be changed whenever necessary to ensure that similar groups of patients have similar waiting times. The evaluation of new kidney allocation rules should depend on simulations that will allow, as far as possible, to predict future results and outcomes.
The Kidney Allocation Rules Simulator (KARS) application20 allows simulating different sets of rules changing the respective parameters and using different data.21 Having as inputs files with transplant candidates’ demographic and immunological data, with KARS we can run simulations on kidney allocation systems and compare final results. In this application, it is possible to simulate allocation rules implemented in Portugal, in countries within Eurotransplant, in the United Kingdom, and an allocation system previously suggested by Lima et al.22
Portuguese kidney allocation system on transplantation (implemented in 2007) is based on a points system that takes in consideration: HLA mismatches between donor and transplant candidate, level of immunization of the candidate, time on dialysis, and age difference between donor and transplant candidate. Additionally, hypersensitized candidates (cPRA >85%) are prioritized and after that all candidates are ordered by their corresponding punctuation.
Also, the KARS app allows changing the original punctuation used by the aforementioned allocation systems, to simulate how these changes are translated into the type of candidates selected for transplantation. For each one of the models, transplant candidates are excluded considering ABO compatibility and virtual crossmatch, and the remaining are ordered for each donor according to the model’s rules. For the option ‘One donor’, the top 10 candidates are displayed regarding the donor characteristics; for the option ‘Multiple donors’ each donor is attributed to de 2 best classified candidates and at the end, we will have a dataset with the number of candidates equal to the double of the number of donors. Finally, a resume of the characteristics of the selected candidates is displayed allowing for the comparison between models. Currently, fifteen years after its enforcement, there are a scientific consensus on the need to revise and upgrade the Portuguese kidney allocation system on transplantation.23 Our KARS app is a tool available to researchers interested on simulating different scoring systems and comparing it with allocation systems implemented in other countries.
We also developed the R package24 that allows generating synthetic data on donors and transplant candidates particularly useful on the KARS app.20 The availability of synthetic data plays an increasingly important role in the development cycle of any data science project, allowing the development and preparation of the project before the use of real data without having to depend on it.25
DISCUSSION
Data science technologies apply to several fields and have the ability to transform them. Health care is no exception and, here too, data science plays an increasingly relevant role.
The growing availability of health data in high volume, variety, and velocity, when reachable for data science, presents an opportunity to respond to those problems postured by precision medicine.5 Also, the concept of Real World Evidence applies to evidence in healthcare obtained through so-called Real World Data and can be used to achieve better data-driven decision-making.26
In order to implement algorithms that can transform data into information or build predictive models that can be used in production, interventions are often necessary both in collecting patients’ data and analyzing institutions’ needs. In kidney transplantation, machinelearning prediction models based on information from donor-recipient pairs can autonomously learn those donor-recipient relationships to predict transplant outcomes.3 However, upstream changes are still needed to implement these new approaches. The main objective of health data science is to allow both clinicians and researchers to make better decisions5 and cannot be seen as something esoteric or distant.
Defining key performance indicators, which permit systematically and objectively measuring access to kidney transplantation and its outcomes, is essential for making informed decisions in health policies’ assessment. Only with a detailed description of candidates on the transplant waiting list, both at a socio-demographic level and their immunological characteristics, it is possible to know what type of interventions can be taken to guarantee equitable access to kidney transplants.27 Unfortunately, the IPST has not been able to provide this kind of data, much less the processed information that could help make better decisions in the field. Furthermore, survival rates of kidney transplantation are not known for the current Portuguese deceased donor allocation model, not allow comparing this model with the ones implemented in other countries.27
A kidney transplanted patient undergoes several stages of clinical follow-ups, such as diagnosis of chronic kidney disease, the assessment of end-stage renal disease, pre-transplantation follow-up, and post-transplantation follow-up. Providing these patients with quality health care is ensured when clinicians have access to reliable patient health data that allows critical decisions to be taken5 and equally with posterior processing of patients’ compiled data. This secondary use of health data is an enhancement to improve personal care planning, research, or policymaking and must be encouraged and stimulated.28
There is a growing movement calling for the availability of open health data allowing its analysis and processing with data science technologies, facilitating access to information and knowledge. The current COVID-19 pandemic also served to enhance and demonstrate the merits of this movement. Similarly, within the scope of kidney transplantation in Portugal, we appeal to the responsibility of public entities to make this kind of data available as a way of stimulating the transparency required to public administrations; even more when these entities have shown themselves incapable of using it.29
Principal applications available at TxOR (scoreTx, cPRA, KARS) can be seen as calculators and one of their main advantages rely on the fact that at no time individual data are stored or processed neither can be accessed by anyone other than the user itself. As we do not manage users’ data neither meta-data, we do not jeopardize any rule imposed by the European General Data Protection Regulation.
Although we need data to develop new policies or to evaluate existing ones, we cannot disregard concerns about individual privacy issues. With this in mind, we also made available an R package to generate synthetic data, this kind of data simulates real data and was used to develop KARS app. We do not ask for individual data to be made public, but we need to have access to a clear and good characterization of patients and donors (as legally required) to generate more accurate synthetic data. On Renal Transplant activity in Portugal and Portugal within the EU applications, we used public available aggregated data that, by its nature, it is not possible to identify any individual person from.
LIMITATIONS
Applications presented at TxOR are not medical devices and do not have a commercial purpose. Likewise, each of them must be seen as a proof of concept, i.e., as realizations of its feasibility.
Moreover, we expect to still build on them, making them more user-friendly and with more functionalities. Also, as open source software, TxOR’s applications are intentionally generic and not intended to be specific. Hence they can be used and adapted by anyone without any cost. We also must emphasize that algorithms on scoreTX were developed by others and do not used data from Portuguese patients. In future versions of these applications, we expect to make them more user-friendly with a better interface if accessed through smartphones.
CONCLUSION
Machine learning techniques alone are not a panacea that will solve everything, and they are also subject to biases. Nevertheless, the potential of Real World Data to generate quality scientific evidence cannot be squandered by its custodians’ failure to make data available.26
Neither data alone nor only complex algorithms can answer complex clinical questions. This is the era of ‘big data’ but there is still a large gap between the amount of accumulated health data and medical domain knowledge. The gap can only be bridged by multidisciplinary teams that should include clinicians, data scientists, epidemiologists, and informaticians.5
TxOR initiative is built on health data science and tries to answer, some of the enduring questions on kidney transplantation in Portugal, with a new approach. It hopes to contribute to a fruitful discussion that allows clarifications on some hot topics such as the constant need for a better kidney allocation system.